Enantioselectivity prediction of pallada-electrocatalysed C–H activation using transition state knowledge in machine learning
Nature Synthesis(2023)
摘要
Enantioselectivity prediction in asymmetric catalysis has been a long-standing challenge in synthetic chemistry because of the high-dimensional nature of the structure–enantioselectivity relationship. A lack of understanding of the synthetic space results in laborious and time-consuming efforts in the discovery of asymmetric reactions, even if the same transformation has already been optimized on model substrates. Here we present a data-driven workflow to achieve a holistic enantioselectivity prediction of asymmetric pallada-electrocatalysed C–H activation by implementing transition state knowledge in machine learning. The vectorization of transition state knowledge allowed for an excellent description and extrapolation of the machine learning model, and enabled the quantitative evaluation of 846,720 possibilities. Model interpretation revealed the non-intuitive olefin effect on the enantioselectivity determination. Subsequent density functional theory calculations unravelled mechanistic knowledge that the rate-determining step depends on the olefin reactivity in the insertion step. Therefore, the olefin insertion step can be involved in the overall enantioselectivity determination. These results highlight the complementary features of knowledge-based machine learning with an interpretation-driven mechanistic study, which provides the opportunity to harness widely existing catalysis screening data and transition state models in molecular synthesis.
更多查看译文
关键词
Asymmetric catalysis,Catalyst synthesis,Stereochemistry,Chemistry/Food Science,general
AI 理解论文
溯源树
样例
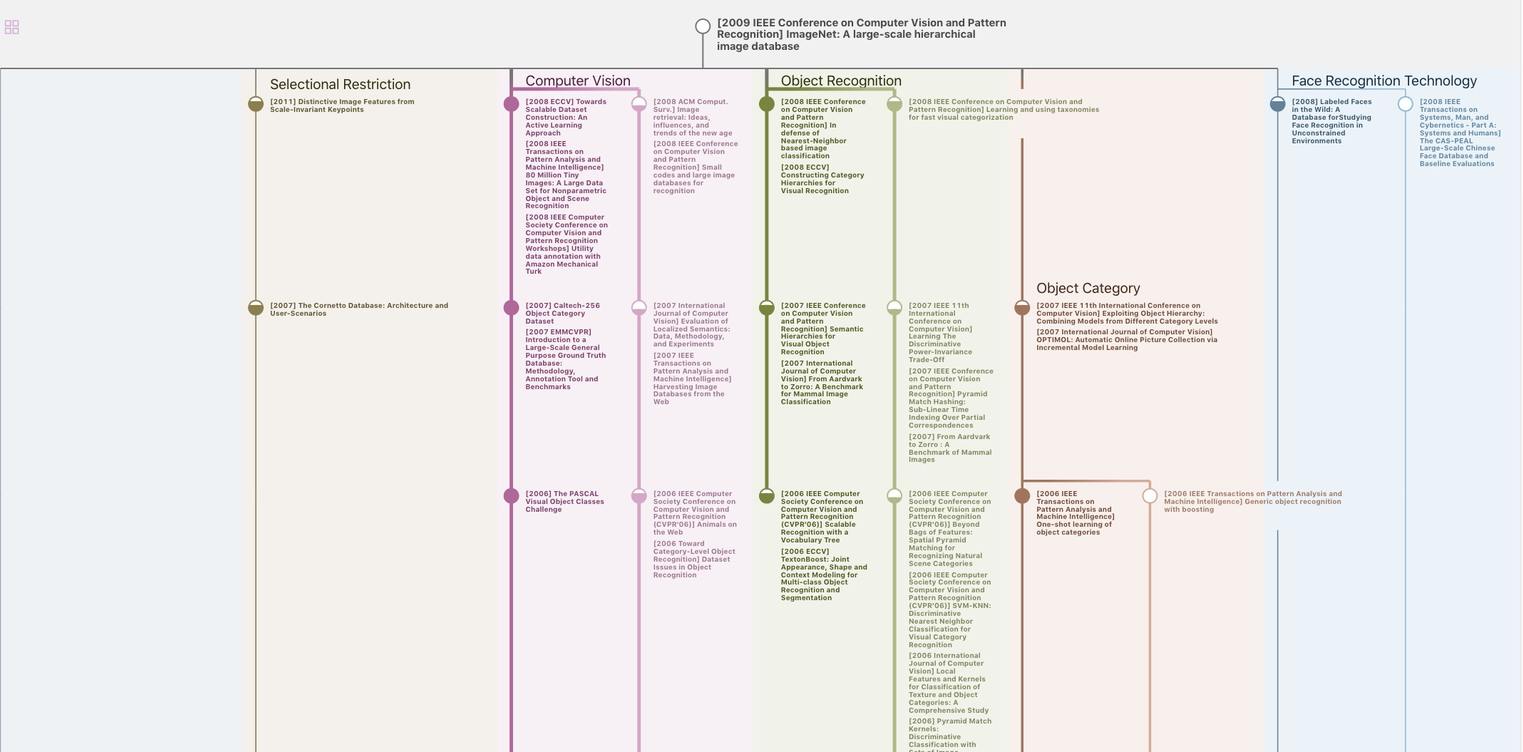
生成溯源树,研究论文发展脉络
Chat Paper
正在生成论文摘要