A Generic Framework for Deploying Video Analytic Services on the Edge
IEEE Transactions on Cloud Computing(2023)
摘要
This article introduces a novel distributed model for handling in real-time, edge-based Artificial Intelligence analytics, such as the ones required for smart video surveillance. The novelty of the model relies on decoupling and distributing the services into several decomposed functions which are linked together, creating virtual function chains (
$VFC$
model). The model considers both computational and communication constraints. Theoretical, simulation and experimental results have shown that the
$VFC$
model can enable the support of heavy-load services to an edge environment while improving the footprint of the service compared to state-of-the art frameworks. In detail, results on the
$VFC$
model have shown that it can reduce the total edge cost, compared with a Monolithic and a Simple Frame Distribution models. For experimenting on a real-case scenario, a testbed edge environment has been developed, where the aforementioned models, as well as a general distribution framework (Spark ©) and an edge-deployement framework (Kubernetes©), have been deployed. A cloud service has also been considered. Experiments have shown that
$VFC$
can outperform all alternative approaches, by reducing operational cost and improving the QoS. Finally, a caching and a QoS monitoring service based on Long-Term-Short-Term models are introduced and evaluated.
更多查看译文
关键词
deploying video analytic services,edge,framework
AI 理解论文
溯源树
样例
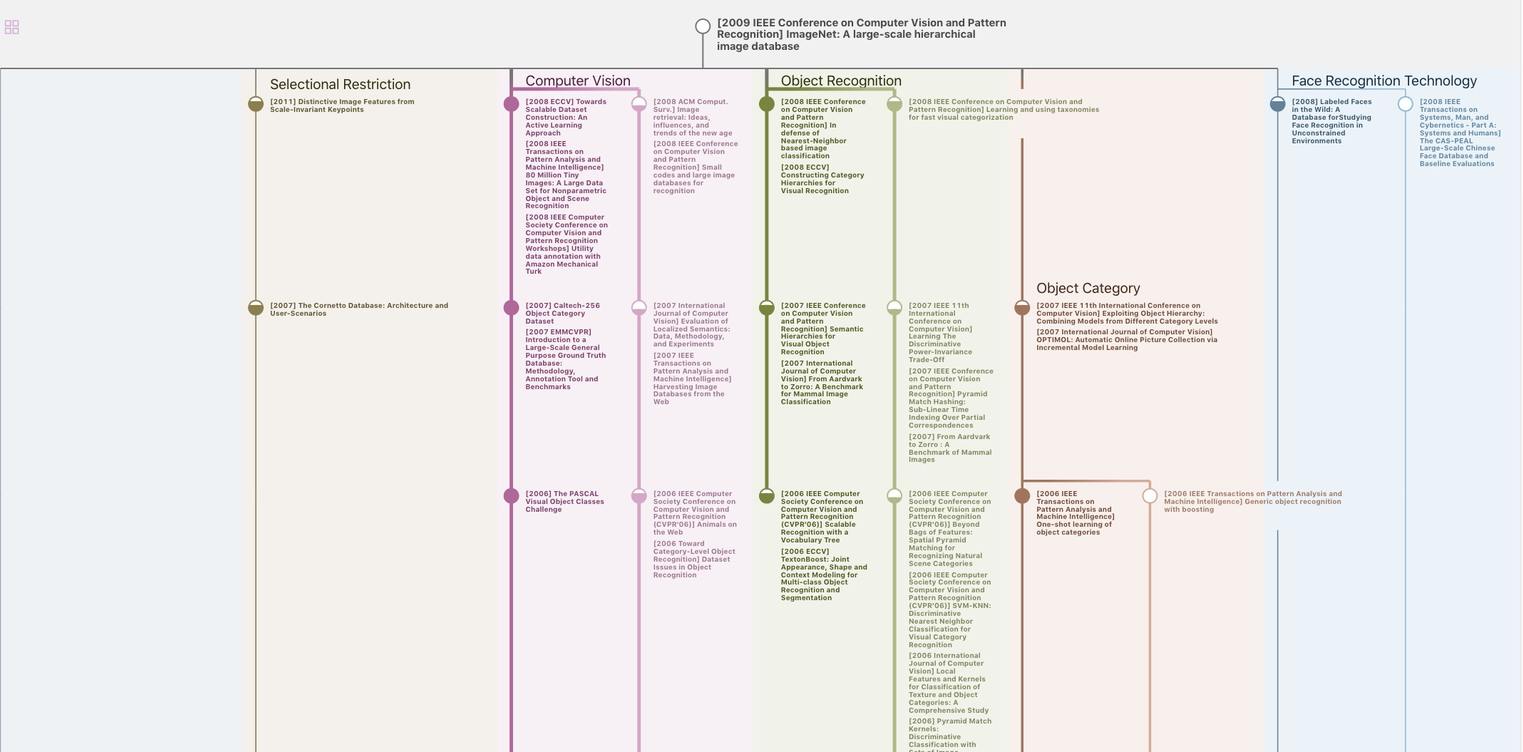
生成溯源树,研究论文发展脉络
Chat Paper
正在生成论文摘要