Multi agent double deep Q-network with multiple reward functions for electric vehicle charge control
2022 IEEE Power & Energy Society General Meeting (PESGM)(2022)
摘要
Even today, electric vehicles (EVs) can endanger grid stability by overloading equipment at the low-voltage (LV) level due to high charging power at private charging points and simultaneity. With a high share ofEVs in the future, it is therefore necessary to control their charging power in such a way that congestion of equipment in the LV grid is avoided. In order to increase the user acceptance of EVs, a fast charging time of the EVs has to be guaranteed despite the control of the charging power. For achieving this, an autonomously acting control algorithm using a multi agent double deep Q-network (MADDQN) has been defined and validated in simulation in the following paper. For this purpose, multiple reward functions have been defined. In the validation on the modified CIGRE LV grid with a share of 100% EVs, it has been shown that the MADDQN can reduce the transformer utilization by up to 50 % relative to the uncontrolled case. At the same time, the charging time can be increased by 51 % relative to the minimum EV charging power of 1.4 kW.
更多查看译文
关键词
multiple reward functions,multi agent,electric vehicle,q-network
AI 理解论文
溯源树
样例
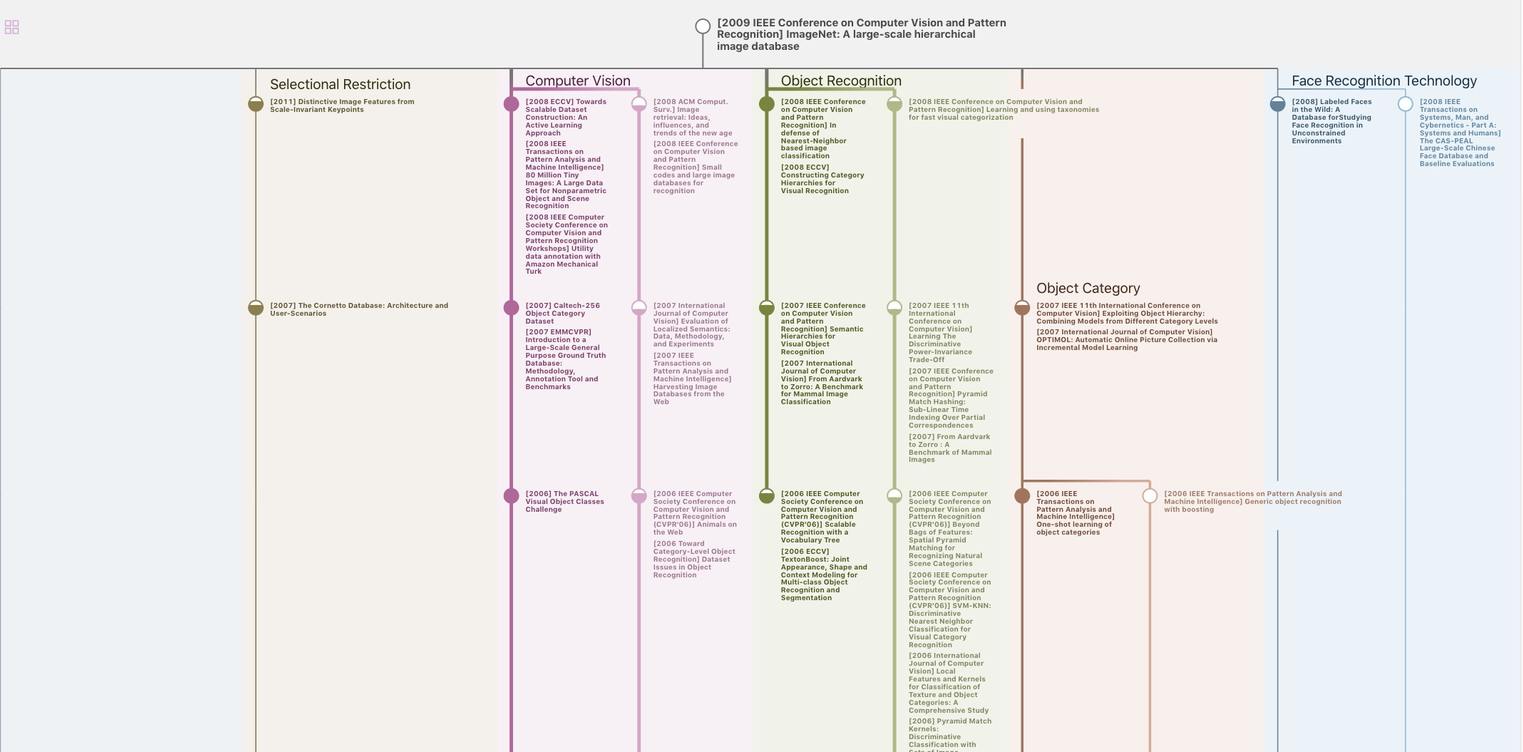
生成溯源树,研究论文发展脉络
Chat Paper
正在生成论文摘要