A Possibilistic Information Fusion-Based Unsupervised Feature Selection Method Using Information Quality Measures
IEEE Transactions on Fuzzy Systems(2023)
摘要
The main goal of most information quality (IQ)-based measures is to combine data provided by multiple information sources to enhance the quality of information essential for decision makers to perform their tasks. However, there is few work to fuse multisource information from the perspective of possibility distribution (PD) and use IQ as the evaluation criteria for feature selection. The PD is one of important concepts in the possibility theory, which is a generally acknowledged method for describing a kind of uncertain knowledge. In this article, we propose a novel representation model of PDs based on FMs, namely, a possibility distribution information system (PDIS). Then, several IQ measures are defined in the PDIS, including Gini entropy, compatibility, conflict, credibility, and separability degrees. In view of this, a minimal-separability-minimal-uncertainty-based unsupervised feature selection algorithm (UmSMU) is designed. The proposed UmSMU can sufficiently fuse multiple possibilistic information. Meanwhile, the selected features maintain as much information as possible while minimizing the uncertainty of information. The experimental results show that the proposed algorithm performs well, especially when it comes to selecting fewer features and improving performance.
更多查看译文
关键词
Feature extraction,Uncertainty,Information systems,Fuses,Entropy,Fuzzy sets,Redundancy,Feature selection,fuzzy multisets (FMs),information quality (IQ) measure,possibilistic information fusion,possibility distribution (PD)
AI 理解论文
溯源树
样例
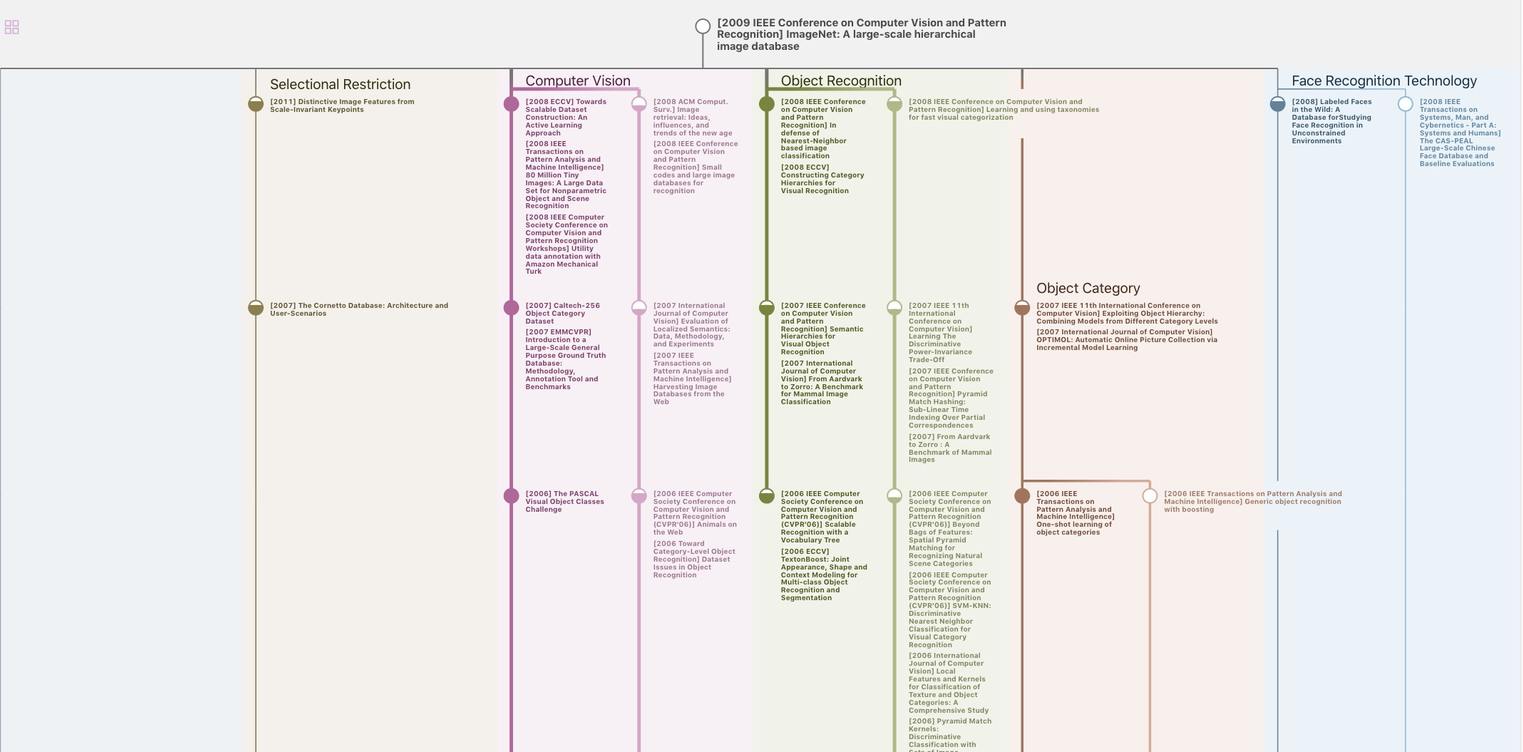
生成溯源树,研究论文发展脉络
Chat Paper
正在生成论文摘要