Data Augmentation and Intelligent Fault Diagnosis of Planetary Gearbox Using ILoFGAN Under Extremely Limited Samples
IEEE Transactions on Reliability(2023)
摘要
Although the existing generative adversarial networks (GAN) have the potential for data augmentation and intelligent fault diagnosis of planetary gearbox, it remains difficult to deal with extremely limited training samples and effectively fuse the representative and diverse information. To tackle the abovementioned challenges, an improved local fusion generative adversarial network is proposed. Time-domain waveforms are first transformed into the time–frequency diagrams to highlight the fault characteristics. Subsequently, a local fusion module is used to fully utilize extremely limited samples and fuse the local features. Finally, a new generator embedded with multi-head attention modules is constructed to effectively improve the accuracy and flexibility of the feature fusion process. The proposed method is applied to the analysis of planetary gearbox vibration signals. The results show that the proposed method can generate a large number of samples with higher similarity and better diversity compared with the existing mainstream GANs using six training samples in each type. The generated samples are used to augment the limited dataset, prominently improving the accuracy of the fault diagnosis task.
更多查看译文
关键词
Extremely few samples, improved local fusion generative adversarial network (ILoFGAN), intelligent fault diagnosis, multihead attention (MHA) mechanism, planetary gearbox
AI 理解论文
溯源树
样例
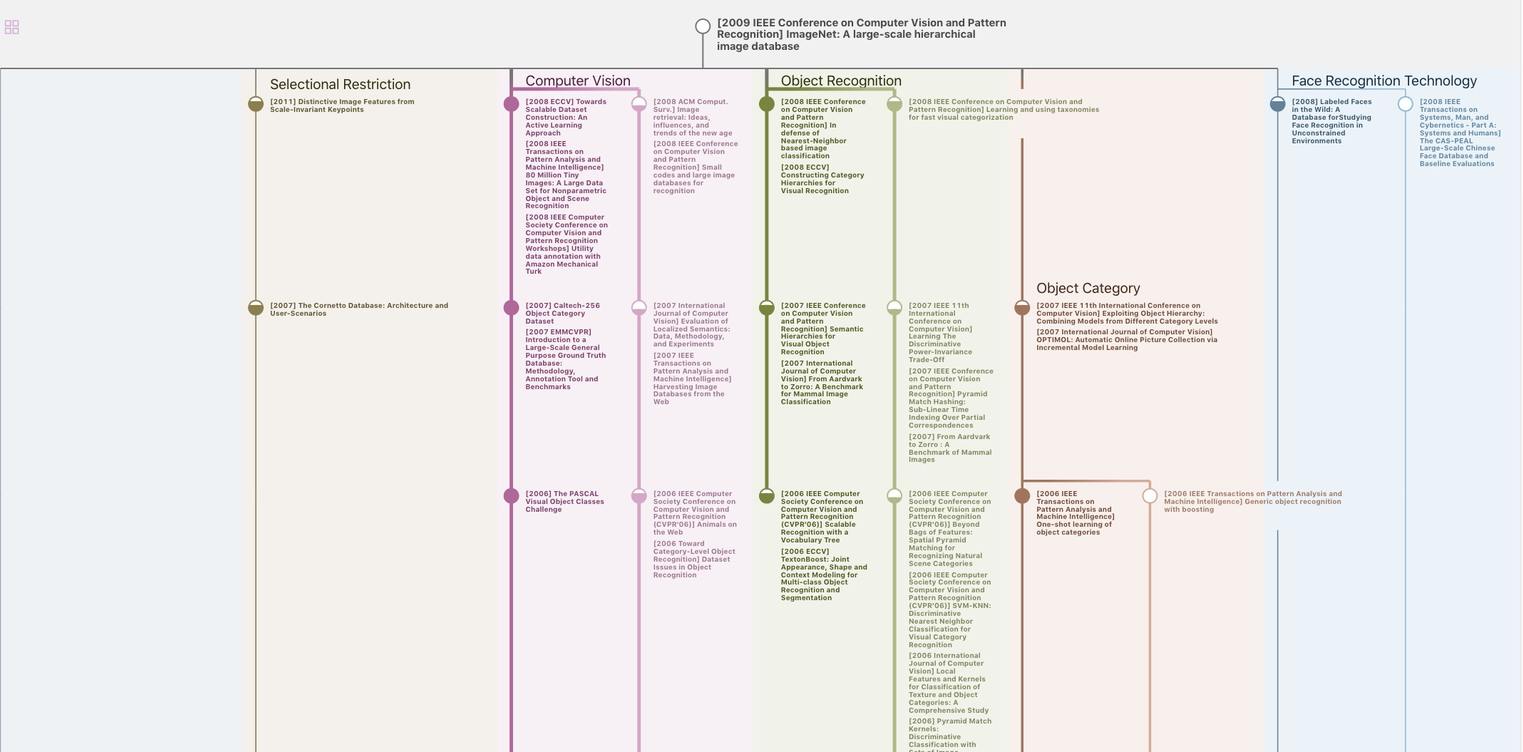
生成溯源树,研究论文发展脉络
Chat Paper
正在生成论文摘要