Recover Meter-Transformer Connectivities Using Geospatial Unsupervised Learning and Q-GIS
2022 IEEE Power & Energy Society General Meeting (PESGM)(2022)
摘要
Deep penetration of distributed energy resources (DERs) and electric vehicles (EVs) introduce benefits, but may cause overloading of service transformers. Such challenges need real-time transformer loading, where an accurate topology is a prerequisite. Previous works attempted to recover the topology as a whole, which is a complicated problem. To simplify the problem, past methods made ideal assumptions of an isolated sub-network and the availability of measurement at every system node. However, for distribution systems, both the assumptions are invalid. To resolve these challenges, we propose to identify the meter-transformer mapping. Due to arbitrary curves in streets, we propose to employ a density-based clustering based on voltage and location information. However, a density-based approach may only be able to localize a meter to a group of few transformers. Hence, we combine the density-based approach with K-means to obtain the meter-transformer mapping. For practical consideration, we provide intuitive usability with Quantum geographic information system (QGIS) software with reduced need for human intervention and memory management for big data. The proposed algorithm has been deployed on real distribution feeders from a northeastern utility in the United States, showing an outstanding performance.
更多查看译文
AI 理解论文
溯源树
样例
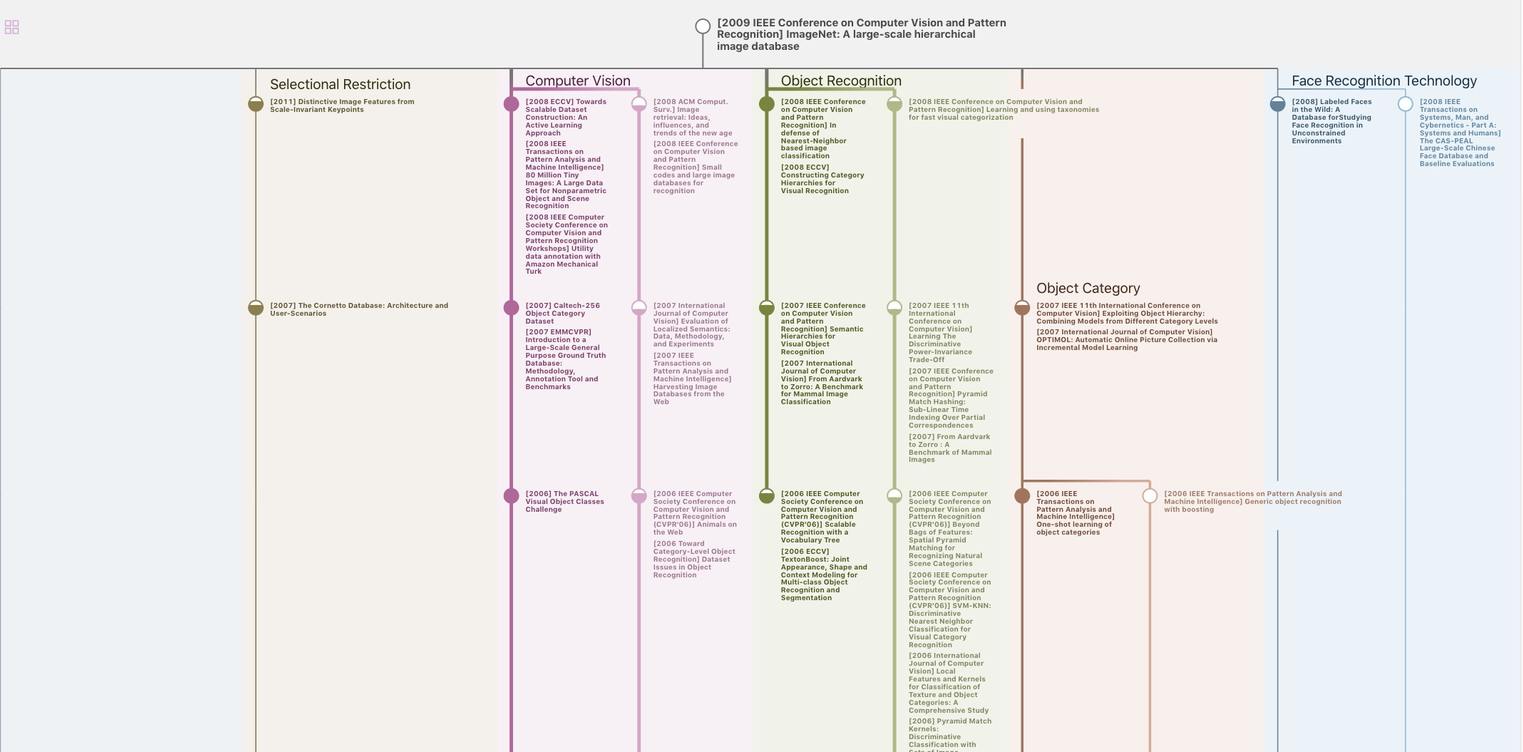
生成溯源树,研究论文发展脉络
Chat Paper
正在生成论文摘要