Machine Learning and Digital Twin-Based Path Planning for AGVs at Automated Container Terminals
Advances in Transdisciplinary EngineeringTransdisciplinarity and the Future of Engineering(2022)
摘要
The complex and dynamic environment in an automated container terminal (ACT) increases the difficulty of path planning, especially for automated guided vehicles (AGVs). Digital twin is an essential means of characterizing complex production systems as the physical objects can be synchronized in the virtual space. Machine learning is also a popular way to solve path planning problems. This study combines digital twin and machine learning to tackle AGV path planning problems in the time-changing operation environment. A digital twin-based AGV scheduling approach is developed to obtain the real-time data from the physical ACT. Based on the information of the dynamic factors obtained, a mathematical model is formulated to minimize the transportation time of AGVs. Subsequently, the path planning schemes solved by machine learning are used asinput to the virtual ACT for validation and optimization. The optimized solution is further compared to a common path plan algorithm without applying digital twin. The experimental results show the superiority of the proposed method, which can provide better decision support for ACT operation.
更多查看译文
关键词
path planning,agvs,machine learning,twin-based
AI 理解论文
溯源树
样例
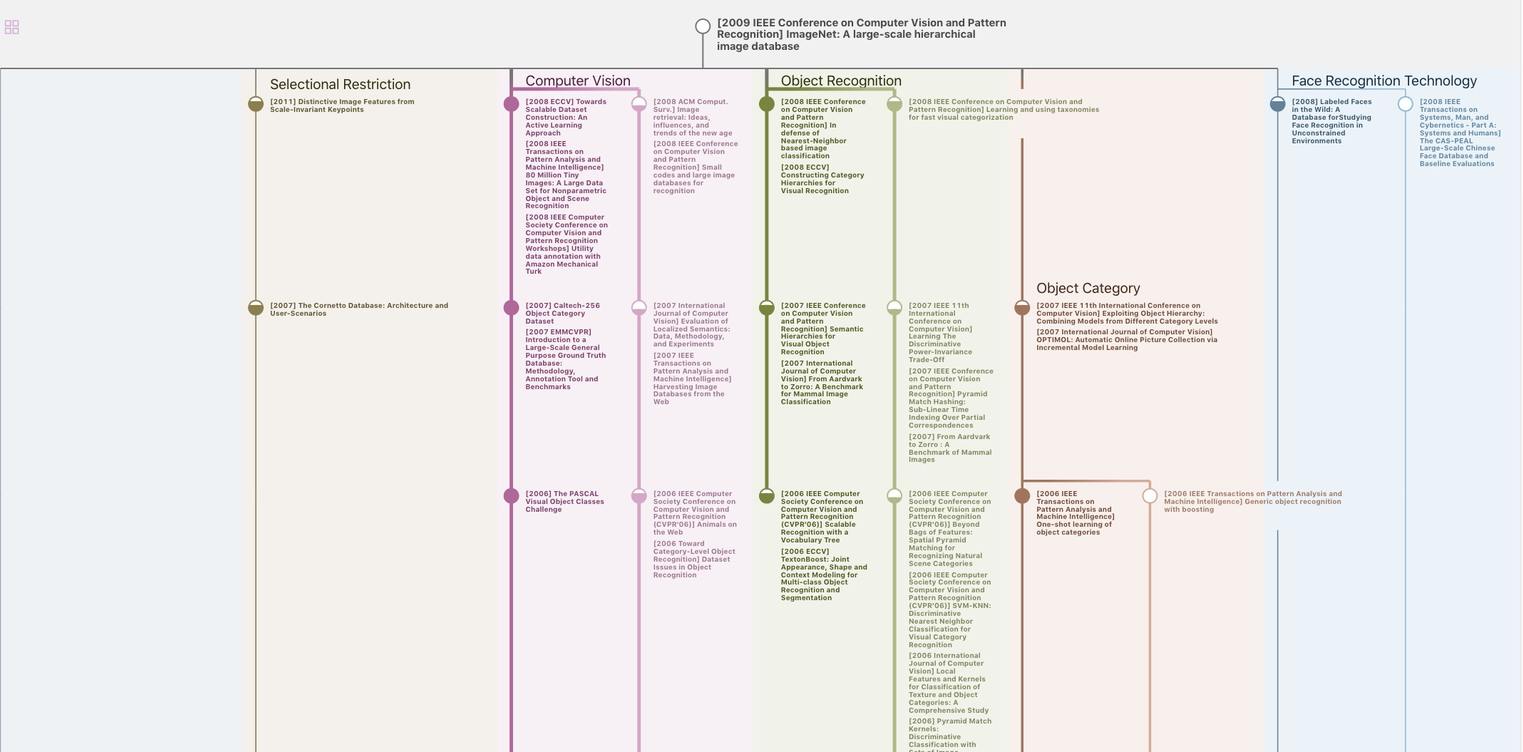
生成溯源树,研究论文发展脉络
Chat Paper
正在生成论文摘要