Patch-based CNN Model for 360 Image Quality Assessment with Adaptive Pooling Strategies
IS&T International Symposium on Electronic Imaging Science and Technology(2022)
摘要
Patch-based training for 360-degree images allows to significantly reduce the complexity compared to multichannel models while maintaining good performances.Differently from multichannel models where multi neural networks are trained in parallel to predict the score of the whole 360-degree image, a pooling stage is required to map local qualities to the global one.This step is often neglected by using a simple arithmetic mean, which does not account for (i) the non-uniformity distribution of quality and (ii) the variability among local qualities.In this paper, we analyze several pooling strategies, including basic statistic methods and adaptive pooling ones.Additionally, we propose a pooling strategy based on scene exploration behavior relying on visual scan-path.The performance analysis showed the benefit of using adaptive pooling over arithmetic mean, as well as the incorporation of perceptual properties during the pooling stage.Besides, the comparison with state-of-the-art multichannel models asserts the effectiveness of patch-based training compared to multichannel models.
更多查看译文
AI 理解论文
溯源树
样例
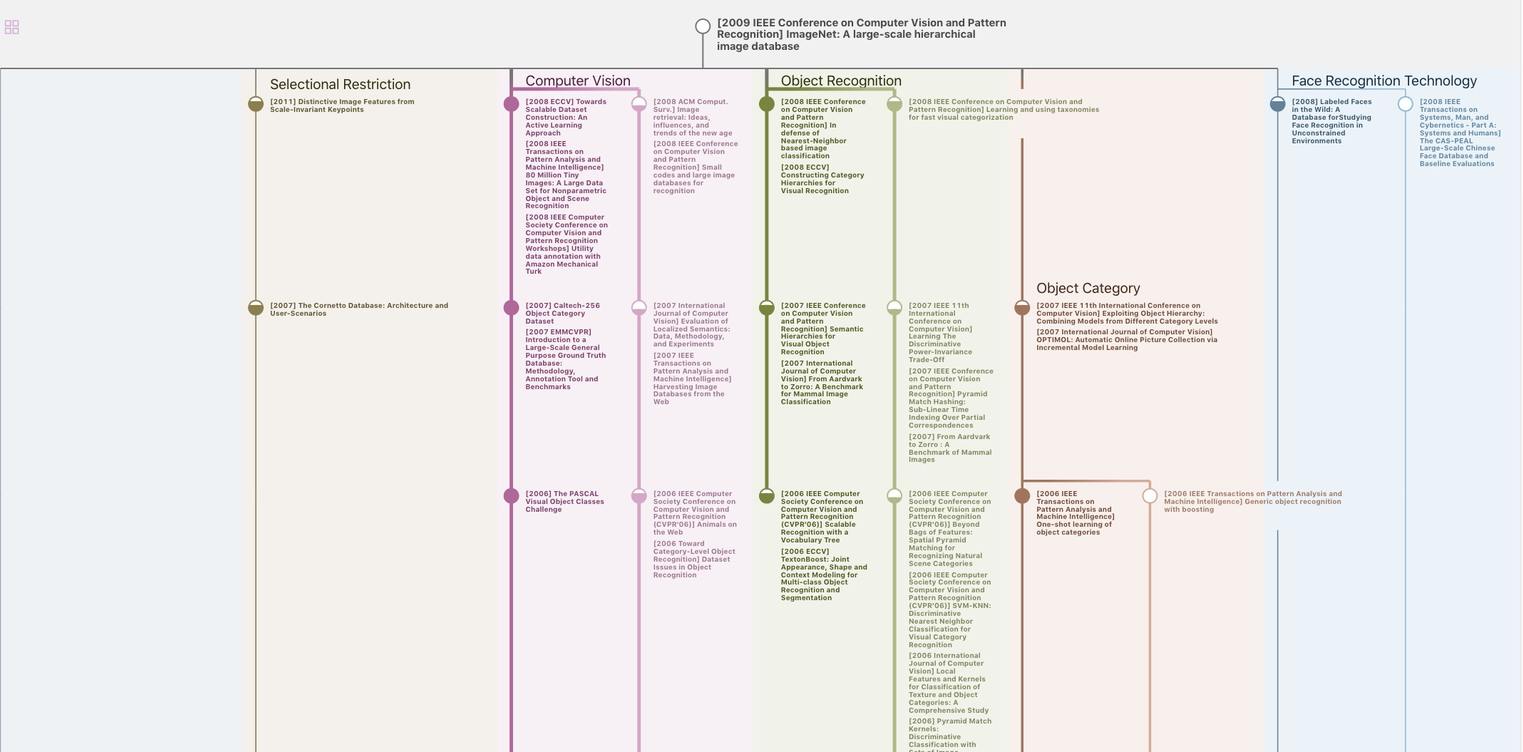
生成溯源树,研究论文发展脉络
Chat Paper
正在生成论文摘要