Regret-Index Neural Network for Finding the Maximum Data Flow of Self-Powered Wireless Sensor Networks
IEEE Sensors Journal(2023)
摘要
There are many complex situations in self-powered wireless sensor networks. First, the signal fluctuation will cause the upper limit of the data transmission volume between the sensor and the base station to change at different times. In addition, with long-term use, equipment failure will cause the loss of some data during transmission, and the rate of data loss is usually undetermined. Based on the above complexity, to determine the maximum data flow of self-powered wireless sensor networks, the fuzzy-wastage time-varying maximum flow problem (FTMFP) is introduced in this article. Existing algorithms are not suitable for solving the FTMFP due to the uncertainty and time variation in the problem. To solve this problem, a regret-index neural network (RINN) algorithm is proposed in this article. The unique minimum regret-index path selection (MRPS) mechanism of the RINN algorithm improves the accuracy when obtaining solutions. The parallel computing and training-free mode of the RINN algorithm improve the computational speed. The Utah channel impulse response (CIR) wireless sensor network and the large-scale New York City road monitoring network are employed to evaluate the performance of the proposed RINN algorithm. The time variance of the data transmission caused by signal fluctuations and the fuzzy loss ratio of data caused by equipment failures are considered in the network. Compared with the well-investigated Ford–Fulkerson (FF), Edmonds–Karp (EK), and Cai–Sha (CS) algorithms, experimental results have proved the effectiveness of the RINN.
更多查看译文
关键词
neural network,maximum data flow,sensor,networks,regret-index,self-powered
AI 理解论文
溯源树
样例
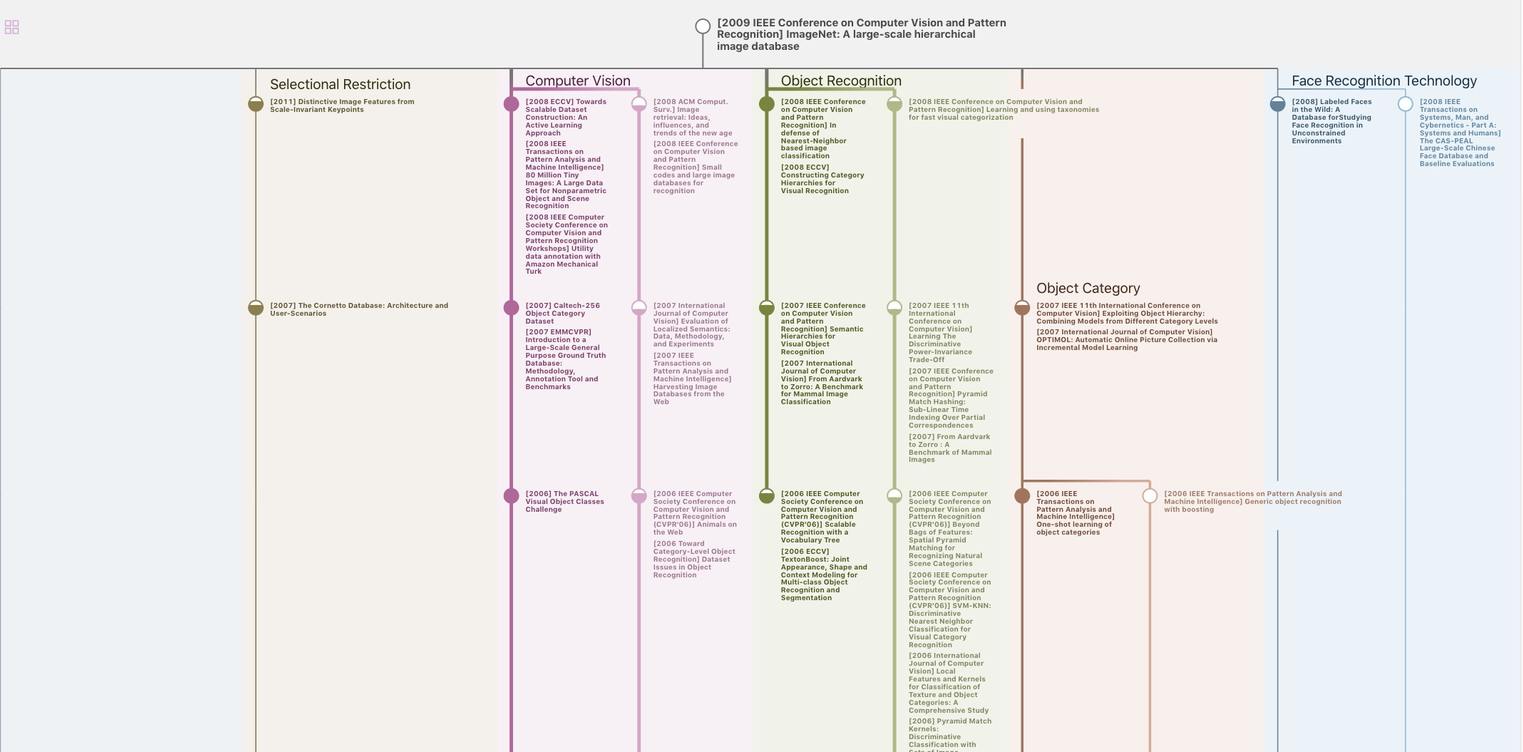
生成溯源树,研究论文发展脉络
Chat Paper
正在生成论文摘要