Quantum chemical data generation as fill-in for reliability enhancement of machine-learning reaction and retrosynthesis planning
DIGITAL DISCOVERY(2023)
摘要
Data-driven synthesis planning has seen remarkable successes in recent years by virtue of modern approaches of artificial intelligence that efficiently exploit vast databases with experimental data on chemical reactions. However, this success story is intimately connected to the availability of existing experimental data. It may well occur in retrosynthetic and synthesis design tasks that predictions in individual steps of a reaction cascade are affected by large uncertainties. In such cases, it will, in general, not be easily possible to provide missing data from autonomously conducted experiments on demand. However, first-principles calculations can, in principle, provide missing data to enhance the confidence of an individual prediction or for model retraining. Here, we demonstrate the feasibility of such an ansatz and examine resource requirements for conducting autonomous first-principles calculations on demand. We demonstrate and discuss the feasibility of autonomous first-principles mechanistic explorations for providing quantum chemical data to enhance the confidence of data-driven retrosynthetic and synthesis design based on molecular transformers.
更多查看译文
AI 理解论文
溯源树
样例
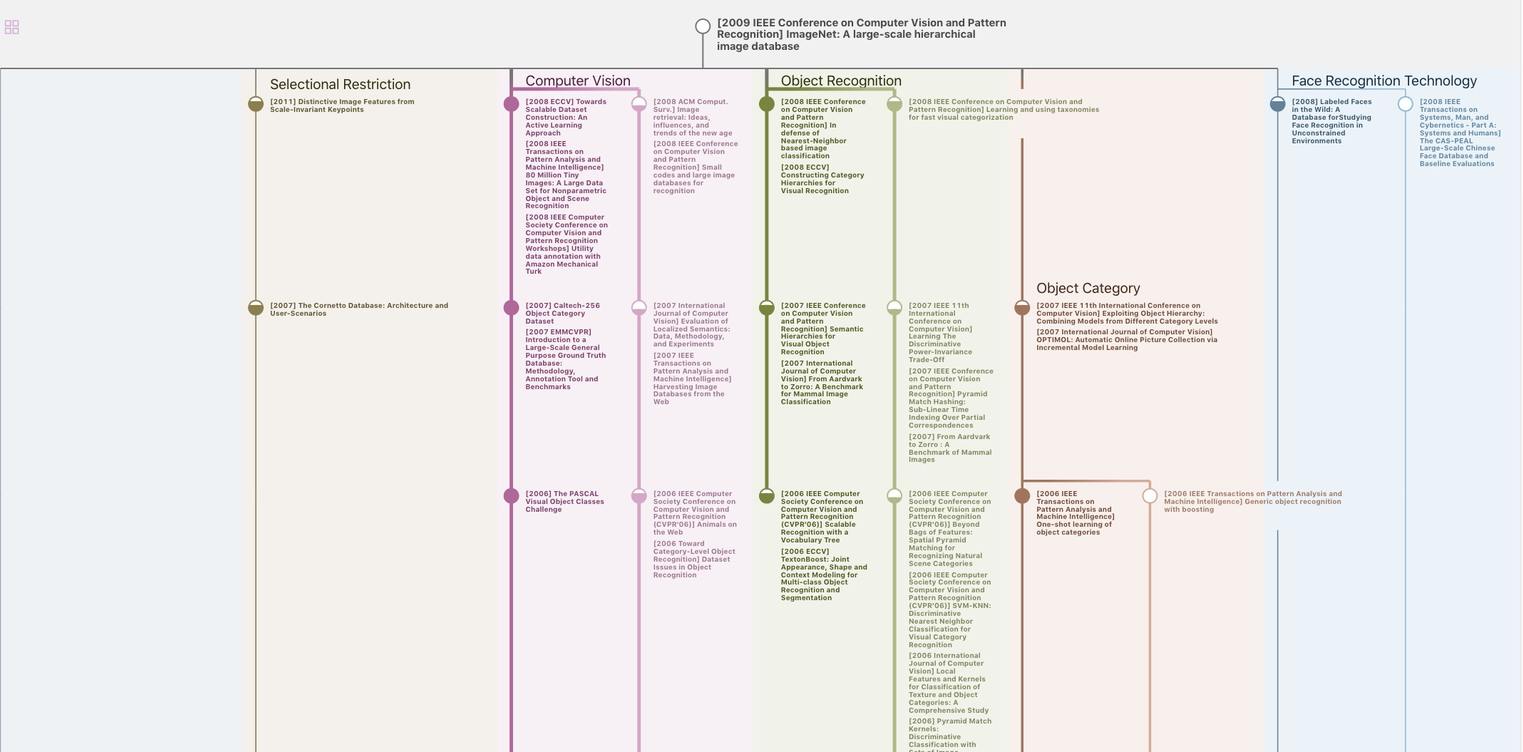
生成溯源树,研究论文发展脉络
Chat Paper
正在生成论文摘要