Deep learning model coupled with proteomics to detect yeast epidemic clones across multiple hospitals
Research Square (Research Square)(2022)
摘要
Abstract The ongoing worldwide emergence of epidemic azole-resistant Candida parapsilosis clones is a threat to human health. In order to monitor a clonal outbreak which involved 2 hospitals in Paris, we used a technology that most clinical laboratories are equipped with: Matrix-Assisted Laser Desorption-Ionization Time of Flight mass spectrometry (MALDI-TOF MS). This physico-chemical analysis technique was combined with machine learning algorithm to evaluate the possibility of monitoring a multi-center epidemic. A total of 96 isolates of Candida parapsilosis constituted of resistant clones, susceptible clones and other susceptible isolates were cultured on various culture conditions. Spectra were acquired on four different mass spectrometers from three hospitals. We used convolutional neural networks (CNN) to classify the isolates and identified the main parameters that can influence the identification of epidemic clonal isolates. We have shown results up to 94% accuracy in a multi-center context, and between 81 and 91% accuracy when the tested site is not part of the training set, showing that it is possible to differentiate the spectra of a clone and a non-clone presenting a very strong similarity. This study shows that it is possible to monitor a certain type of clone, namely the R2 clones in our case study, using MALDI-TOF coupled to CNN. The experimental conditions that impacted the results should be considered for future work to improve performances for difficult classification tasks like clonal fungal identification.
更多查看译文
关键词
yeast epidemic clones,proteomics,deep learning,multiple hospitals
AI 理解论文
溯源树
样例
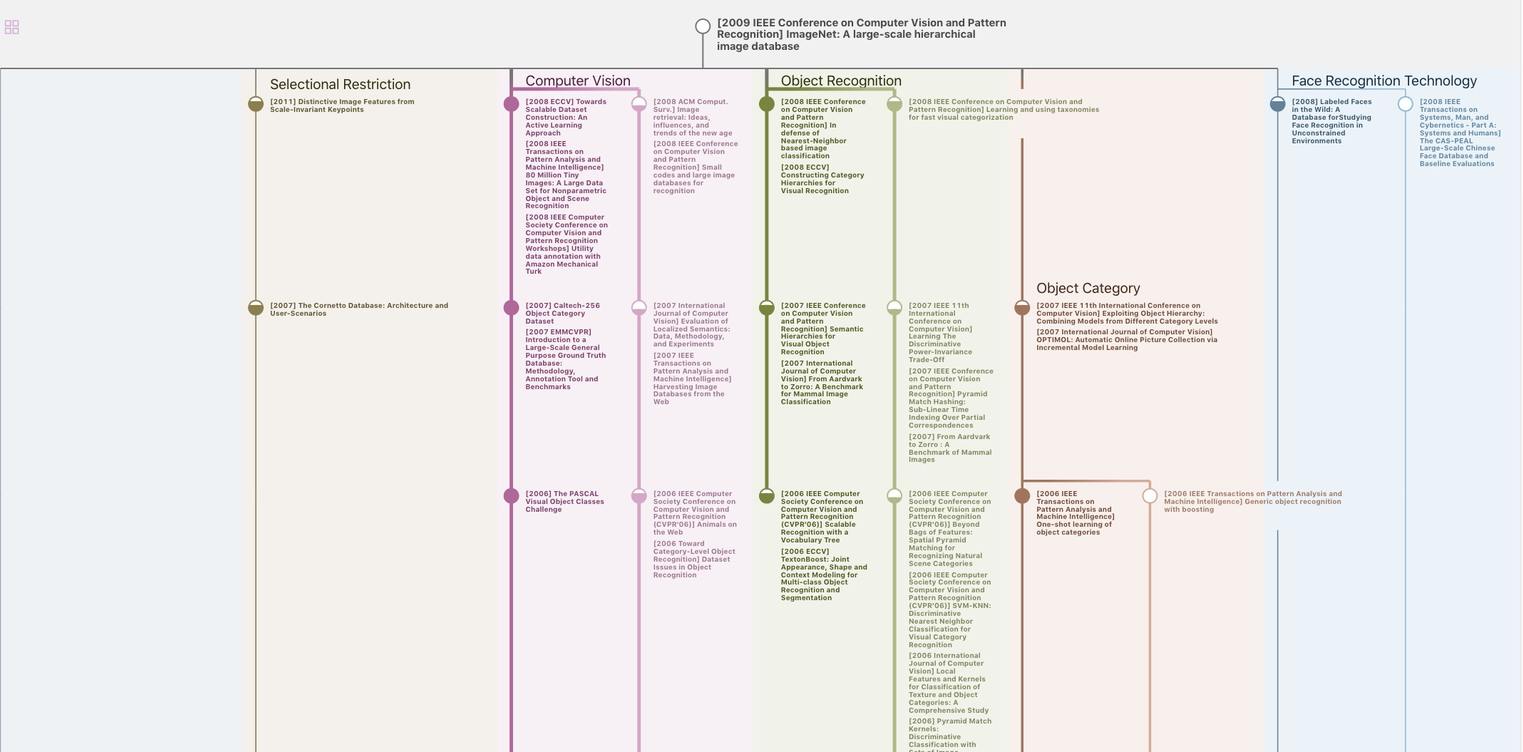
生成溯源树,研究论文发展脉络
Chat Paper
正在生成论文摘要