Predicting Propellant Properties of Boron-Based Hypergolic Ionic Liquids via Machine Learning
crossref(2022)
摘要
Boron-based hypergolic ionic liquids (HILs) have gained increasing attention in the field of propellants due to the low toxicity, high energy density, and short ignition delay time. However, the performance of propellants based on boron-based HILs is still inferior to hydrazine derivatives, restricting their widespread applications as a rocket propellant. To boost the propellant performance of boron-based HILs, constantly engineering their chemical structures is highly necessary. The conventional approaches modify the anions and cations of the ionic liquids based on experiences and heuristics, and then experimentally verify the physicochemical properties of the synthesized compounds. However, such a trial-and-error design cycle is biased by practitioners’ preferences and the variations of experimental conditions. Meanwhile, it is expensive and tedious to measure all relevant properties of the ionic liquids by experiments. To solve these problems, we propose a protocol of combining machine learning and density functional theory (DFT) calculations to predict boron-based HILs’ properties such as ignition delay time, thermal stability, and energy storage capacity. With well-curated data, the experimental results show that the machine learning approaches can satisfactorily predict the chemical properties of boron-based HILs. We also use the Shapley value to find that (1) the thermal correction to enthalpy is critical for ignition delay, (2) HOMO, electronic energy and entropy values have a major impact on decomposition temperature, and (3) electronic energy also has a significant impact on the specific impulse.
更多查看译文
AI 理解论文
溯源树
样例
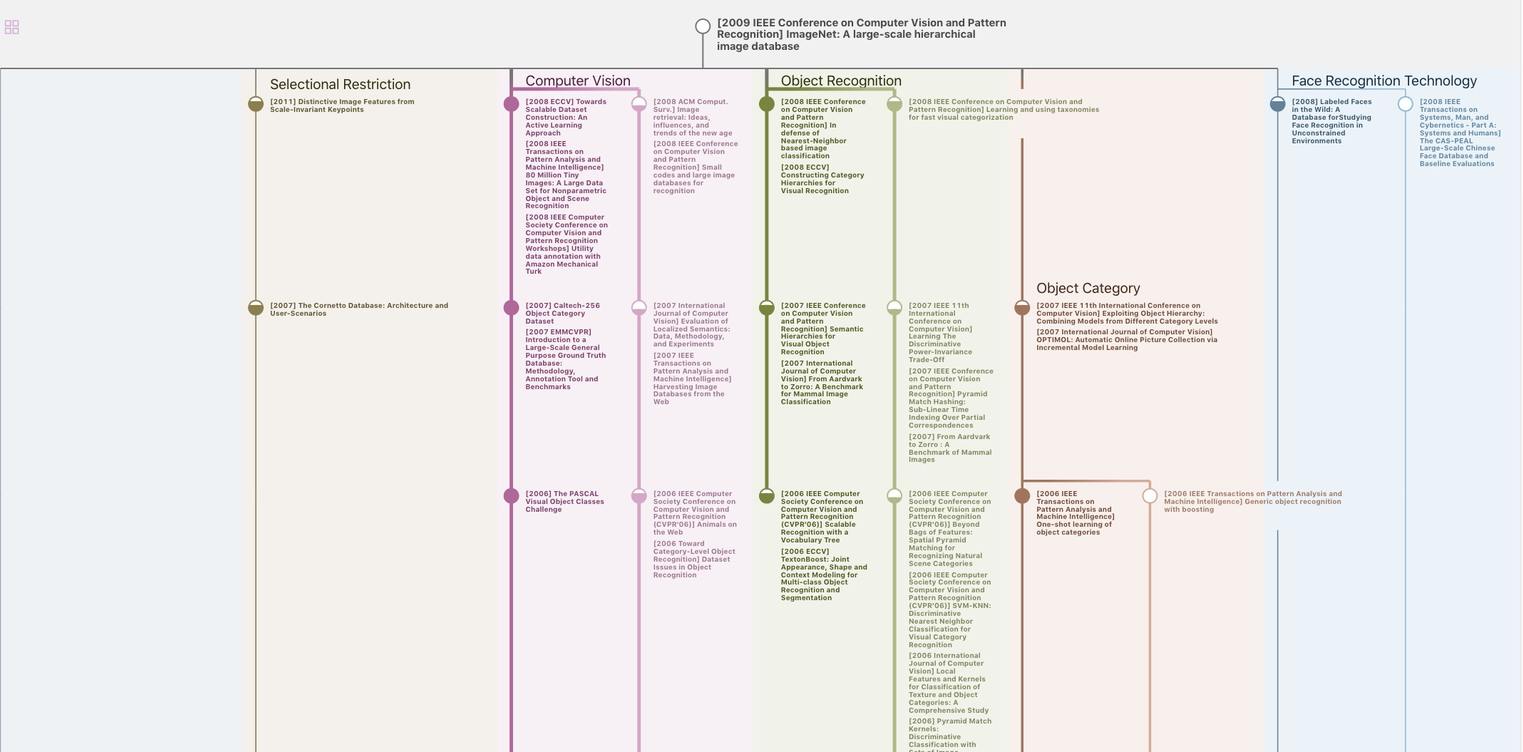
生成溯源树,研究论文发展脉络
Chat Paper
正在生成论文摘要