Variational Quantum Approximate Support Vector Machine With Inference Transfer
crossref(2022)
摘要
Abstract A kernel-based quantum classifier is the most interesting and powerful quantum machine learning technique for hyperlinear classification of complex data, which can be easily realized in shallow-depth quantum circuits such as a SWAP test classifier. A variational quantum approximate support vector machine (VQASVM) can be realized inherently and explicitly on these circuits by introduction of a variational scheme to map the quadratic optimization problem of the support vector machine theory to a quantum-classical variational optimization problem. Probability weight modulation in index qubits of a classifier can designate support vectors among training vectors, which can be achieved with a parameterized quantum circuit (PQC). The classical parameters of PQC is then transferred to many copies of other decision inference circuits. Our VQASVM algorithm is experimented with toy example data sets on cloud-based quantum machines for feasibility evaluation, and numerically investigated to evaluate its performance on a standard iris flower and MNIST data set. The empirical run-time complexity of VQASVM is estimated to be sub-quadratic on the training data set size, while that of the classical solver is quadratic.
更多查看译文
AI 理解论文
溯源树
样例
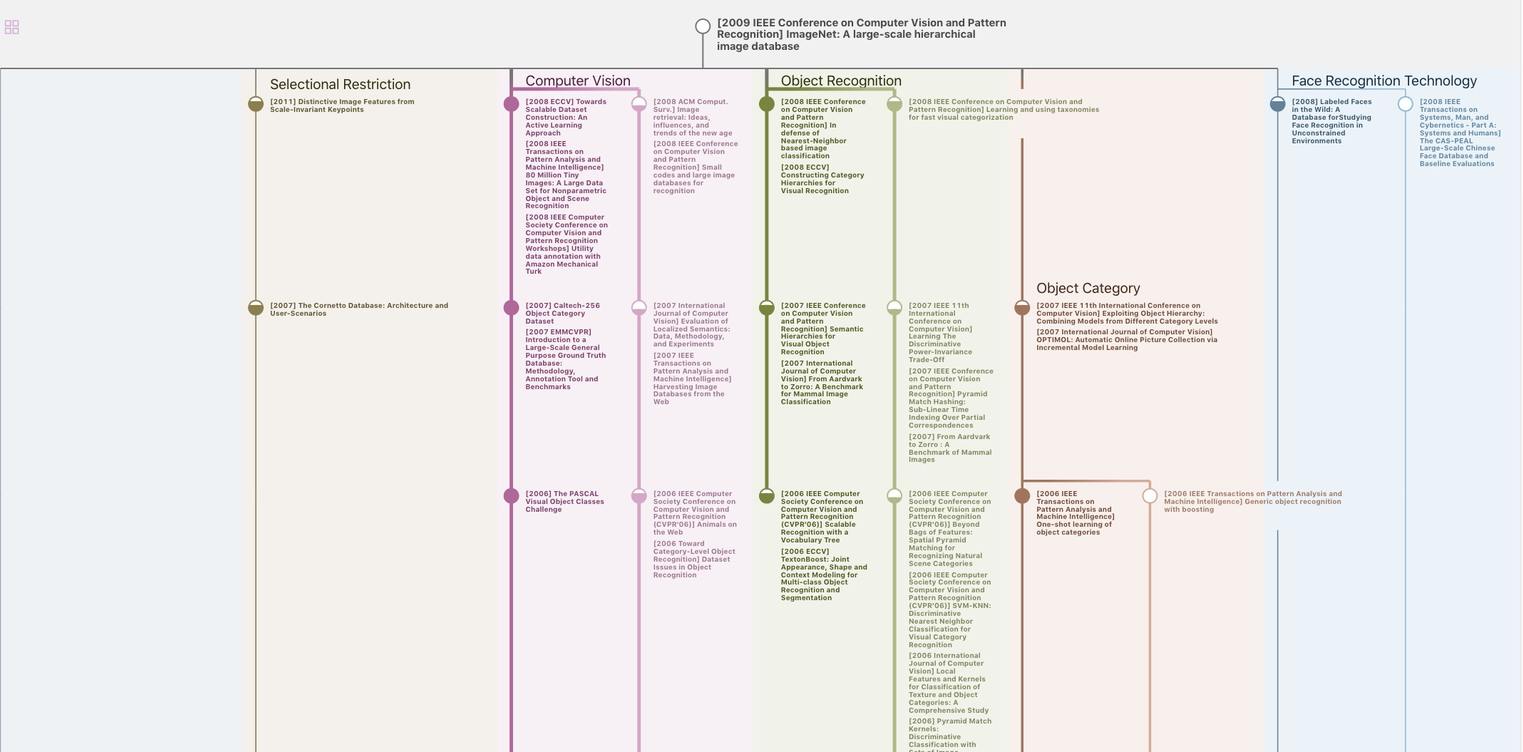
生成溯源树,研究论文发展脉络
Chat Paper
正在生成论文摘要