Machine learning in nanomaterial electron microscopy data analysis
Intelligent Nanotechnology(2023)
摘要
The morphology of nanomaterials plays important roles in determining their macroscopic properties and functions. While traditional characterization techniques such as small-angle X-ray scattering probes the ensemble-averaged material feature length scale, they fail to provide information on structural heterogeneity. The real-space imaging techniques including scanning/transmission electron microscopy (S/TEM), scanning electron microscopy (SEM), and atomic force microscopy (AFM) can directly visualize the nanomaterial structures, providing the most intuitive information. However, real-space images focus on a local area instead of characterizing the whole sample, and thus usually work as a qualitative and supplementary technique in nanomaterial research. To comprehensively characterize the whole sample, one should take multiple microscope images from different locations and extract the information of interest in a quantitative and efficient way. The recently developed real-time imaging techniques, for example, liquid-phase TEM (LP-TEM), enable the ability to study nanomorphology change overtime and nanoparticle diffusion and self-assembly. Such technique generates videos, or stacks of images, which usually contains hundreds of frames and thus also requires efficient methods to locate and extract the features. Unlike the spectroscopy data, a real-space image is high dimensional, and the features inside are variant to translation, rotation, and scaling, which makes it inapplicable to treat images as a feature vector, retrieving information from fixed positions or fitting them into simple statistical algorithms. The quantitative analysis of nanomaterial microscope images usually starts from feature recognition, either by hand annotation or image processing algorithms (e.g., filtering and thresholding). While hand annotation by expert researchers is believed to be accurate, it only works on a small volume of data due to its low efficiency. On the other hand, most conventional image processing algorithms are less “smart,” which struggles to find various features of interest buried under the complicated contrast profile and inevitable noises in the images. Machine learning (ML) is a fast-developing area in computer science in the past decade and a lot of ML models are proven to be capable for classification, feature detection, segmentation, and trajectory tracking of real-life pictures. Efforts have also been put into applying, adapting, and designing ML models for scientific image data and show excellent performance in analyzing biomedical and optical microscope images. In this chapter, the recent progresses on ML applications in processing and analyzing microscopy data of nanomaterials will be reviewed with a wide range of different imaging techniques, data type (images, videos, and tomographs), and ML models covered.
更多查看译文
AI 理解论文
溯源树
样例
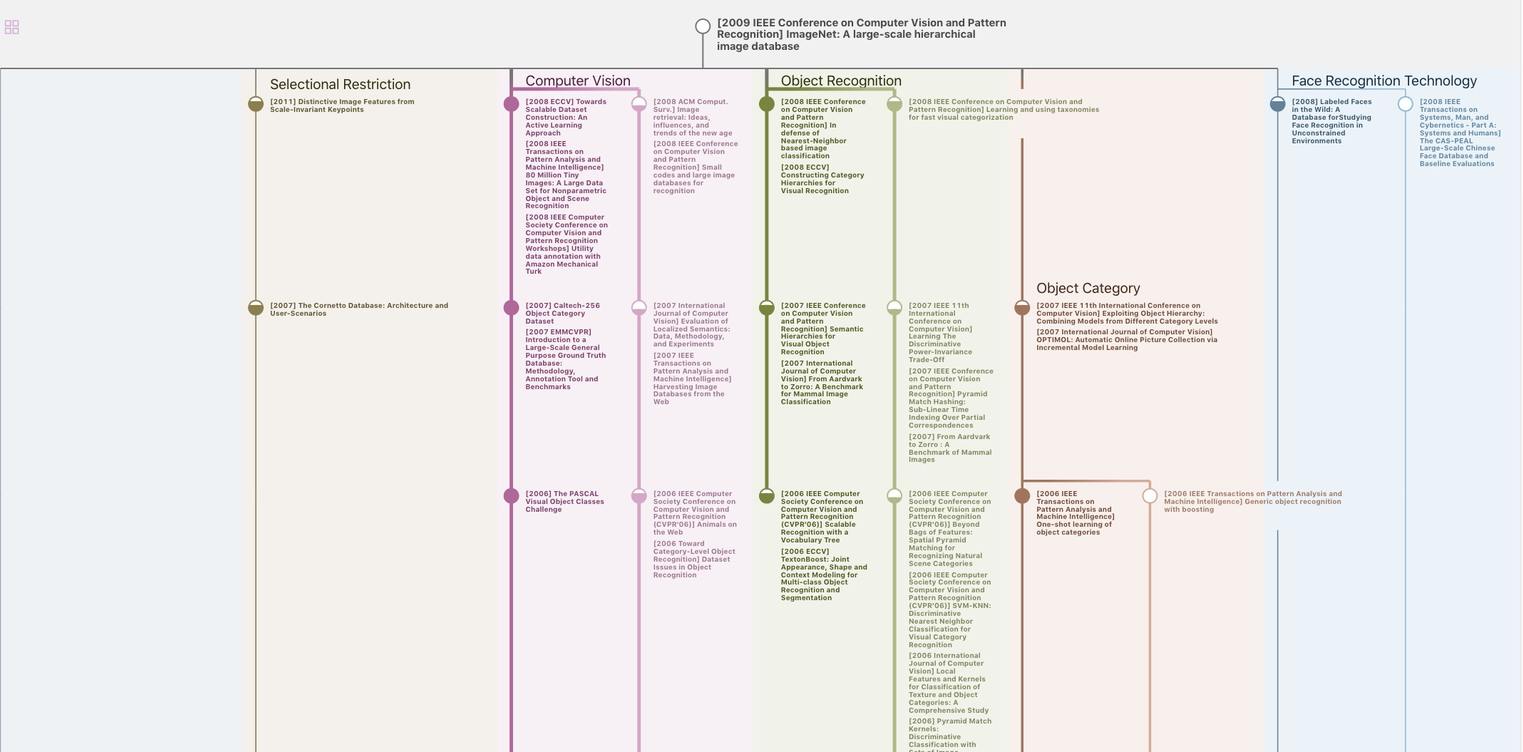
生成溯源树,研究论文发展脉络
Chat Paper
正在生成论文摘要