Spatio-Temporal Features for Fast Early Warning of Unplanned Self-Extubation in Icu
Engineering applications of artificial intelligence(2024)
摘要
Patients’ behaviors in the Intensive Care Units (ICU) have garnered research attention, particularly regarding the impact of Unplanned Extubation (UEX). However, there is currently no existing report on methods for early warning of UEX action in RGB video. Applying traditional human action recognition algorithms to UEX in the complex ICU environment proves challenging. To address the above issue, we propose a novel feature for early warning of UEX action in patients using RGB videos. Firstly, we employ the YOLOv3 detection method to extract the region of interest (ROI), which corresponds to the region where the patient is located. Subsequently, we develop a spatio-temporal (ST) feature for human action tracking by using the L-K optical flow algorithm. This ST feature encompasses optical flow corner number, trajectory distance, and wavelet transform features. Finally, we utilize support vector machine (SVM) for patient action classification and early warning. Experimental results on the ICU monitoring dataset demonstrate the superior performance of the proposed feature in UEX prediction.
更多查看译文
关键词
Unplanned extubation,Action recognition,Early warning,Spatio-temporal feature,L-K optical flow
AI 理解论文
溯源树
样例
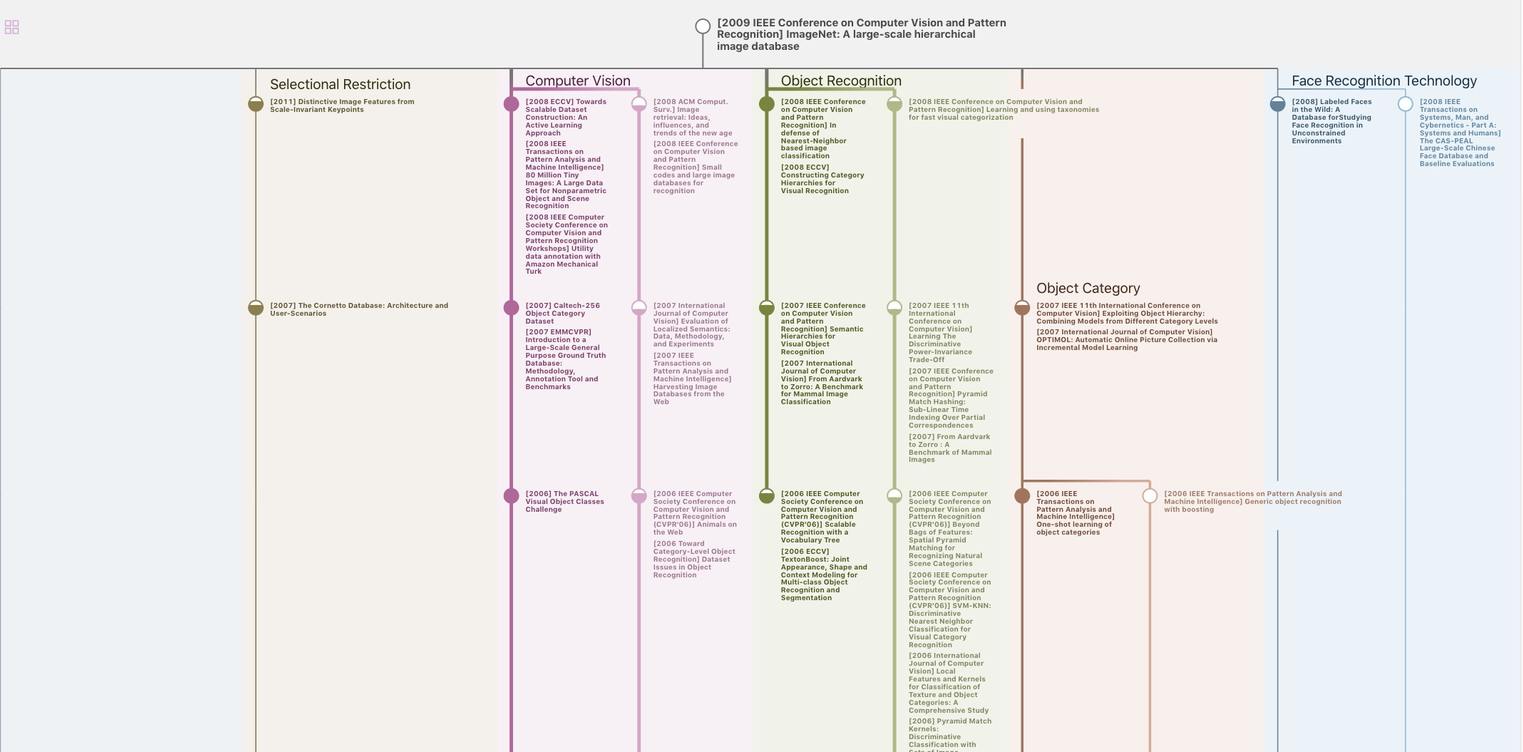
生成溯源树,研究论文发展脉络
Chat Paper
正在生成论文摘要