Graph Inductive Inference of Personalized Content for Out-of-matrix Users Via Content Baskets
Research Square (Research Square)(2022)
摘要
Abstract Guest users are common in real world applications, requiring industrial recommendation systems to handle the "cold start" problem, where no existing interactions between new users and recommendable items can be drawn from to make predictions. Prior work addresses this problem by learning profiling user representations to bootstrap recommendations for new users. However, this process can often be invasive, requiring new users to submit personal data, or shallow, yielding unexpressive representations for accurate recommendations. In this work, we propose new representations for guest users based on their ``content basket’'. A set of seed items is submitted by the user to use the service, allowing each user to be represented as a function of a collection of items. Simultaneously, we design a graph representation space, where items (nodes) are connected by edges that signify joint, written recommendations between items. We propose a graph neural network architecture that inductively learns item and inter-item (edge) representations as a combination of deep language encodings of textual content descriptions and graph embeddings learned via message passing on the edges. This enables effective generalization to items unseen during training. To evaluate our model and demonstrate a novel application, we present a new dataset for anime recommendations, AnimeULike, containing anonymized interactions between ~13k users and 10k animes, and the accompanying recommendation engine which can exclusively serve guest users. Our empirical results on AnimeULike and a standard recommender systems benchmark demonstrate significant performance improvements over previous cold start solutions that do not learn to dynamically represent new users.
更多查看译文
关键词
personalized content,inference,out-of-matrix
AI 理解论文
溯源树
样例
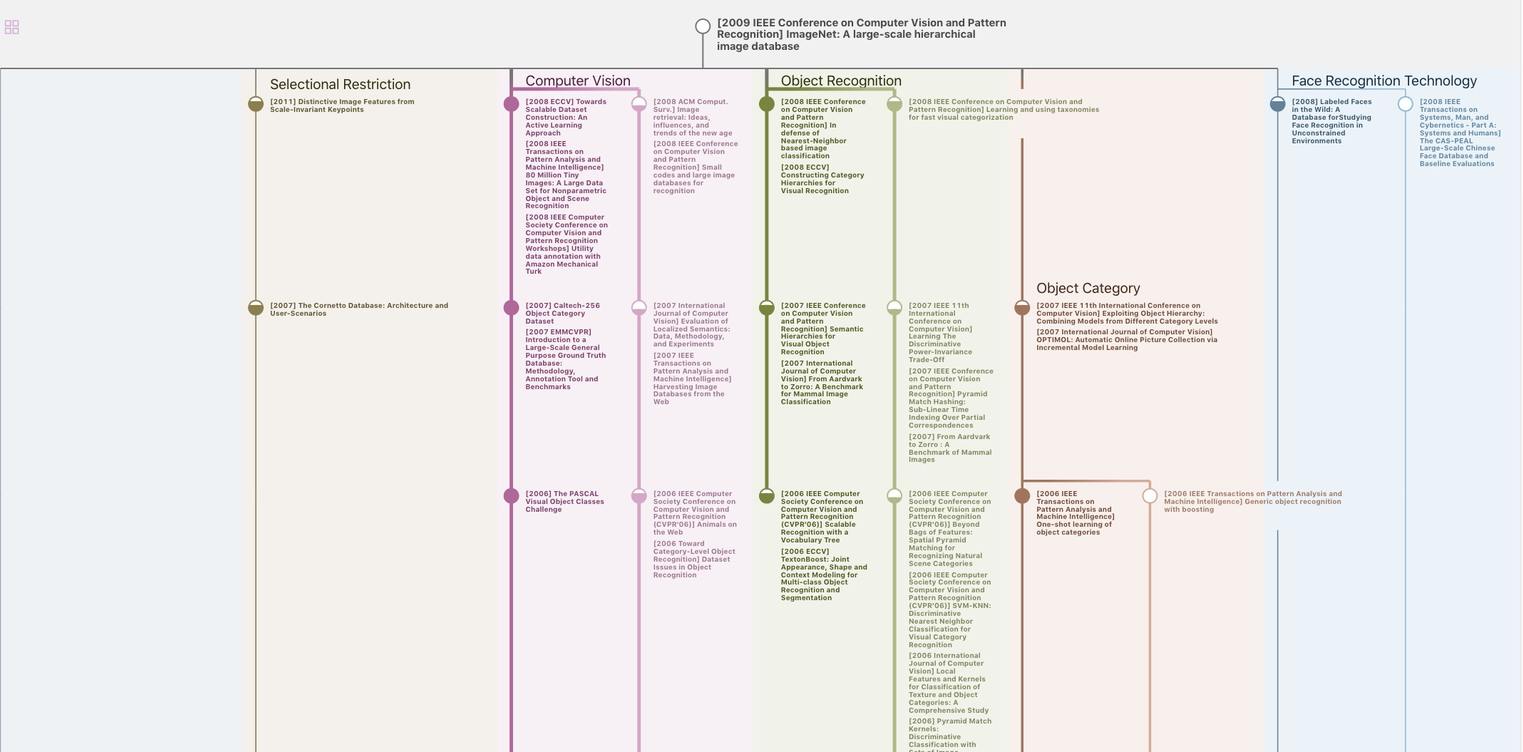
生成溯源树,研究论文发展脉络
Chat Paper
正在生成论文摘要