Detection of Tuberculosis in Microscopy Images Using Mask Region Convolutional Neural Network
Computational Intelligence in Image and Video Processing(2023)
摘要
The early diagnosis of tuberculosis carries vital importance as this can spread from person to person. In developing countries, the diagnosis is mainly carried out through the Ziehl-Neelsen stained sputum smear test as the test provides a relatively simple and inexpensive approach. However, diagnosis through manual techniques is time-consuming and susceptible to fault because of the associated workload and the lack of trained technicians. In this chapter, a deep learning method for automated bacilli detection and segmentation is presented. The method uses the mask region convolution neural network-a recently developed segmentation model. Experiments are performed on the ZNSM-iDB dataset with suitable customization and labeling for ground truth preparation. Evaluation is done in terms of accuracy (98%), recall (98%), precision (86%), and F1-score (91.6%). Results of the work are also compared with the U-Net model. The method can automate the detection of tuberculosis bacilli in microscopy images. In addition, it can help to reduce the workload of a laboratory technician, decrease chances of human error, and enhance the sputum smear test performance. Furthermore, an added value is provided by the instance segmentation which makes the bacilli detection explainable and traceable.
更多查看译文
关键词
microscopy images,tuberculosis,convolutional neural network
AI 理解论文
溯源树
样例
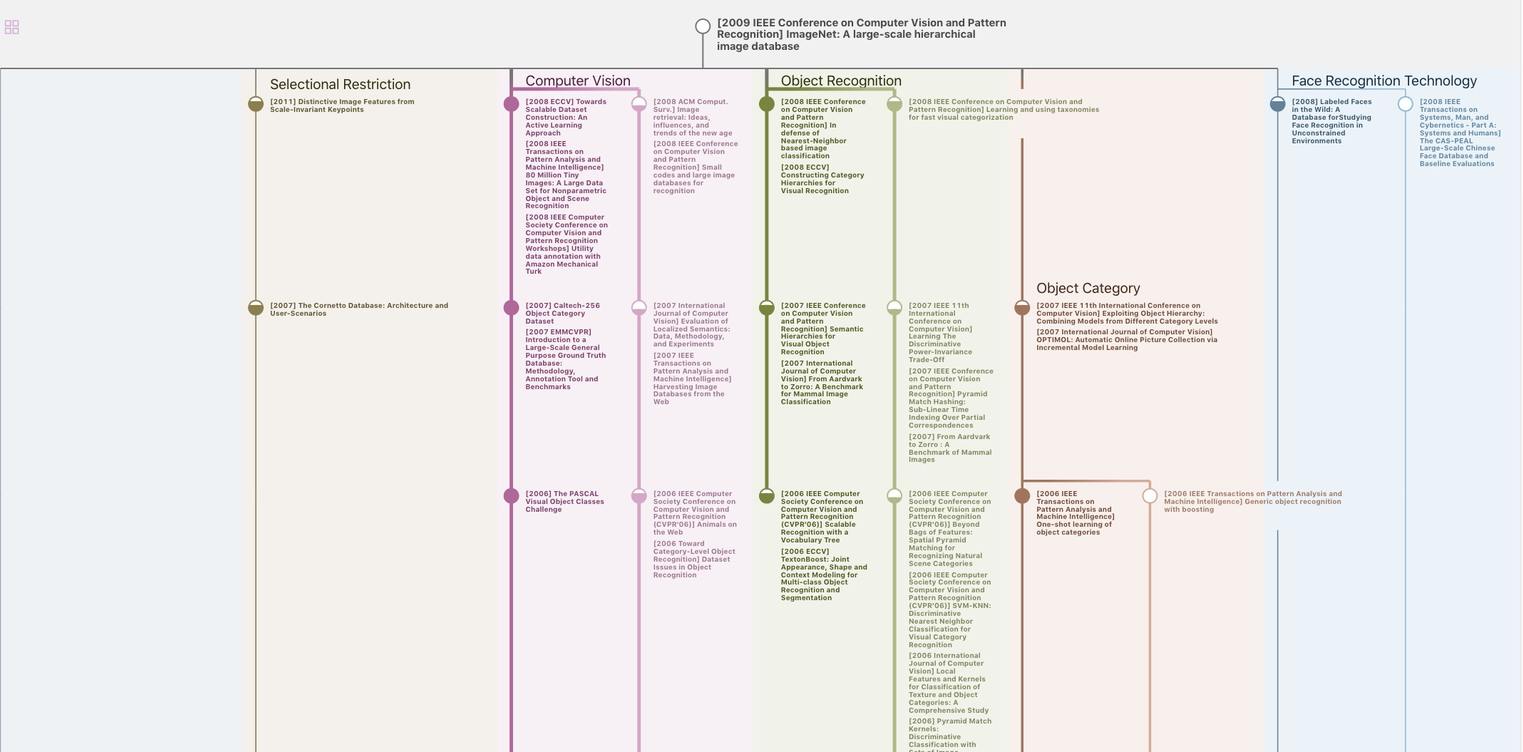
生成溯源树,研究论文发展脉络
Chat Paper
正在生成论文摘要