Acquisition and Analysis of Crowd-Sensed Traffic Data at Multiple Spatial Scales
ISEE Conference Abstracts(2022)
摘要
BACKGROUND/AIM: Measuring traffic activity at high spatial (e.g., street level) and temporal (e.g., sub-hourly) resolution is critical for studying traffic-related exposures and evaluating the efficacy of traffic-reduction interventions, yet such data is not easily available. Crowd-sensed traffic data offer great promise to fill this resource gap, but often require complex collection pipelines, image processing, and GIS manipulation before use in exposure, health, or policy evaluations. We present a validated approach for acquiring and analyzing timeseries of crowd-sensed traffic data, at multiple spatial scales. METHODS: We developed software to automatically download tiles of crowd-sensed Google traffic congestion maps for a user-specifiable region of interest. To support research, we used image-processing to identify the relevant features of interest (i.e., the congestion-color-coded streets), and aggregate the traffic data to user-specified time scales and spatial polygons (e.g., census tracts, zip codes). We performed quality assurance to assess the validity and reproducibility of our approach. RESULTS: We demonstrate broad and international applicability of our method with examples of Manhattan in New York City and Mexico City. We also illustrate that the data has sufficient resolution and sensitivity to quantify decreases in traffic congestion due to COVID-19-related social distancing policies in the South Bronx in New York City. Our analysis identified decreases at the census tract level as well as for individual street segments, using traffic data at three-hour increments. CONCLUSIONS: The methods presented here are broadly scalable and easy to replicate by other researchers across the globe, including communities with limited on-the-ground monitoring. Crowd-sensed traffic data have great promise for empirically modeling traffic congestion, evaluating interventions, assessing exposure for epidemiologic studies, and combining with other neighborhood level characteristics for environmental justice evaluations and other studies. KEYWORDS: crowd-sensed data; traffic congestion; modeling; spatial scales
更多查看译文
关键词
multiple spatial scales,data,crowd-sensed
AI 理解论文
溯源树
样例
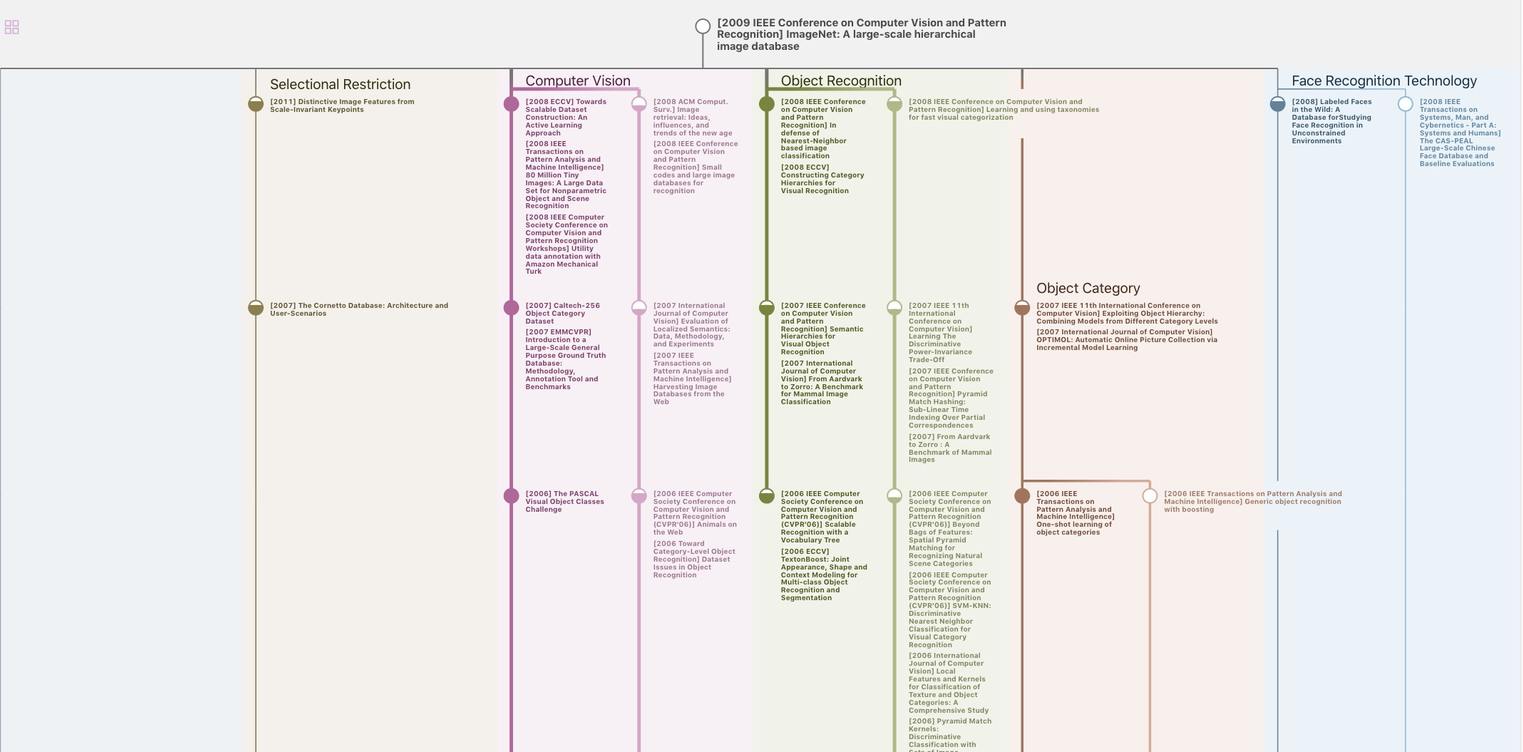
生成溯源树,研究论文发展脉络
Chat Paper
正在生成论文摘要