Validation of a Deep Machine Learning Tool to Determine Intra-Procedural Screening Colonoscopy Quality Indicators in an Academic Health System
American Journal of Gastroenterology(2022)
摘要
Introduction: High quality screening colonoscopy is the hallmark of effective colorectal cancer (CRC) prevention. However, continuously monitoring colonoscopy quality indicators for providers and health systems remains a challenge. We developed and validated a natural language processing (NLP) tool to automatically measure 4 intraprocedural colonoscopy quality improvement (QI) metrics and characterized its performance. Methods: We implemented this quality initiative in a large academic healthcare system that performs >15,100 screening colonoscopies yearly in 6 endoscopy centers. We trained and developed an NLP algorithm that extracts and analyzes data from free-text colonoscopy reports to measure colonoscopy indication (IND), bowel preparation (BP), cecal intubation (CI), and successful cecal intubation (SCI) (Figure). We then randomly selected 600 screening colonoscopies performed between 6/2020-2/2021 to validate the NLP’s performance. We compared the NLP-derived quality metrics to manual chart review (gold standard). We calculated the sensitivity, specificity, positive predictive value, negative predictive value, F-score, and accuracy for each metric. When NLP and manual review were discordant, another physician repeated manual review to resolve the discrepancy. Results: Our validation cohort (n=600) was 49.2% female with mean age 61.5 (sd=8.9, Table). Overall, the NLP had excellent performance across all four evaluated quality metrics when compared to manual chart review. For all metrics, sensitivity ranged from 99.3 to 100.0% and specificity ranged from 94.3 to 100.0% (Table). Within our cohort, the NLP misclassified only 2 cases for the documentation of IND. For documentation of BP, the NLP misclassified 1 case. Both misclassifications (IND and BP) were due to conflicting documentation by the endoscopist in the same colonoscopy report. The NLP had perfect performance for the documentation of CI. Finally, for SCI, NLP misclassified 12 cases, mainly due to the endoscopist not mentioning the word “cecum” or documenting “terminal ileum” instead. Conclusion: We developed an automated NLP algorithm that is highly accurate and sensitive in determining four priority intraprocedural colonoscopy quality indicators. Metrics from this tool can inform where to invest resources to further improve QI measures. In the future, we hope to optimize the NLP performance, measure additional colonoscopy quality metrics, and disseminate the NLP algorithm to other health systems to improve CRC outcomes.Figure 1.: Schematic of the natural language processing pipeline. This diagram depicts a model of how the NLP algorithm process data. All new colonoscopy reports are automatically imported daily into our neural network. Relevant information is then identified and labeled appropriately converting free text into a structured format. The data extracted by the NLP enables downstream analyses and interpretation of the quality indicators Table 1. - Performance of the NLP for 4 quality metrics: (1) colonoscopy indication, (2) bowel preparation, (3) cecal intubation, and (4) successful cecal intubation; N=600 Documentation of colonoscopy indication (IND) Manual review Natural Language Processor (NLP) Total “Screening” detected “Screening” not detected “Screening” detected 314 0 314 “Screening” not detected 2 284 286 Total 316 284 600 Test characteristics Sensitivity 99.3% Specificity 100% Positive Predictive Value (PPV) 100% Negative Predictive Value (NPV) 99.4% F1-score* 0.996 Accuracy 99.7% Documentation of bowel preparation (BP) Manual review NLP Total BP documented BP not documented BP documented 599 0 599 BP not documented 1 9 1 Total 600 0 600 Test characteristics Sensitivity 100% Specificity 97.5% PPV 99.8% NPV N/A F1-score* 0.999 Accuracy 99.8% Documentation of cecal intubation (CI) Manual review NLP Total CI documented CI not documented CI documented 599 0 599 CI not documented 0 1 1 Total 599 1 600 Test characteristics Sensitivity 100% Specificity 100% PPV 100% NPV 100% F1-score* 1 Accuracy 100% Documentation of successful cecal intubation (SCI) Manual review NLP Total SCI documented SCI not documented SCI documented 437 3 440 SCI not documented 9 150 159 Total 446 153 599 Test characteristics Sensitivity 99.3% Specificity 94.3% PPV 98.0% NPV 98.0% F1-score* 0.987 Accuracy 98.0% Abbreviations: NLP: natural language processor; IND: colonoscopy indication; BP: documentation of bowel preparation; CI: cecal intubation; SCI: successful cecal intubation; PPV: positive predictive value; NPV: negative predictive value.*The F1-score combines the precision and recall of a classifier into a single metric by taking their harmonic mean.
更多查看译文
关键词
deep machine learning tool,screening,validation,machine learning,academic health system,intra-procedural
AI 理解论文
溯源树
样例
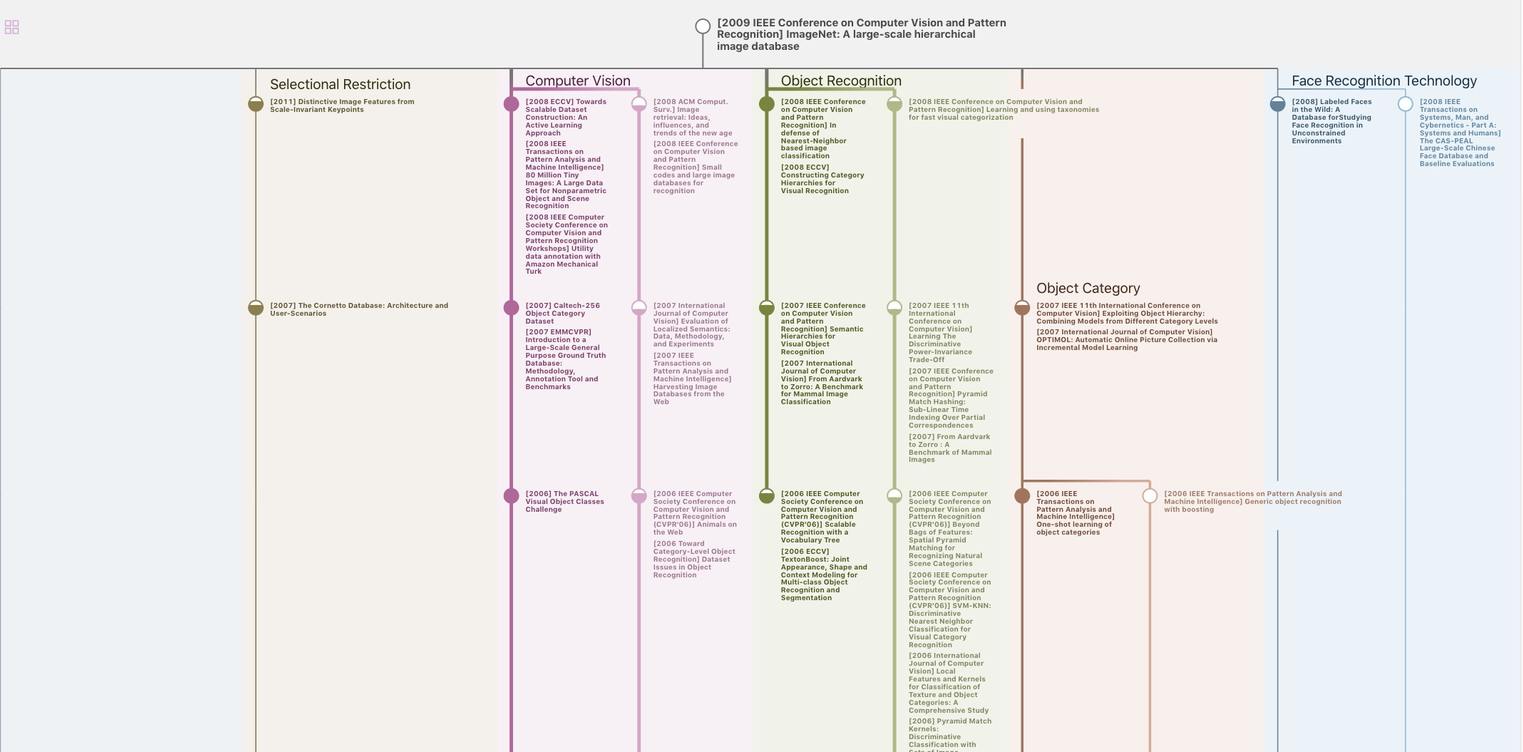
生成溯源树,研究论文发展脉络
Chat Paper
正在生成论文摘要