Unsupervised direct generation of defect residual images forfabric defect detection
crossref(2022)
摘要
Abstract When performing fabric defect detection, ground truth is required for training with supervisedlearning, more steps are required for training with unsupervised learning, and background noise isgenerated during the training process. To solve the above problems, we propose the fabric defectdetection model with unsupervised direct defect residual image generation (UDDGAN). The gener-ative adversarial network model architecture is used in the main body of the model, and we designthe patch structure such that the defect residual images can be generated directly. We use a gen-erator with block blocks and a double discriminator to make the generated image closer to thetarget image. We incorporate similar image loss when training the generator to minimize the gen-erated background noise, which ensures the accuracy of the detection results. We achieve betterresults on a benchmark dataset for fabric defect detection at Zhejiang University and compare itwith six methods. The experimental results show that our method works well on a variety of metrics.
更多查看译文
AI 理解论文
溯源树
样例
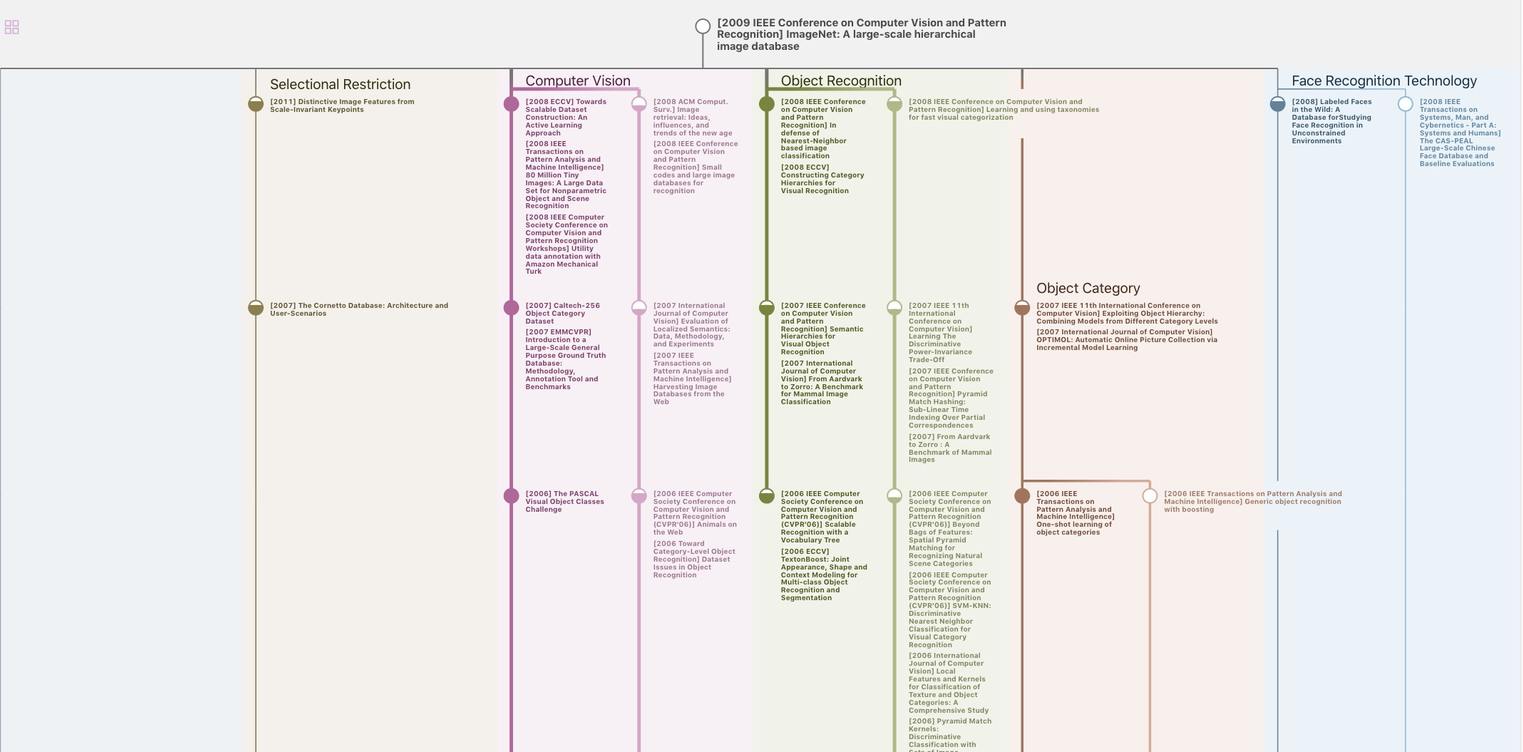
生成溯源树,研究论文发展脉络
Chat Paper
正在生成论文摘要