Federated Dynamic Spectrum Access through Multi-Agent Deep Reinforcement Learning
GLOBECOM 2022 - 2022 IEEE Global Communications Conference(2022)
摘要
Dynamic spectrum access (DSA) has emerged as a promising solution for spectrum usage enhancement by allowing opportunistic access of secondary users to the licensed spectrum. In this paper, we introduce Fed-MADRL, a collaborative DSA technique that exploits both federated learning (FL) and multiagent deep reinforcement learning (MADRL). FL allows numerous users to collaborate on the system goal optimization without sharing their training data. By keeping all training data at the user's end, FL simultaneously enhances communication efficiency and protects data privacy. To further reduce communication costs, each user in Fed-MADRL only shares quantized data. To the best of our knowledge, Fed-MADRL is the first effort that employs FL in DSA networks with quantized communication. Simulation results show that the introduced Fed-MADRL approach beats the independent learning method and provides comparable results to the synchronous FL method, which involves significantly greater communication overheads.
更多查看译文
关键词
federated dynamic spectrum access,reinforcement learning,multi-agent
AI 理解论文
溯源树
样例
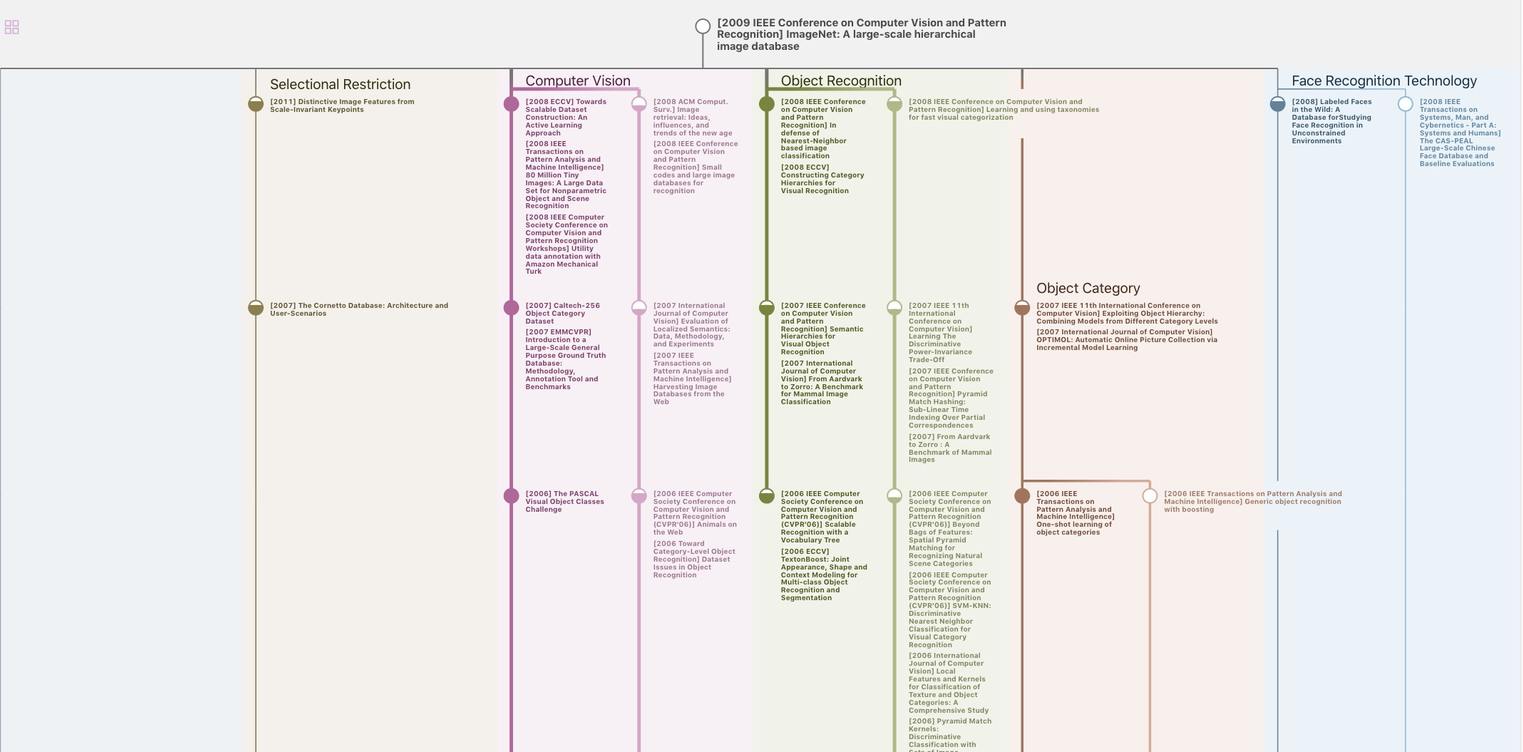
生成溯源树,研究论文发展脉络
Chat Paper
正在生成论文摘要