Uncertainty quantification of waterflooding in oil reservoirs computational simulations using a probabilistic learning approach
International Journal for Uncertainty Quantification(2023)
摘要
In the present paper, we propose an approach based on probabilistic learning for uncertainty quantification of the water-flooding processes in oil reservoir simulations, considering geological and economic uncertainties and multiple quanti-ties of interest (QoIs). We employ the probabilistic learning on manifolds (PLoM) method, which has achieved success in many different applications. This methodology enables the construction of surrogate models to cope with expensive computational costs using high-fidelity simulators. It also allows the incorporation of unavoidable uncertainties, like in the porosity and permeability fields, resulting from difficulties in the characterization of the heterogenous subsurface media, or arising from economic instabilities. We are particularly interested in computing high-order statistics of the system response, which combines oil operational production and economic aspects, to evaluate risk losses. In this pa-per, we assess the efficacy of the PLoM stochastic surrogate through two numerical examples contemplating the above uncertainties and typical reservoir configurations.
更多查看译文
关键词
uncertainty quantification,machine learning,risk analysis,porous media flow,reservoir engineering
AI 理解论文
溯源树
样例
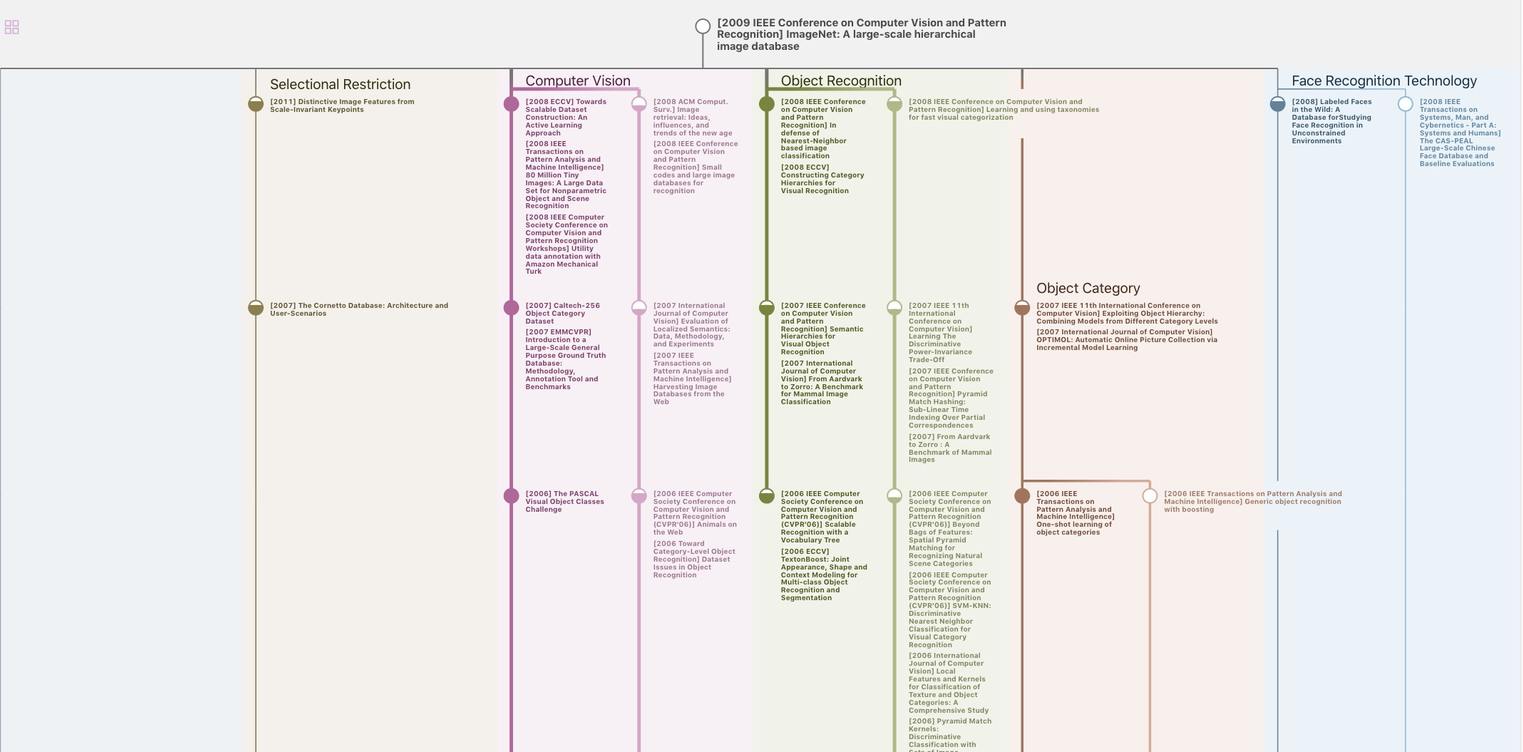
生成溯源树,研究论文发展脉络
Chat Paper
正在生成论文摘要