Prediction of Local PM2.5 Concentrations Based on Time-Series Feature Learning Using Multivariate LSTM
2022 22nd International Conference on Control, Automation and Systems (ICCAS)(2022)
摘要
Air pollution causes various health problems and diseases. Long-term PM 2.5 monitoring and prediction of its occurrence and sources are necessary not only in global areas based on public monitoring stations but also in local areas using cost-effective sensor systems. For this study, we developed a sensor system to achieve simplified and high-frequency PM 2.5 measurements. We attempted to learn and to predict local PM 2.5 concentrations from observed data using long short-term memory (LSTM) as a dominant time-series feature learning network. For improving learning and prediction accuracy evaluated according to the root mean square error (RMSE), sensor calibration is performed using a higher sensor. Moreover, we strove to reduce RMSE by optimizing its five major parameters. Experimentally obtained results demonstrate that the prediction accuracy is improved gradually after calibration and parameter optimization. As an ablation experiment, five meteorological factors are imported externally to verify the factors which contribute to reducing RMSE. Results verify the strong effects of local pressure and temperature for training and relative humidity and temperature for testing as validation.
更多查看译文
关键词
multivariate lstm,concentrations,prediction,time-series
AI 理解论文
溯源树
样例
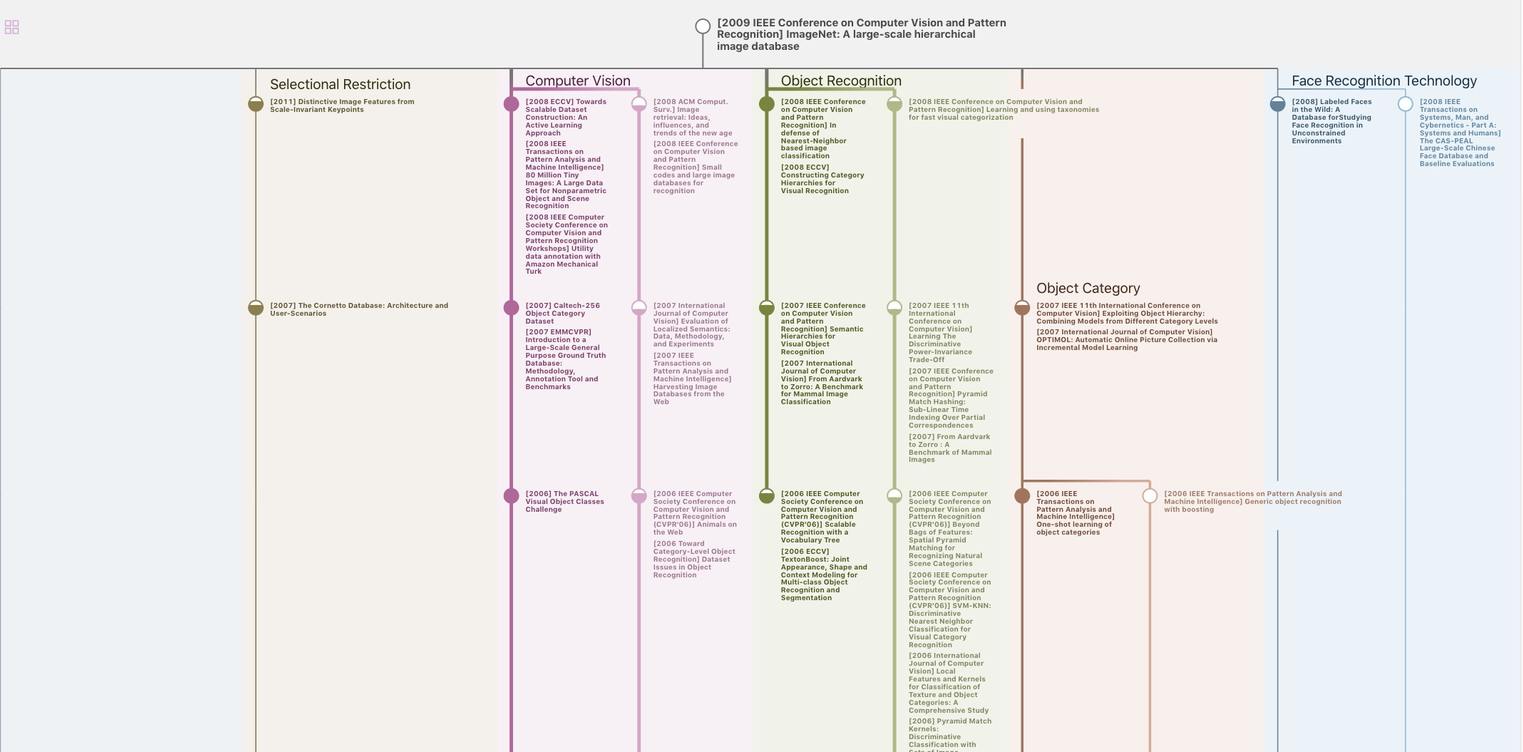
生成溯源树,研究论文发展脉络
Chat Paper
正在生成论文摘要