Predicting disease severity in multiple sclerosis using multimodal data and machine learning
Journal of Neurology(2024)
摘要
Background Multiple sclerosis patients would benefit from machine learning algorithms that integrates clinical, imaging and multimodal biomarkers to define the risk of disease activity. Methods We have analysed a prospective multi-centric cohort of 322 MS patients and 98 healthy controls from four MS centres, collecting disability scales at baseline and 2 years later. Imaging data included brain MRI and optical coherence tomography, and omics included genotyping, cytomics and phosphoproteomic data from peripheral blood mononuclear cells. Predictors of clinical outcomes were searched using Random Forest algorithms. Assessment of the algorithm performance was conducted in an independent prospective cohort of 271 MS patients from a single centre. Results We found algorithms for predicting confirmed disability accumulation for the different scales, no evidence of disease activity (NEDA), onset of immunotherapy and the escalation from low- to high-efficacy therapy with intermediate to high-accuracy. This accuracy was achieved for most of the predictors using clinical data alone or in combination with imaging data. Still, in some cases, the addition of omics data slightly increased algorithm performance. Accuracies were comparable in both cohorts. Conclusion Combining clinical, imaging and omics data with machine learning helps identify MS patients at risk of disability worsening.
更多查看译文
关键词
Multiple sclerosis,Omics,Imaging,Machine learning,Precision medicine
AI 理解论文
溯源树
样例
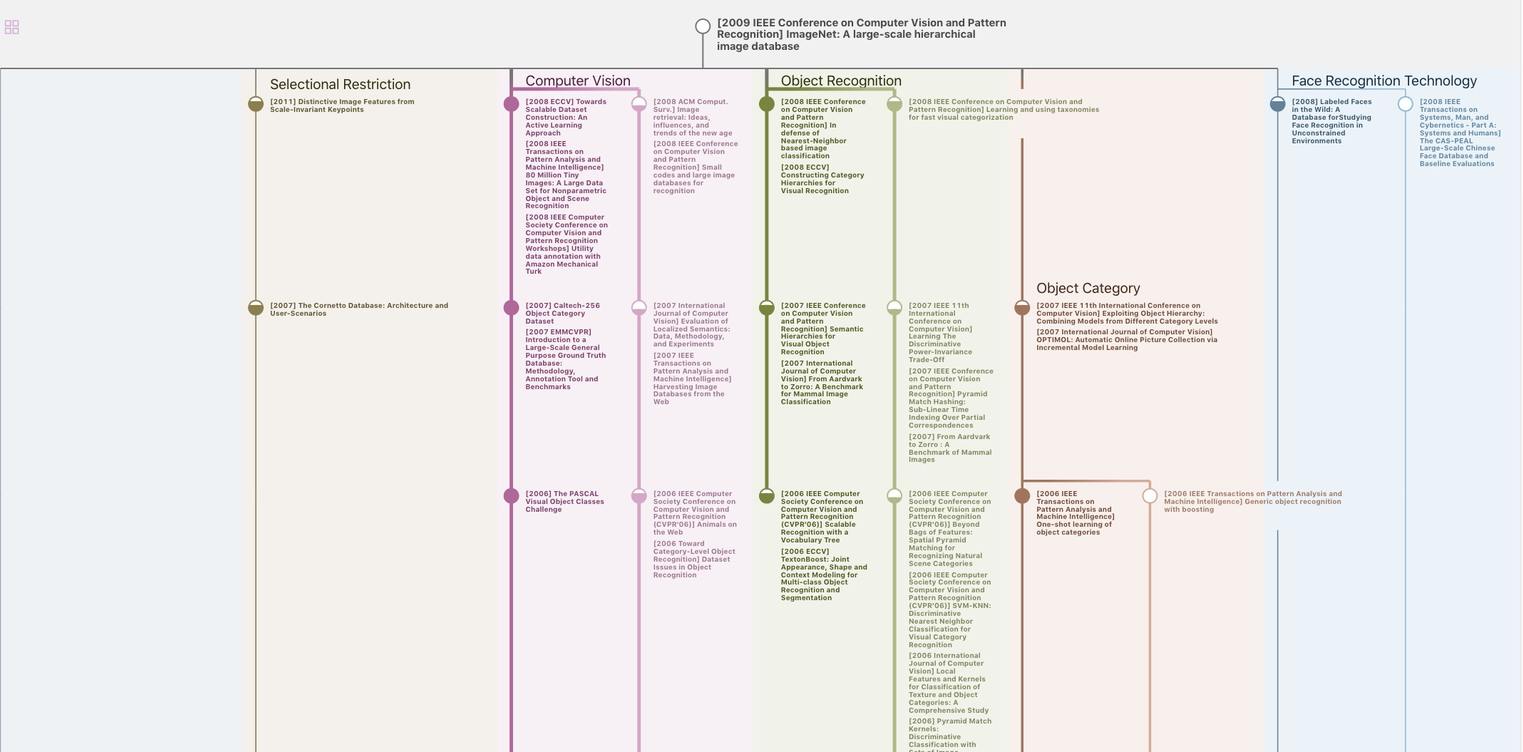
生成溯源树,研究论文发展脉络
Chat Paper
正在生成论文摘要