The use of Twitter data enhances LSTM - generated prediction of the spread of influenza-like-illness based on surveillance and weather reports (Preprint)
crossref(2022)
摘要
BACKGROUND The potential of harnessing the plurality of available data in real time along with advanced data analytics towards the accurate prediction of influenza-like-illness (ILI) outbreaks has gained significant scientific interest. Different methodologies based on the use of machine learning techniques and traditional and alternative data sources such as ILI surveillance reports, weather reports, search engine queries, and social media, have been explored with the ultimate goal to be utilized in the development of electronic surveillance systems that could complement existing monitoring resources. OBJECTIVE The aim of the present study is to investigate for the first time the combined use of ILI surveillance data, weather data, and Twitter data, along with deep learning techniques towards the development of prediction models able to nowcast and forecast weekly ILI cases. METHODS The model’s input space consists of information related to weekly ILI surveillance, online social (e.g., Twitter) behavior, and weather conditions. For the design and development of the model, relevant data corresponding to the period 2010-2019 and focusing on the Greek population and weather have been collected. Long short term memory neural networks (LSTMs) are leveraged to efficiently handle the sequential and nonlinear nature of the multitude of collected data. The three data categories are firstly utilized separately for training three LSTM-based primary models. Subsequently, different transfer learning (TL) approaches are explored with the aim of creating various feature spaces combining the features extracted from the corresponding primary models’ LSTM layers in order for the latter to feed a dense layer. RESULTS The primary model which learns from weather data yields better forecast accuracy (root mean square error - RMSE = 0.144, pearson correlation coefficient - PCC= 0.801) than the model which is trained with ILI historical data (RMSE = 0.159, PCC= 0.794). The best performance is achieved by the TL-based model leveraging the combination of the three data categories (RMSE = 0.128, PCC = 0.822). CONCLUSIONS The superiority of the TL-based model which takes into account Twitter data, weather data, and ILI surveillance data reflects the potential of alternative public sources to enhance accurate and reliable prediction of the ILI spread.
更多查看译文
AI 理解论文
溯源树
样例
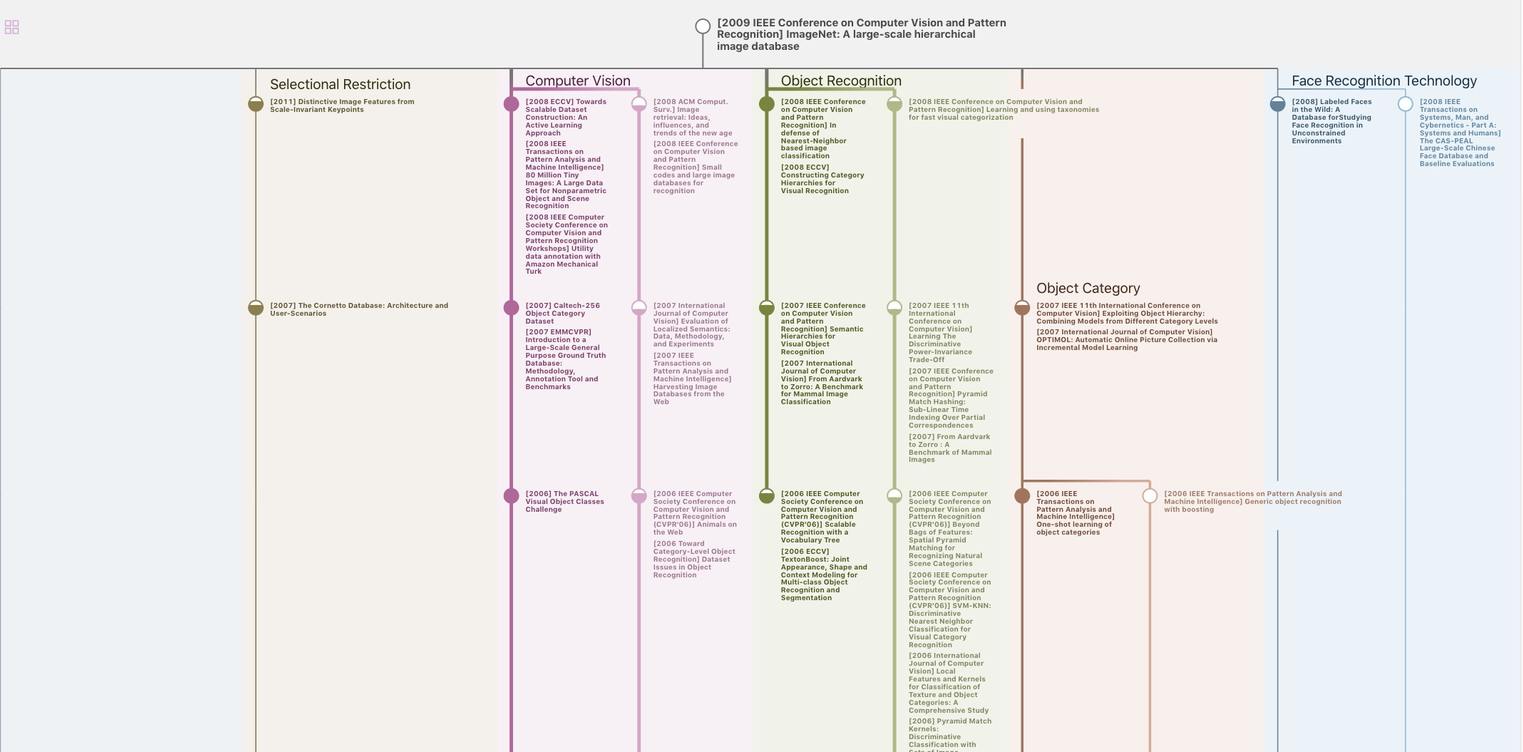
生成溯源树,研究论文发展脉络
Chat Paper
正在生成论文摘要