Wetland Mapping integrating Sentinel-1 and Sentine-2 data: a Multilevel Global-to-Local Feature Optimization Wetlands Hierarchical Classification Approach
Authorea (Authorea)(2022)
摘要
In this article, Sentinel-1 and Sentine-2 data were integrated to mapping wetlands in Yellow River Delta. Given that different wetlands may owning different optimal feature subsets, we propose a multilevel global-to-local feature optimization wetlands hierarchical classification approach. This method constructs classification trees according to the classification difficulty of wetlands and implements wetland classification in three layers. During the process of hierarchical classification, we utilized the effectiveness of random forest algorithm in feature evaluation to select the most suitable feature subset for each classification node of the classification tree for training classifiers and implementing classification. Specifically, this paper uses Stacking method to construct classifiers. Seven sets of comparison experiments were designed to verify the effects of the proposed multilevel global-to-local feature optimization wetlands hierarchical classification approach. Using the proposed method, the overall accuracy and kappa statistic are improved to 90.06% and 0.8768 compared with other classification approach.
更多查看译文
关键词
wetland mapping,classification,global-to-local
AI 理解论文
溯源树
样例
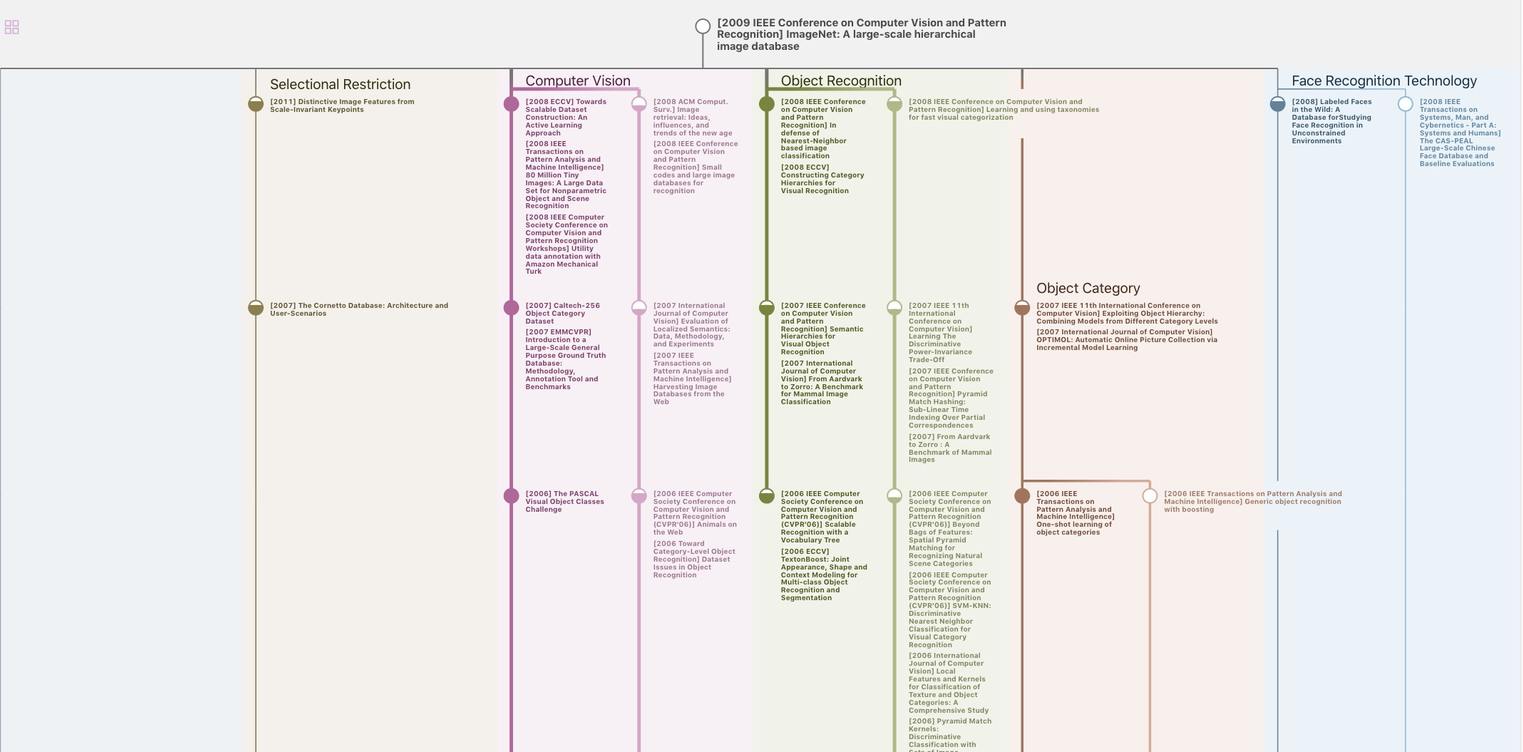
生成溯源树,研究论文发展脉络
Chat Paper
正在生成论文摘要