A Significant Feature Selection to Improve the Accuracy of a Classification Algorithm for Steel Defect
2022 4th International Conference on Inventive Research in Computing Applications (ICIRCA)(2022)
摘要
Buildings and a lot of other such construction elements include steel as the main part. The quality of the steel is often overlooked. This may lead to fatal consequences when prolonged for a long time. To solve this issue, this study aims to find the best combination of feature extraction and classification technique. For this procedure, a dataset consisting of various types of defects that can occur in steel is collected. This dataset will be used to test and train the models that consist of both feature extraction and classification algorithms. The Gray-Level Co-occurrence Matrix (GLCM) and the Gray-Level Run Length Matrix (GLRM) are two extraction approaches employed in this procedure for clear data extraction from the brain picture. The extracted data is then examined using four Machine Learning (ML) classifiers: SVM or the Support Vector Machine (SVM), the K-Nearest Neighbor (KNN), and the Random Forest (RF). The data are then evaluated further using a confusion matrix to determine a technique with more precision and accuracy. The confusion matrix employs the TPR/FPR - True and False Positive Rate - and TNR/FNR - True and False Negative Rate - concepts. The values are then compared in the form of a bar graph and the combination has higher accuracy and other values. The result of the comparison provides us with the best combination of feature extraction techniques and classifier algorithms that can be used to detect and classify the type of defect from the steel plates and pipes.
更多查看译文
关键词
significant feature selection,classification algorithm
AI 理解论文
溯源树
样例
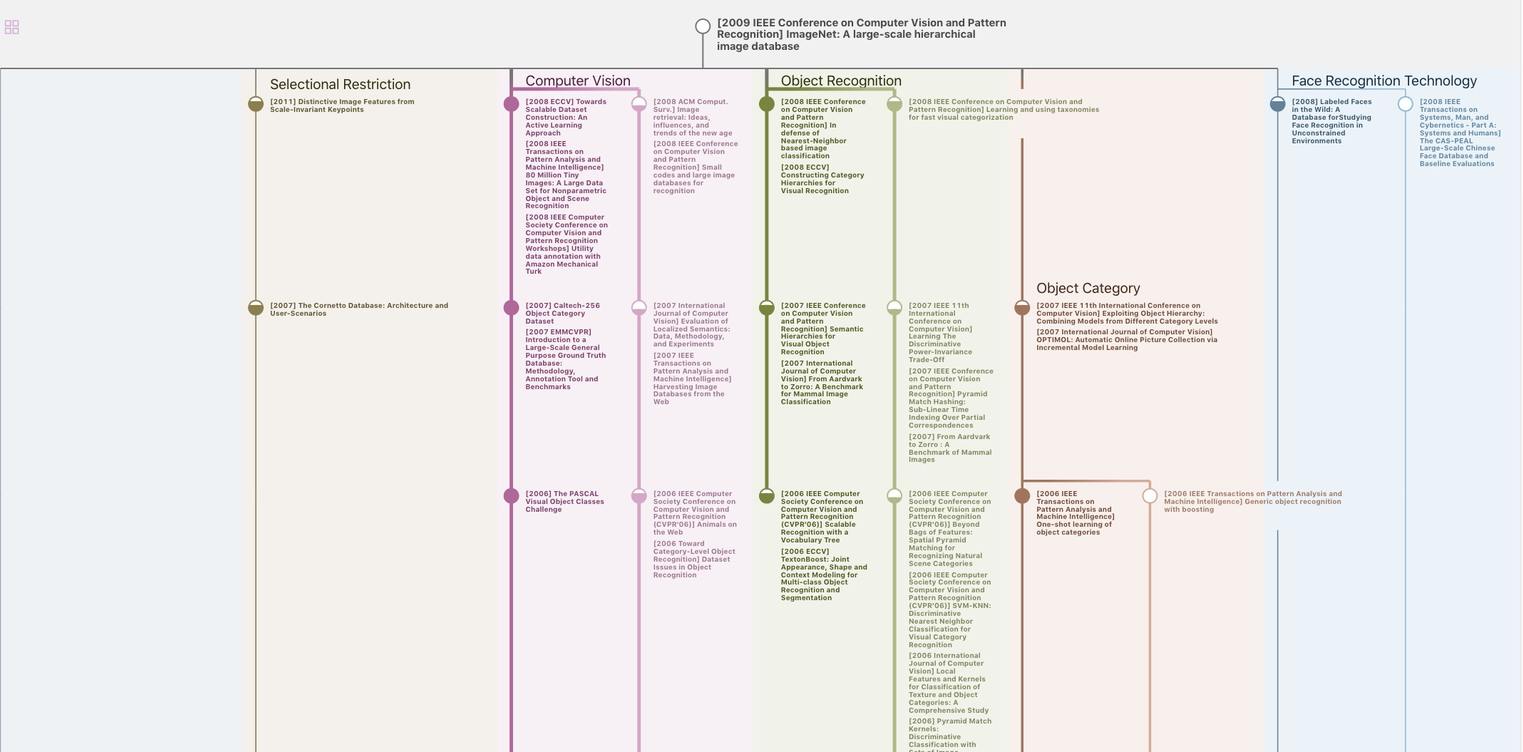
生成溯源树,研究论文发展脉络
Chat Paper
正在生成论文摘要