Relating enhancer genetic variation across mammals to complex phenotypes using machine learning
bioRxiv (Cold Spring Harbor Laboratory)(2022)
摘要
AbstractProtein-coding differences between mammals often fail to explain phenotypic diversity, suggesting involvement of enhancers, often rapidly evolving regions that regulate gene expression. Identifying associations between enhancers and phenotypes is challenging because enhancer activity is context-dependent and may be conserved without much sequence conservation. We developed TACIT (Tissue-Aware Conservation Inference Toolkit) to associate open chromatin regions (OCRs) with phenotypes using predictions in hundreds of mammalian genomes from machine learning models trained to learn tissue-specific regulatory codes. Applying TACIT for motor cortex and parvalbumin-positive interneurons to neurological phenotypes revealed dozens of new OCR-phenotype associations. Many associated OCRs were near relevant genes, including brain size-associated OCRs near genes mutated in microcephaly or macrocephaly. Our work creates a forward genomics foundation for identifying candidate enhancers associated with phenotype evolution.One Sentence SummaryA new machine learning-based approach associates enhancers with the evolution of brain size and behavior across mammals.
更多查看译文
关键词
enhancer,complex phenotypes,genetic variation,machine learning,mammals
AI 理解论文
溯源树
样例
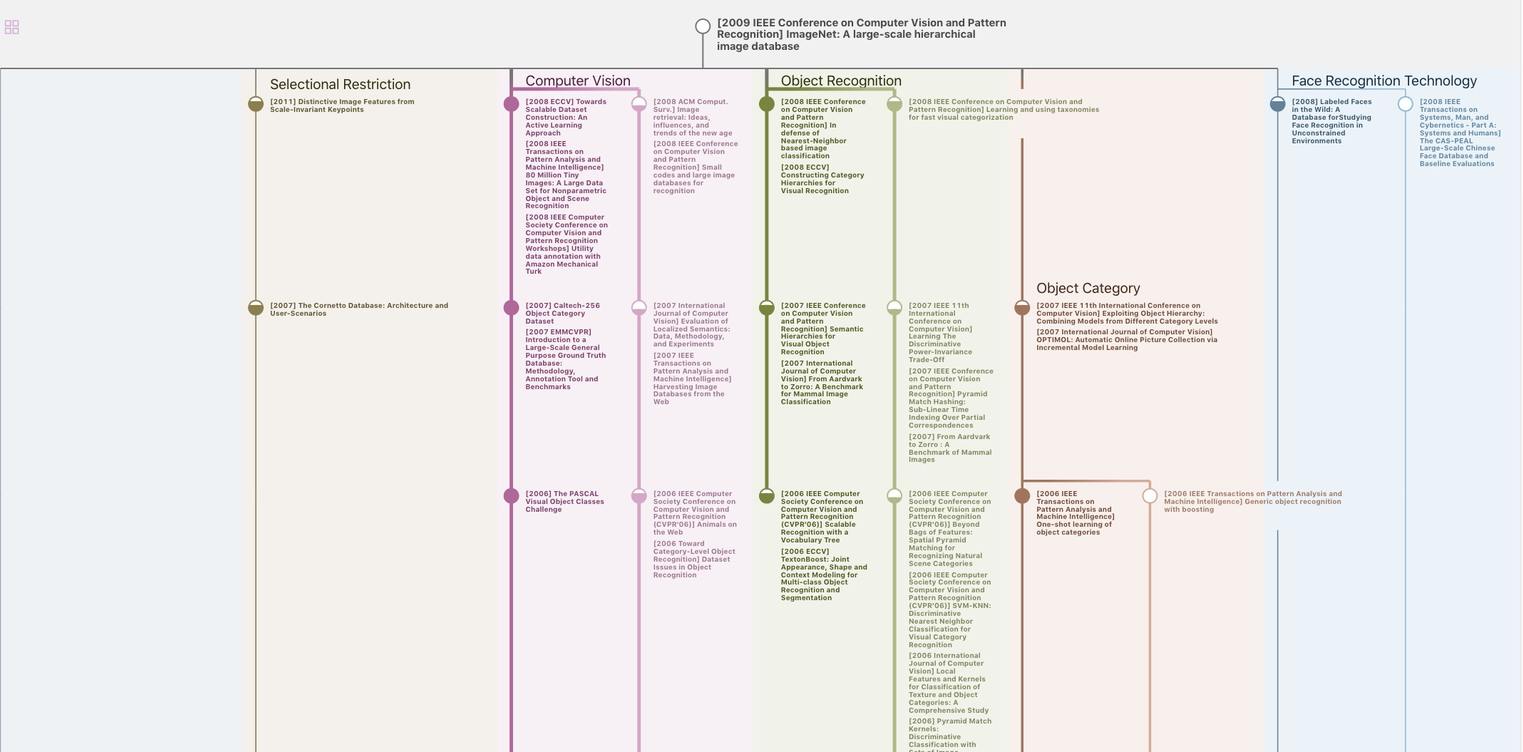
生成溯源树,研究论文发展脉络
Chat Paper
正在生成论文摘要