A Borehole Porosity Prediction Method with Focusing on Local Shape
Social Science Research Network(2022)
摘要
Porosity is a valuable parameter reflecting petroleum storage performance and plays an important role in reservoir exploration. Various studies have confirmed the feasibility of establishing a mapping between geophysical logging and porosity by using machine learning methods. The existing works are generally in the form of depth point-to-point. However, due to the temporal and spatial characteristics of the stratum deposition, the correlation under continuous depths of logging curves should be considered in addition to the absolute values. Attempts have been made to achieve the memory for low-frequency information with continuous depths by introducing the concept of time in recurrent neural networks. This logging porosity prediction strategy has disadvantages due to two reasons: (i) periodicity is present in the logging data, but is weak, and (ii) small-scale information of the logging curves have a guiding meaning on the detail of porosity. In this paper, we present the first attempt to describe the high-frequency variation in the local shape of the logging curves as spatial small-scale features and propose a ConvLSTM-based framework in the porosity prediction task. Specifically, the local shape features of the curves are further extracted by convolutional operations as the contextual information is memorized. We form 1-dimensional (1D) images in a segment-to-point manner (fixing segment to 16 depth points) to make the logging data suitable for convolution operations while increasing the volume of data. Further, segment-to-point samples are automatically intercepted and feed into model with segment-to-segment prediction patterns according to the view window size. Various experiments are carried out on a real dataset from the Shengli Oilfield, Jiyang Depression, and the results validate the effectiveness of ConvLSTM for local shape information extraction and the superiority in porosity prediction.
更多查看译文
关键词
Porosity prediction,Well Logging,ConvLSTM,CNN-LSTM,LSTM,CNN
AI 理解论文
溯源树
样例
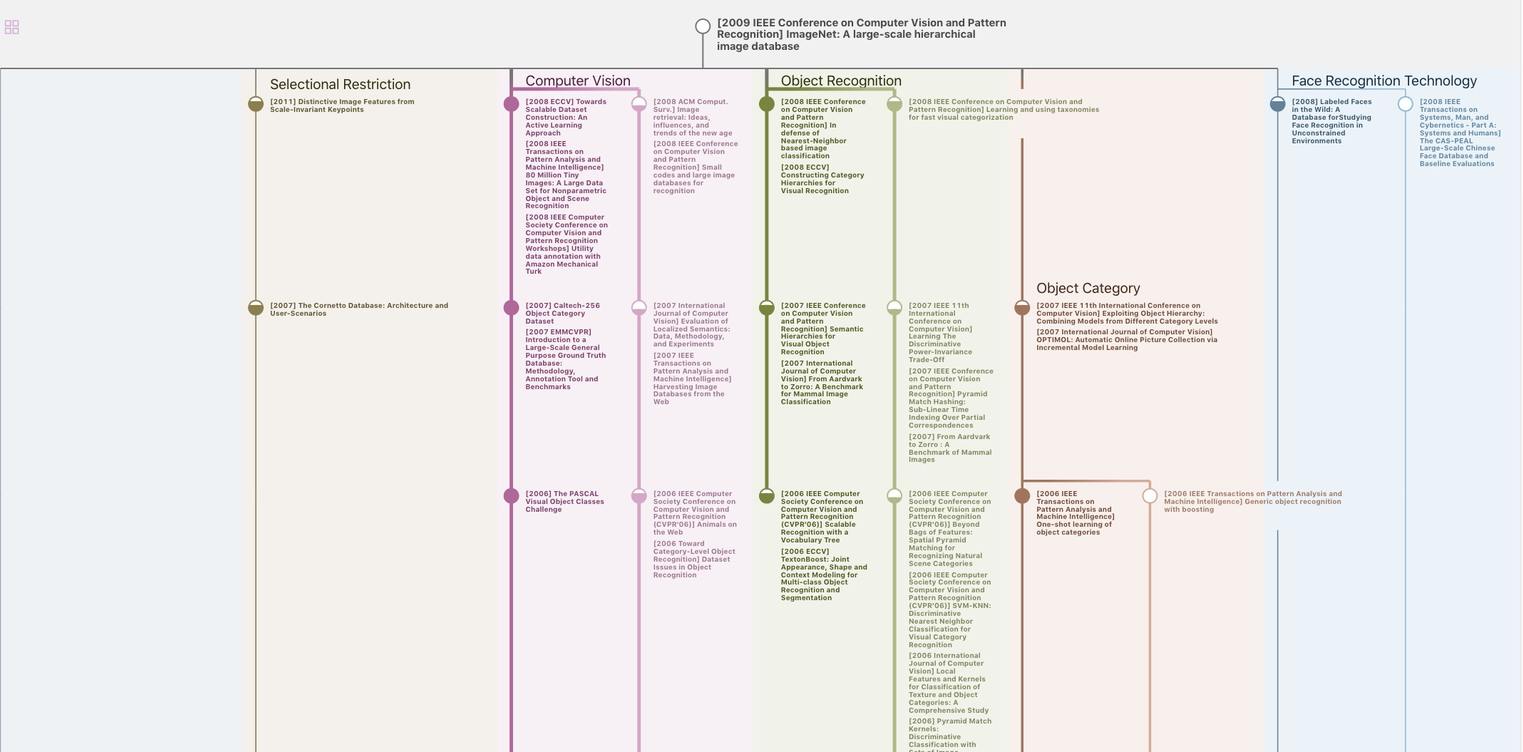
生成溯源树,研究论文发展脉络
Chat Paper
正在生成论文摘要