Spatial depth for data in metric spaces
arxiv(2023)
摘要
We propose a novel measure of statistical depth, the metric spatial depth, for data residing in an arbitrary metric space. The measure assigns high (low) values for points located near (far away from) the bulk of the data distribution, allowing quantifying their centrality/outlyingness. This depth measure is shown to have highly interpretable geometric properties, making it appealing in object data analysis where standard descriptive statistics are difficult to compute. The proposed measure reduces to the classical spatial depth in a Euclidean space. In addition to studying its theoretical properties, to provide intuition on the concept, we explicitly compute metric spatial depths in several different metric spaces. Finally, we showcase the practical usefulness of the metric spatial depth in outlier detection, non-convex depth region estimation and classification.
更多查看译文
关键词
spatial depth,metric spaces,data
AI 理解论文
溯源树
样例
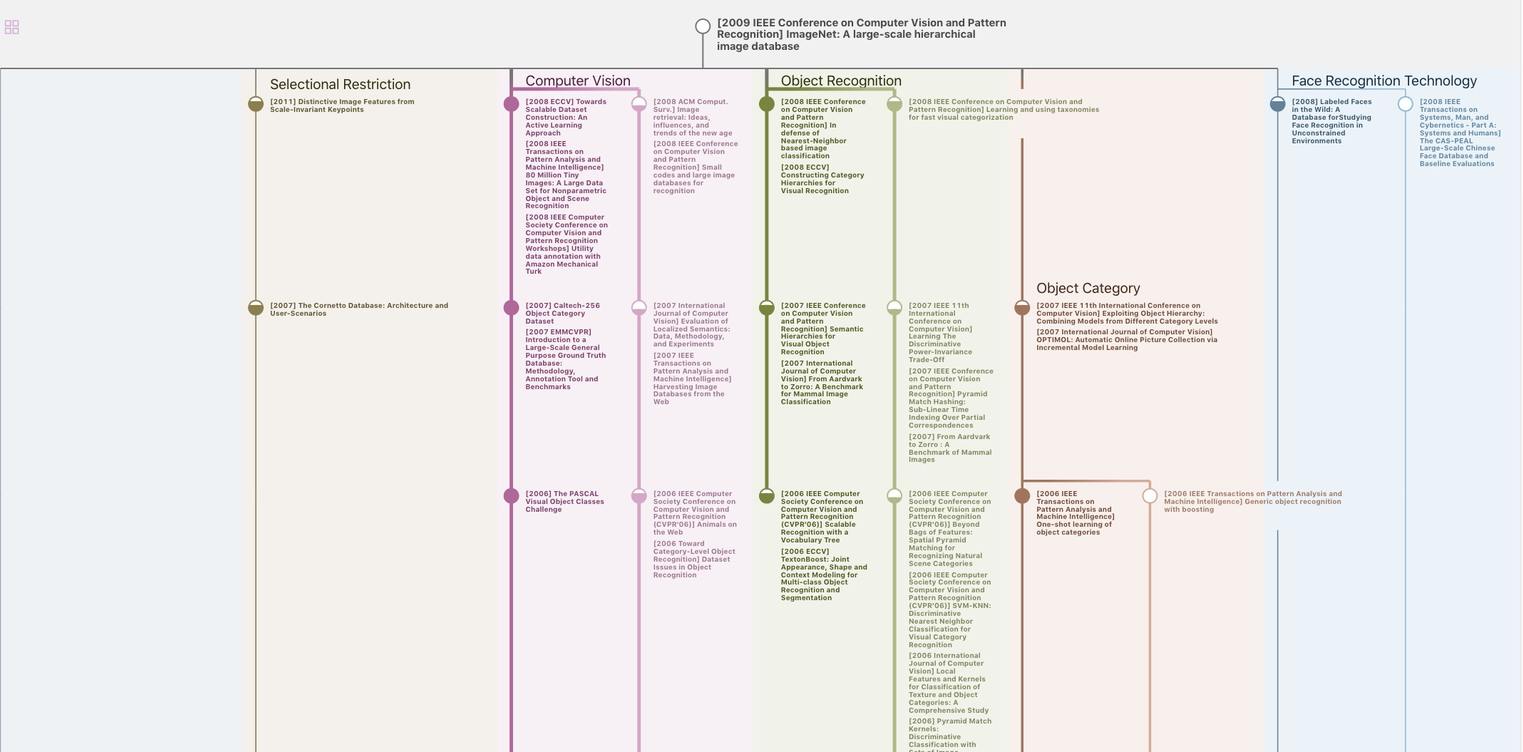
生成溯源树,研究论文发展脉络
Chat Paper
正在生成论文摘要