Actor-Critic Model Predictive Control
CoRR(2023)
Abstract
Despite its success, Model Predictive Control (MPC) often requires intensive task-specific engineering and tuning. On the other hand, Reinforcement Learning (RL) architectures minimize this effort, but need extensive data collection and lack interpretability and safety. An open research question is how to combine the advantages of RL and MPC to exploit the best of both worlds. This paper introduces a novel modular RL architecture that bridges these two approaches. By placing a differentiable MPC in the heart of an actor-critic RL agent, the proposed system enables short-term predictions and optimization of actions based on system dynamics, while retaining the end-to-end training benefits and exploratory behavior of an RL agent. The proposed approach effectively handles two different time-horizon scales: short-term decisions managed by the actor MPC and long term ones managed by the critic network. This provides a promising direction for RL, which combines the advantages of model-based and end-to-end learning methods. We validate the approach in simulated and real-world experiments on a quadcopter platform performing different high-level tasks, and show that the proposed method can learn complex behaviours end-to-end while retaining the properties of an MPC.
MoreTranslated text
AI Read Science
Must-Reading Tree
Example
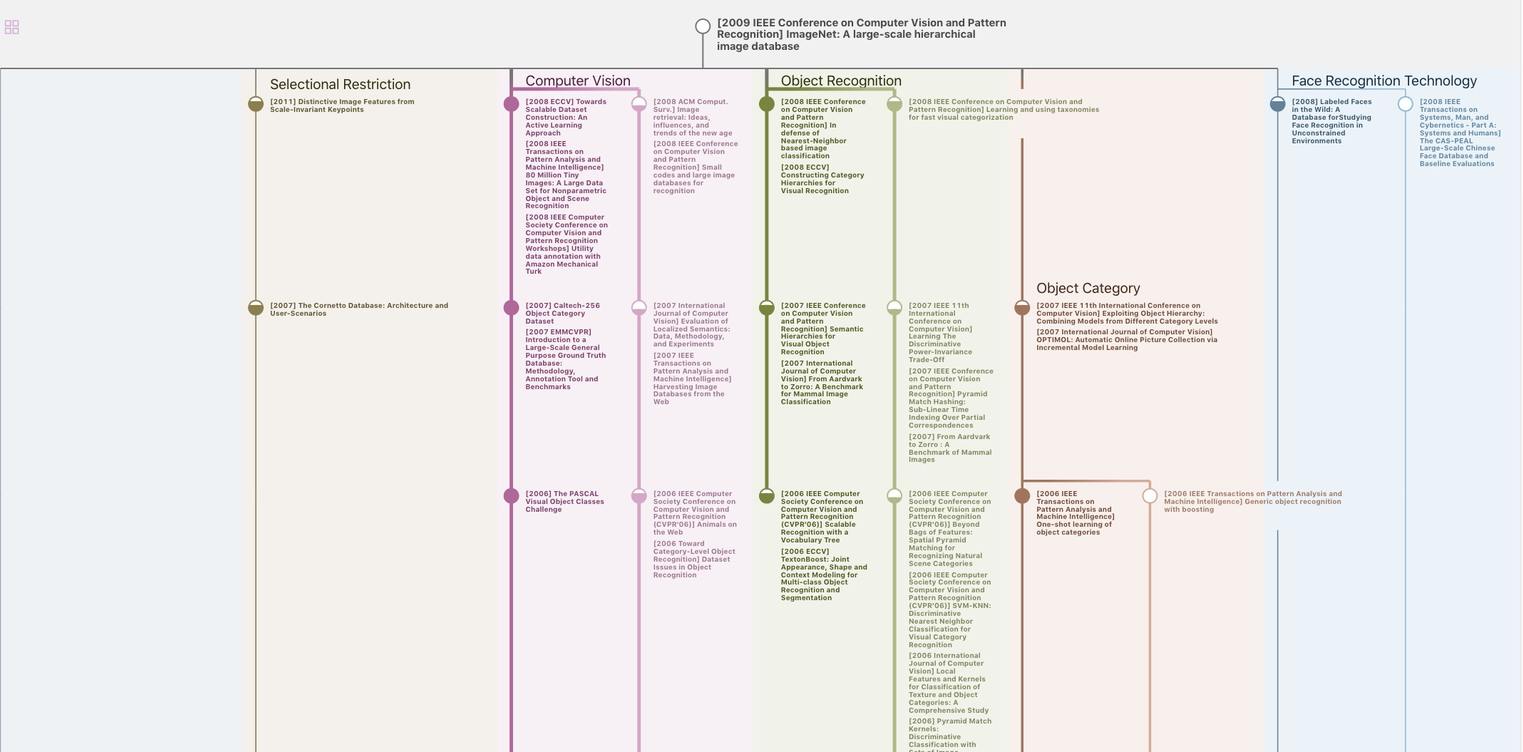
Generate MRT to find the research sequence of this paper
Chat Paper
Summary is being generated by the instructions you defined