Online Map Vectorization for Autonomous Driving: A Rasterization Perspective
arXiv (Cornell University)(2023)
摘要
Vectorized high-definition (HD) map is essential for autonomous driving, providing detailed and precise environmental information for advanced perception and planning. However, current map vectorization methods often exhibit deviations, and the existing evaluation metric for map vectorization lacks sufficient sensitivity to detect these deviations. To address these limitations, we propose integrating the philosophy of rasterization into map vectorization. Specifically, we introduce a new rasterization-based evaluation metric, which has superior sensitivity and is better suited to real-world autonomous driving scenarios. Furthermore, we propose MapVR (Map Vectorization via Rasterization), a novel framework that applies differentiable rasterization to vectorized outputs and then performs precise and geometry-aware supervision on rasterized HD maps. Notably, MapVR designs tailored rasterization strategies for various geometric shapes, enabling effective adaptation to a wide range of map elements. Experiments show that incorporating rasterization into map vectorization greatly enhances performance with no extra computational cost during inference, leading to more accurate map perception and ultimately promoting safer autonomous driving.
更多查看译文
关键词
map,autonomous driving,rasterization
AI 理解论文
溯源树
样例
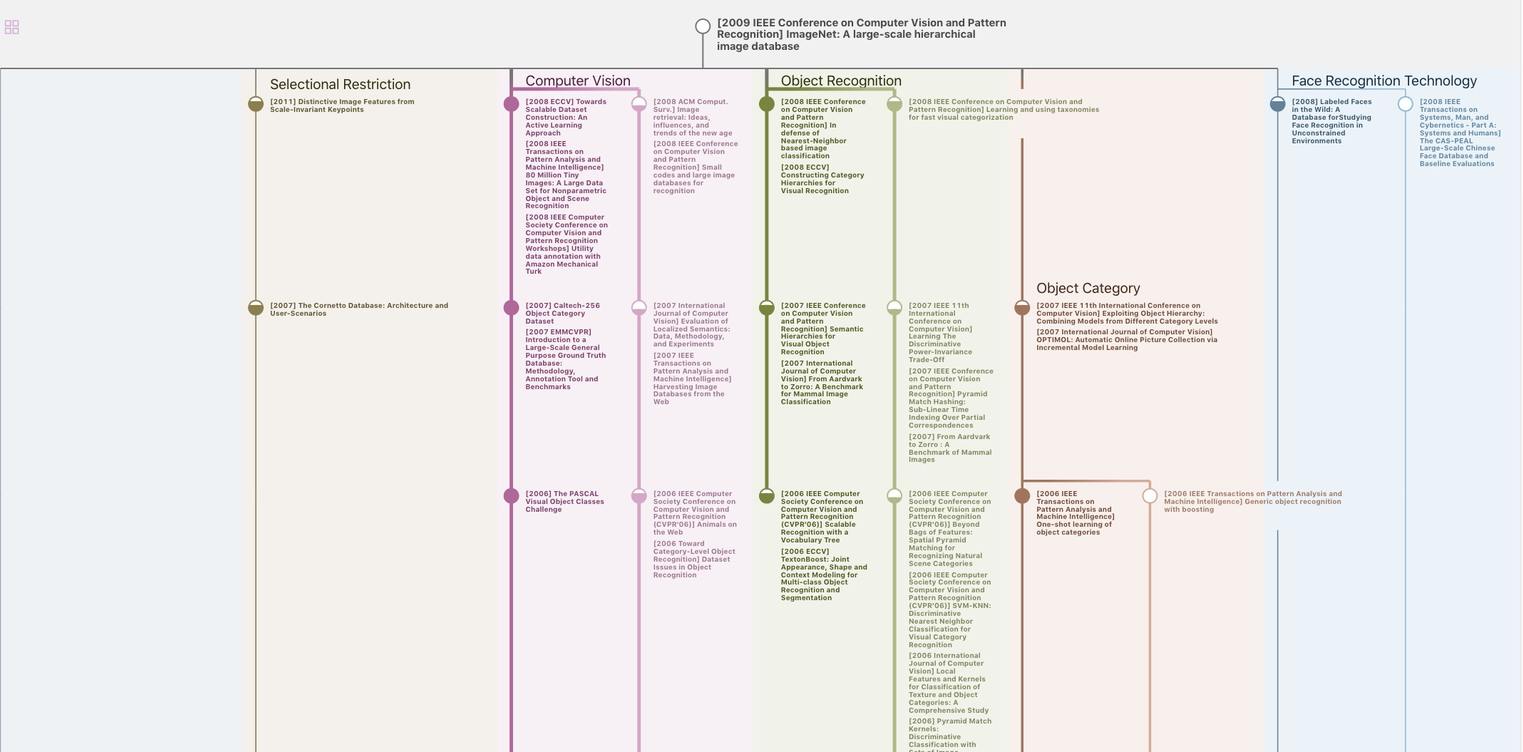
生成溯源树,研究论文发展脉络
Chat Paper
正在生成论文摘要