Renderers are Good Zero-Shot Representation Learners: Exploring Diffusion Latents for Metric Learning
CoRR(2023)
摘要
Can the latent spaces of modern generative neural rendering models serve as representations for 3D-aware discriminative visual understanding tasks? We use retrieval as a proxy for measuring the metric learning properties of the latent spaces of Shap-E, including capturing view-independence and enabling the aggregation of scene representations from the representations of individual image views, and find that Shap-E representations outperform those of the classical EfficientNet baseline representations zero-shot, and is still competitive when both methods are trained using a contrative loss. These findings give preliminary indication that 3D-based rendering and generative models can yield useful representations for discriminative tasks in our innately 3D-native world. Our code is available at \url{https://github.com/michaelwilliamtang/golden-retriever}.
更多查看译文
AI 理解论文
溯源树
样例
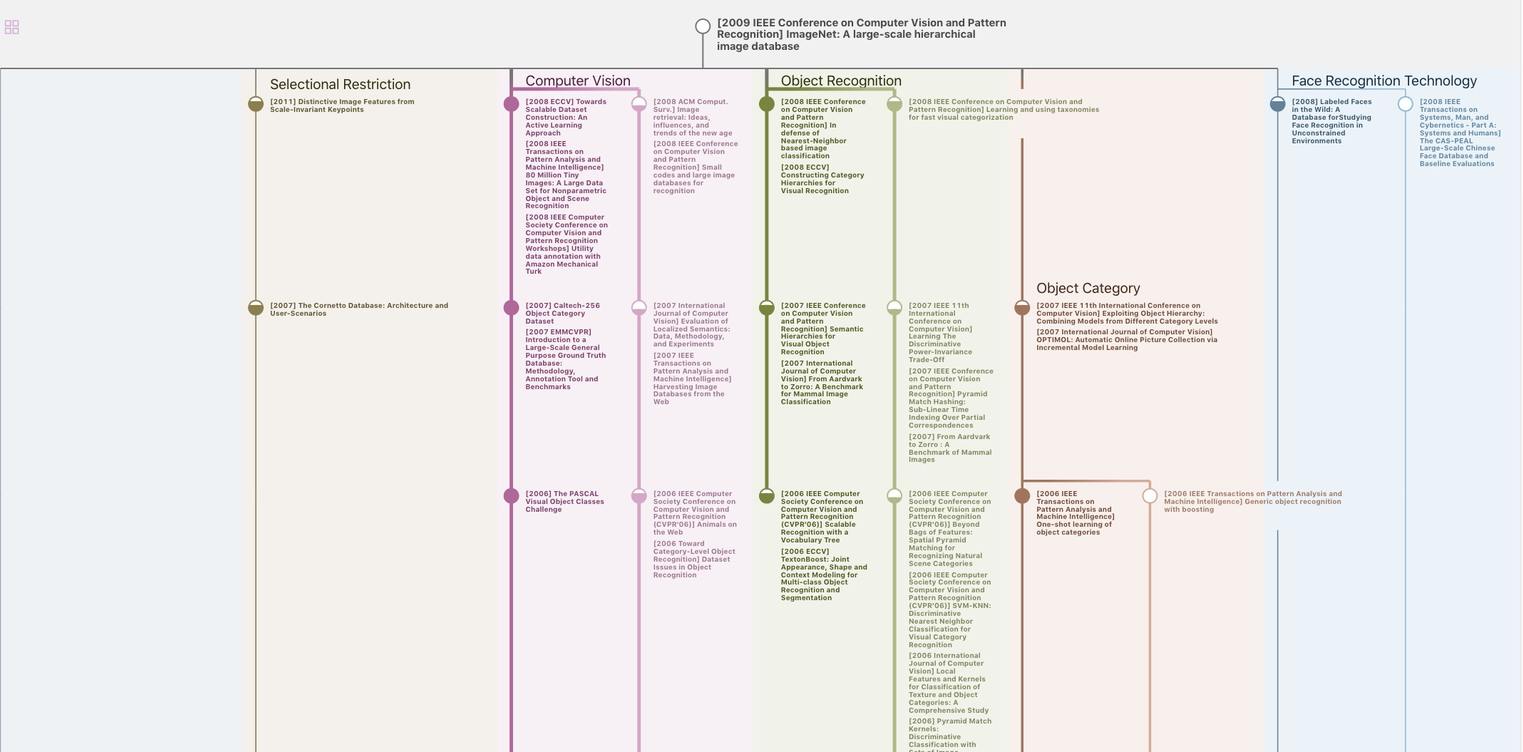
生成溯源树,研究论文发展脉络
Chat Paper
正在生成论文摘要