Partial Hypernetworks for Continual Learning
CoRR(2023)
摘要
Hypernetworks mitigate forgetting in continual learning (CL) by generating task-dependent weights and penalizing weight changes at a meta-model level. Unfortunately, generating all weights is not only computationally expensive for larger architectures, but also, it is not well understood whether generating all model weights is necessary. Inspired by latent replay methods in CL, we propose partial weight generation for the final layers of a model using hypernetworks while freezing the initial layers. With this objective, we first answer the question of how many layers can be frozen without compromising the final performance. Through several experiments, we empirically show that the number of layers that can be frozen is proportional to the distributional similarity in the CL stream. Then, to demonstrate the effectiveness of hypernetworks, we show that noisy streams can significantly impact the performance of latent replay methods, leading to increased forgetting when features from noisy experiences are replayed with old samples. In contrast, partial hypernetworks are more robust to noise by maintaining accuracy on previous experiences. Finally, we conduct experiments on the split CIFAR-100 and TinyImagenet benchmarks and compare different versions of partial hypernetworks to latent replay methods. We conclude that partial weight generation using hypernetworks is a promising solution to the problem of forgetting in neural networks. It can provide an effective balance between computation and final test accuracy in CL streams.
更多查看译文
关键词
learning
AI 理解论文
溯源树
样例
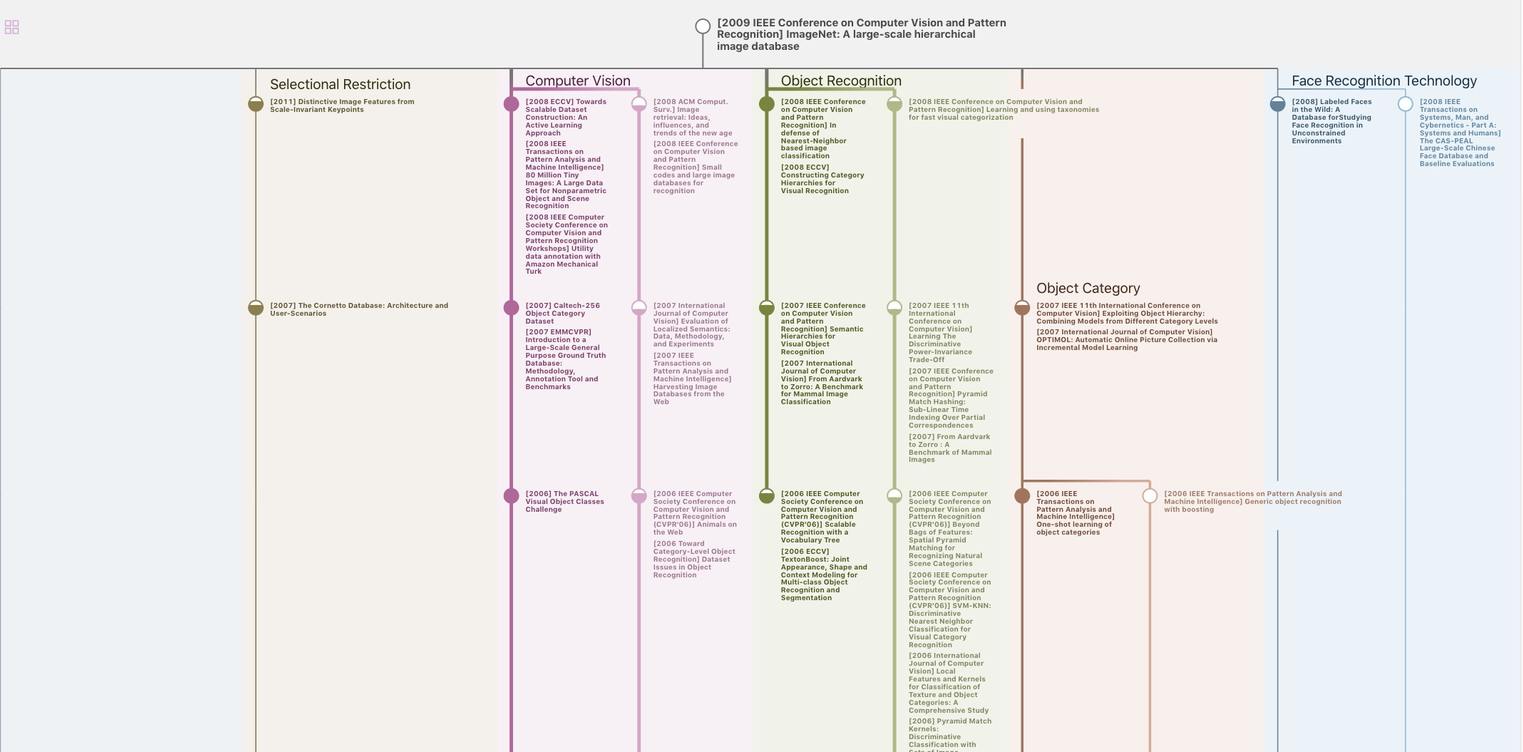
生成溯源树,研究论文发展脉络
Chat Paper
正在生成论文摘要