Efficient Large-scale Nonstationary Spatial Covariance Function Estimation Using Convolutional Neural Networks.
CoRR(2023)
摘要
Spatial processes observed in various fields, such as climate and environmental science, often occur on a large scale and demonstrate spatial nonstationarity. Fitting a Gaussian process with a nonstationary Matérn covariance is challenging. Previous studies in the literature have tackled this challenge by employing spatial partitioning techniques to estimate the parameters that vary spatially in the covariance function. The selection of partitions is an important consideration, but it is often subjective and lacks a data-driven approach. To address this issue, in this study, we utilize the power of Convolutional Neural Networks (ConvNets) to derive subregions from the nonstationary data. We employ a selection mechanism to identify subregions that exhibit similar behavior to stationary fields. In order to distinguish between stationary and nonstationary random fields, we conducted training on ConvNet using various simulated data. These simulations are generated from Gaussian processes with Matérn covariance models under a wide range of parameter settings, ensuring adequate representation of both stationary and nonstationary spatial data. We assess the performance of the proposed method with synthetic and real datasets at a large scale. The results revealed enhanced accuracy in parameter estimations when relying on ConvNet-based partition compared to traditional user-defined approaches.
更多查看译文
关键词
Forecasting,Variable Selection,Multivariate Calibration
AI 理解论文
溯源树
样例
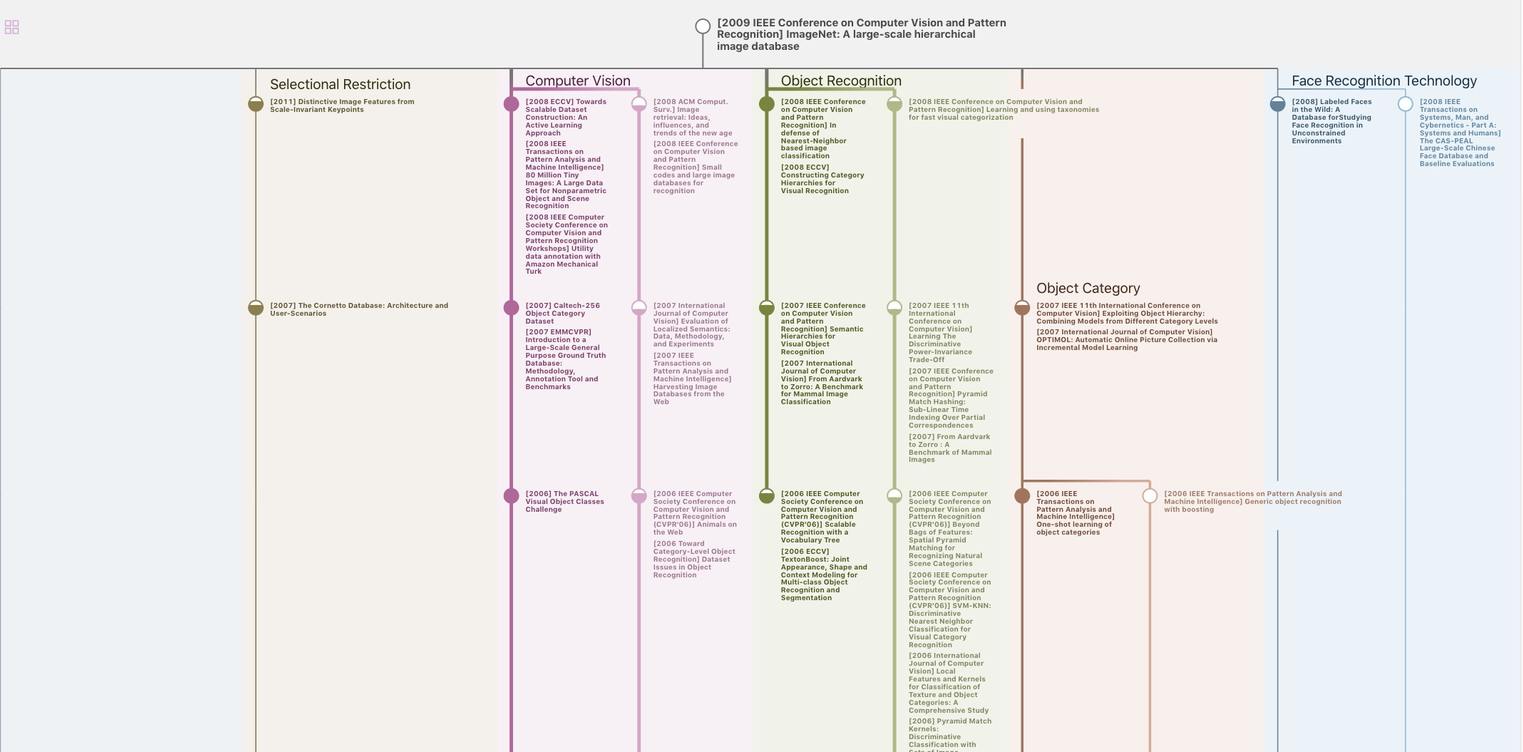
生成溯源树,研究论文发展脉络
Chat Paper
正在生成论文摘要