Computing a human-like reaction time metric from stable recurrent vision models
arXiv (Cornell University)(2023)
摘要
The meteoric rise in the adoption of deep neural networks as computational models of vision has inspired efforts to "align" these models with humans. One dimension of interest for alignment includes behavioral choices, but moving beyond characterizing choice patterns to capturing temporal aspects of visual decision-making has been challenging. Here, we sketch a general-purpose methodology to construct computational accounts of reaction times from a stimulus-computable, task-optimized model. Specifically, we introduce a novel metric leveraging insights from subjective logic theory summarizing evidence accumulation in recurrent vision models. We demonstrate that our metric aligns with patterns of human reaction times for stimulus manipulations across four disparate visual decision-making tasks spanning perceptual grouping, mental simulation, and scene categorization. This work paves the way for exploring the temporal alignment of model and human visual strategies in the context of various other cognitive tasks toward generating testable hypotheses for neuroscience.
更多查看译文
关键词
stable recurrent vision,models,human-like
AI 理解论文
溯源树
样例
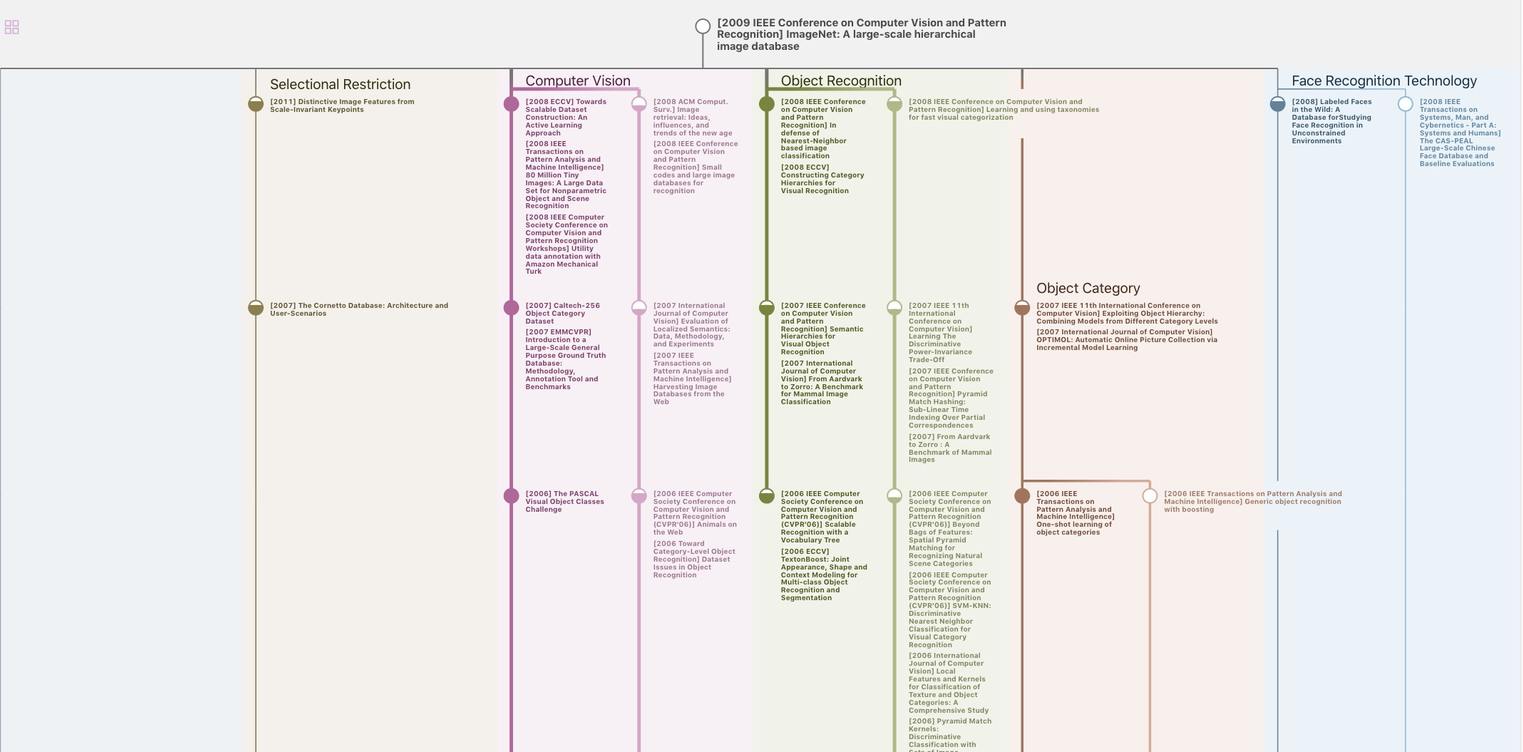
生成溯源树,研究论文发展脉络
Chat Paper
正在生成论文摘要