BEVScope: Enhancing Self-Supervised Depth Estimation Leveraging Bird's-Eye-View in Dynamic Scenarios
CoRR(2023)
Abstract
Depth estimation is a cornerstone of perception in autonomous driving and robotic systems. The considerable cost and relatively sparse data acquisition of LiDAR systems have led to the exploration of cost-effective alternatives, notably, self-supervised depth estimation. Nevertheless, current self-supervised depth estimation methods grapple with several limitations: (1) the failure to adequately leverage informative multi-camera views. (2) the limited capacity to handle dynamic objects effectively. To address these challenges, we present BEVScope, an innovative approach to self-supervised depth estimation that harnesses Bird's-Eye-View (BEV) features. Concurrently, we propose an adaptive loss function, specifically designed to mitigate the complexities associated with moving objects. Empirical evaluations conducted on the Nuscenes dataset validate our approach, demonstrating competitive performance. Code will be released at https://github.com/myc634/BEVScope.
MoreTranslated text
Key words
depth estimation,self-supervised,s-eye-view
AI Read Science
Must-Reading Tree
Example
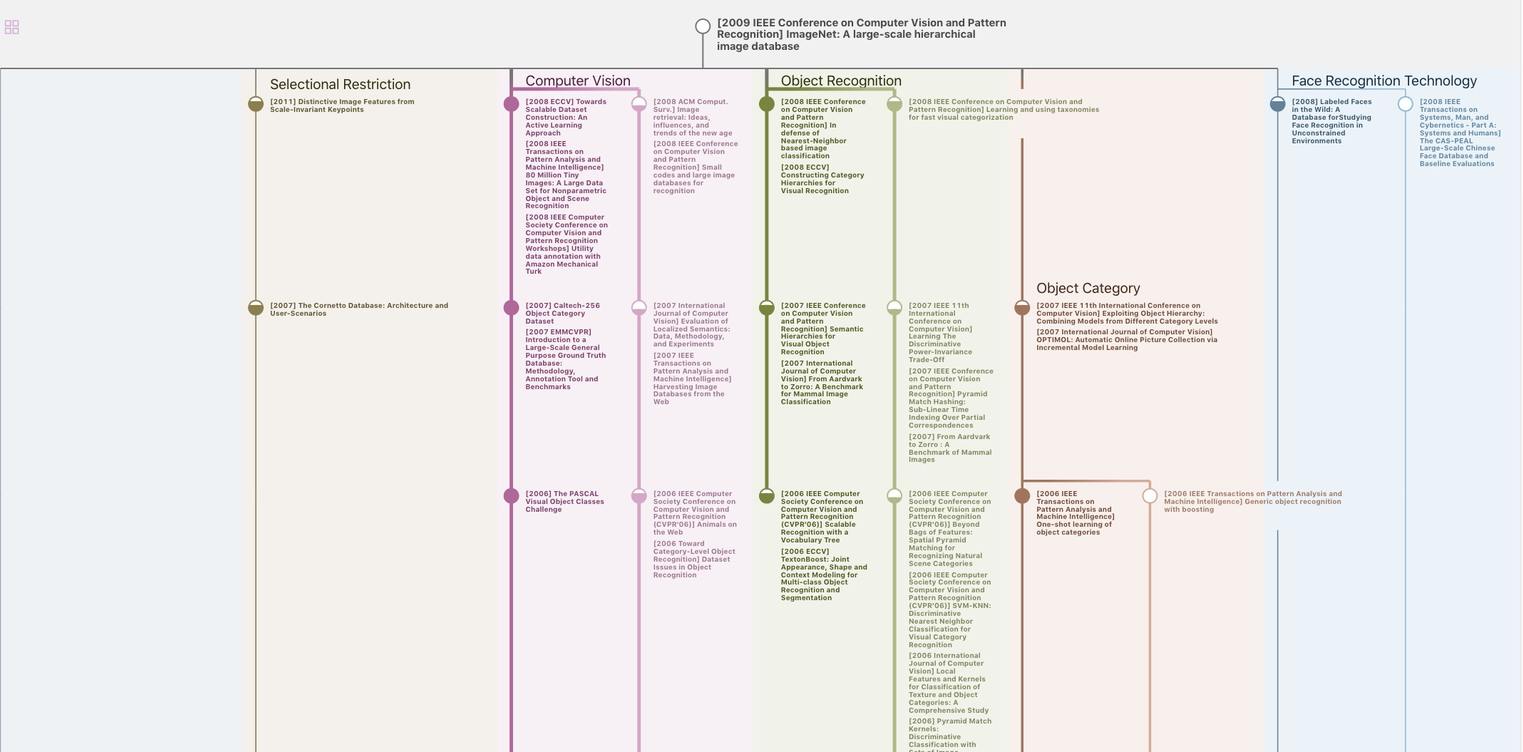
Generate MRT to find the research sequence of this paper
Chat Paper
Summary is being generated by the instructions you defined