Indoor Environment Learning via RF-Mapping
IEEE Journal on Selected Areas in Communications(2023)
摘要
Intelligent integrated sensing and communication is one of key aspects of future wireless networks in which sensing can be leveraged to enhance communications and vice-versa. In this paper, we propose a novel sensing solution that can be used to represent an RF-environment. The proposed solution accounts for practical challenges such as limited time resolution due to limited bandwidth with no angle measurements while providing robustness to wireless propagation phenomena such as diffraction. Our proposed method leverages offline data collection during RF-mapping, and finds the location of virtual anchors (VAs), i.e., mirror images of a physical anchor w.r.t reflectors, through an iterative process called successive tap removal (STR). Afterwards, machine learning (ML) models are trained to predict dominant multipath components of the received wireless channel at a given location. Found VAs and their associated ML models stand for intermediate entities that represent an RF-environment. As an application, we use the developed models in the context of multipath assisted positioning to improve positioning accuracy in challenging indoor environments with heavy non-line-of-sight (NLoS) conditions. Finally, we extend our ideas to systems with multi-antenna transmitters and show that VA detection accuracy can be improved, bringing higher accuracy to the downstream positioning applications.
更多查看译文
关键词
Environment learning,AI/machine learning (ML),5G advanced,sixth generation (6G),precise positioning
AI 理解论文
溯源树
样例
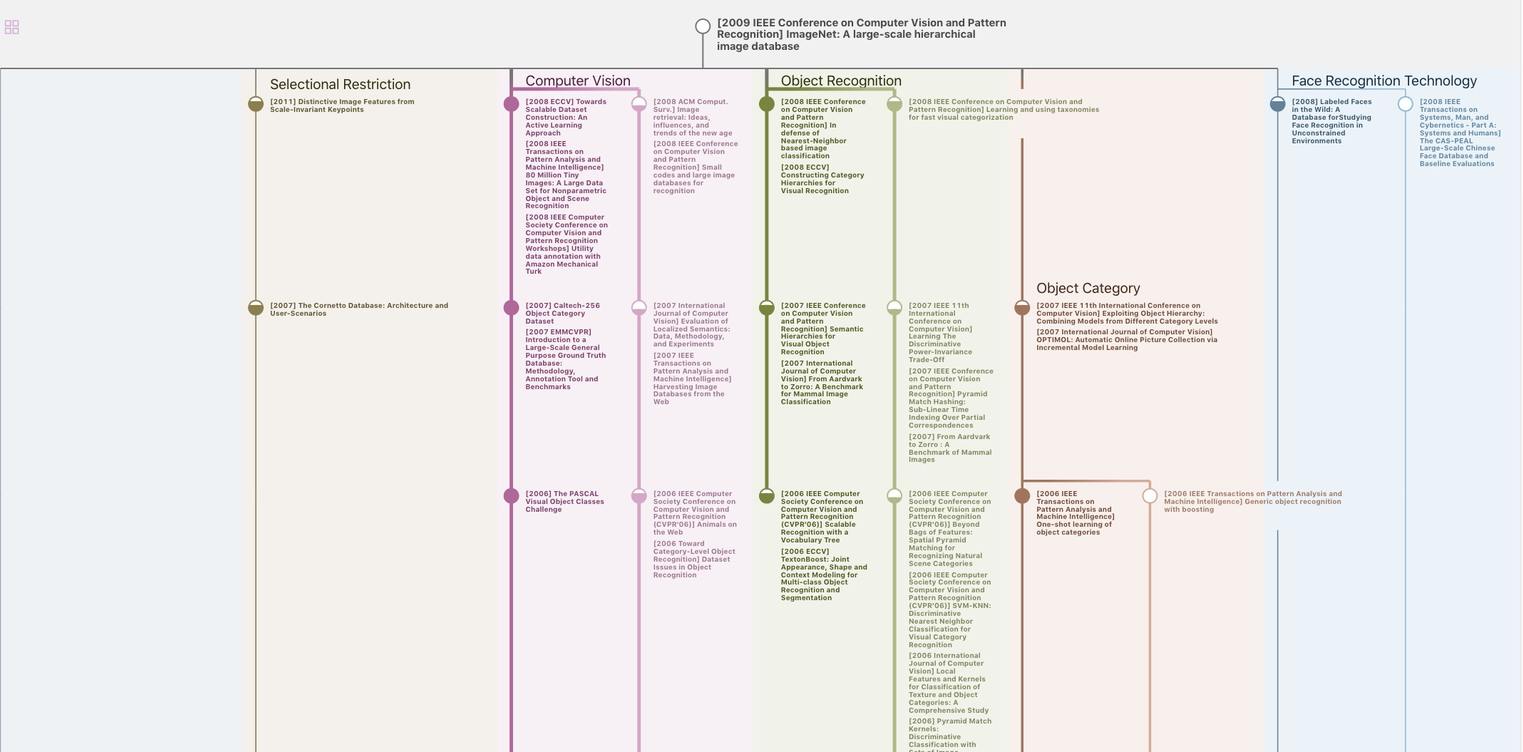
生成溯源树,研究论文发展脉络
Chat Paper
正在生成论文摘要