Few-Shot Rotation-Invariant Aerial Image Semantic Segmentation
IEEE TRANSACTIONS ON GEOSCIENCE AND REMOTE SENSING(2024)
摘要
Few-shot aerial image semantic segmentation is a challenging task that requires precisely parsing unseen-category objects in query aerial images with limited annotated support aerial images. Formally, category prototypes would be extracted from support samples to segment query images in a pixel-to-pixel matching manner. However, aerial objects in aerial images are often distributed with arbitrary orientations, and varying orientations could cause a dramatic feature change. This unique property of aerial images renders conventional matching manner without consideration of orientations fails to activate same-category objects with different orientations. Furthermore, the oscillation of the confidence scores in existing rotation-insensitive algorithms, engendered by the striking changes of object orientations, often leads to false recognition of lower scored rotated semantic objects. To tackle these challenges, inspired by the intrinsic rotation invariance in aerial images, we propose a novel few-shot rotation-invariant aerial semantic segmentation network (FRINet) to efficiently segment aerial semantic objects with diverse orientations. Specifically, through extracting orientation-varying yet category-consistent support information, FRINet provides rotation-adaptive matching for each query feature in a feature-aggregation manner. Meanwhile, to encourage consistent predictions for aerial objects with arbitrary orientations, segmentation predictions from different orientations are supervised by the same label and further fused to obtain the final rotation-invariant prediction in a complementary manner. Moreover, aiming at providing a better solution searching space, the backbones are newly pretrained in the base category to basically boost the segmentation performance. Extensive experiments on the few-shot aerial image semantic segmentation benchmark demonstrate that the proposed FRINet achieves a new state-of-the-art performance. The code is available at https://github.com/caoql98/FRINet.
更多查看译文
关键词
Consistent prediction,few-shot aerial semantic segmentation,rotation invariance,rotation-adaptive matching
AI 理解论文
溯源树
样例
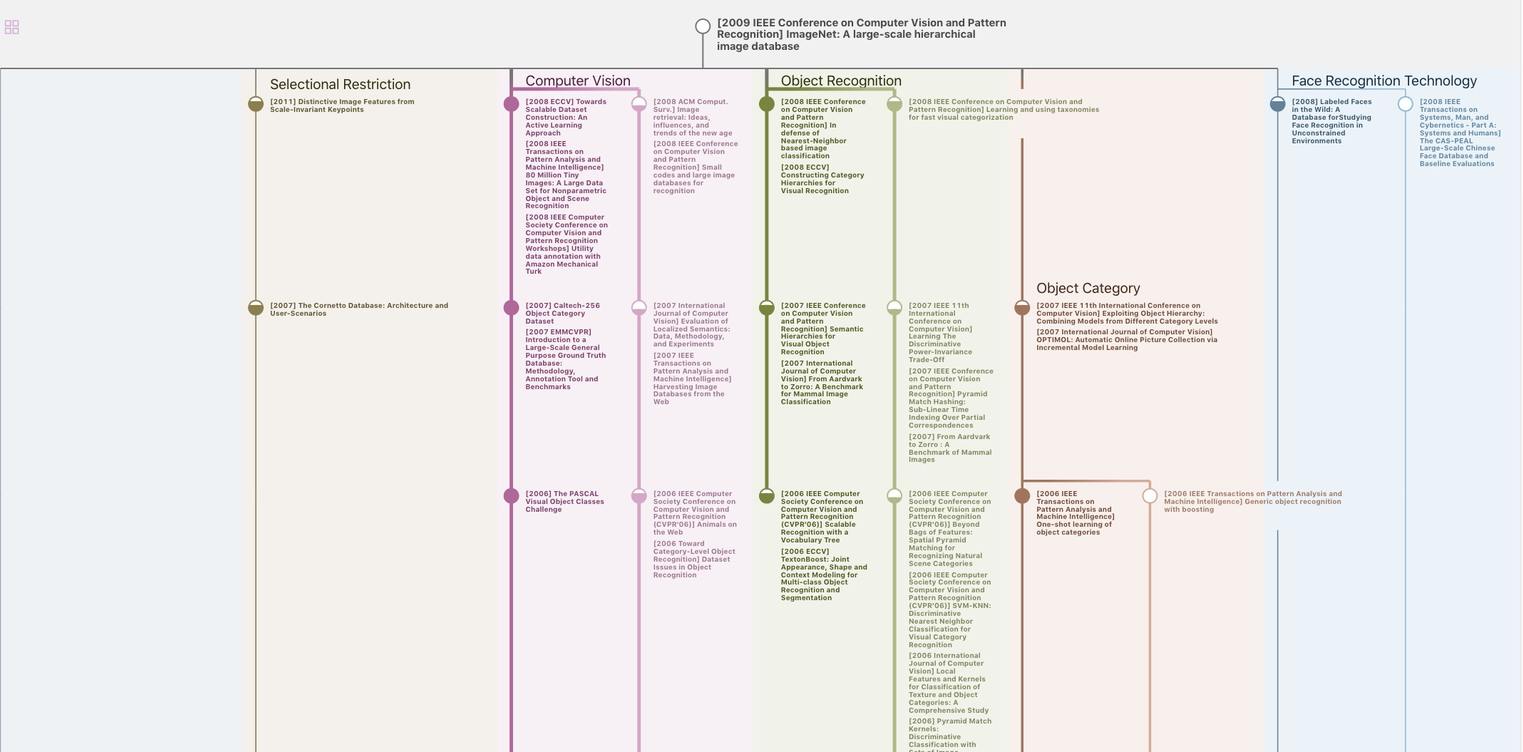
生成溯源树,研究论文发展脉络
Chat Paper
正在生成论文摘要