Towards Mitigating Spurious Correlations in the Wild: A Benchmark & a more Realistic Dataset
CoRR(2023)
摘要
Deep neural networks often exploit non-predictive features that are spuriously correlated with class labels, leading to poor performance on groups of examples without such features. Despite the growing body of recent works on remedying spurious correlations, the lack of a standardized benchmark hinders reproducible evaluation and comparison of the proposed solutions. To address this, we present SpuCo, a python package with modular implementations of state-of-the-art solutions enabling easy and reproducible evaluation of current methods. Using SpuCo, we demonstrate the limitations of existing datasets and evaluation schemes in validating the learning of predictive features over spurious ones. To overcome these limitations, we propose two new vision datasets: (1) SpuCoMNIST, a synthetic dataset that enables simulating the effect of real world data properties e.g. difficulty of learning spurious feature, as well as noise in the labels and features; (2) SpuCoAnimals, a large-scale dataset curated from ImageNet that captures spurious correlations in the wild much more closely than existing datasets. These contributions highlight the shortcomings of current methods and provide a direction for future research in tackling spurious correlations. SpuCo, containing the benchmark and datasets, can be found at https://github.com/BigML-CS-UCLA/SpuCo, with detailed documentation available at https://spuco.readthedocs.io/en/latest/.
更多查看译文
关键词
spurious correlations,benchmark,dataset,wild
AI 理解论文
溯源树
样例
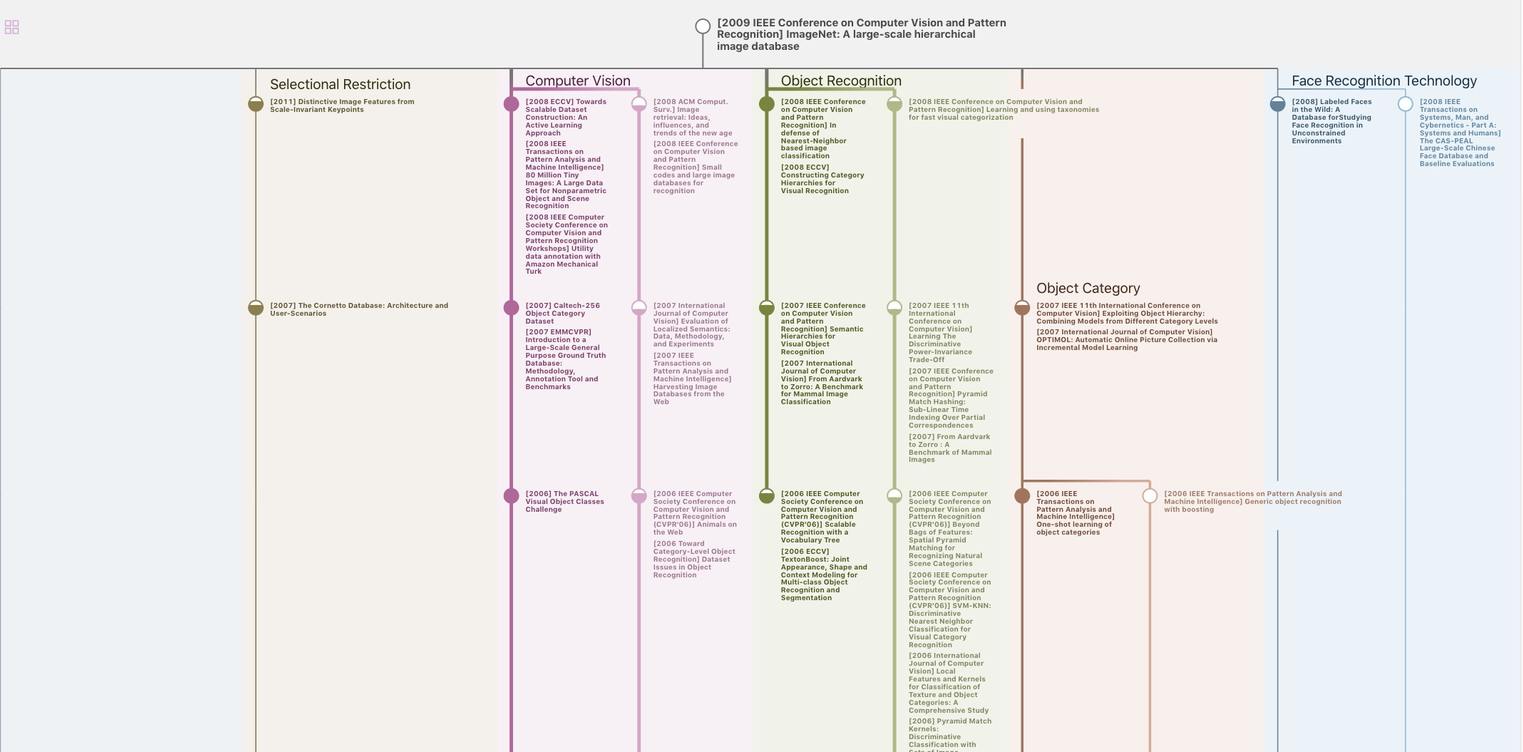
生成溯源树,研究论文发展脉络
Chat Paper
正在生成论文摘要