Sea Clutter Feature Prediction and Parameters Inversion Using Deep Learning Model
IEEE Transactions on Industrial Informatics(2023)
摘要
The characteristics of sea clutter in real marine environments in different sea areas play a vital role in military industry, such as radar detection, remote sensing, SAR imaging, and situational awareness. In this article, a deep neural network (DNN) sea clutter model is proposed based on the sea clutter big data under the real marine environment to study the characteristics of sea clutter and parameter inversion. Based on the ERA-Interim reanalysis (2015-2017), a database of marine environmental elements in China's offshore waters was established, and a spatiotemporal prediction model for marine environmental elements was proposed to improve missing values. Considering the scattering mechanism of sea surface at different scales comprehensively, a large database of sea clutter time series of multiscale real surface is established and comparison with the experimental data. Aiming at the coastal waters of China, the long short-term memory model and DNN are used to establish the correlation model between marine environmental elements and sea clutter characteristics, and the prediction and parameter inversion of sea clutter characteristics based on sea clutter big data are studied. The results show that the coefficients of determination of the predicted fitted curves for the HH and VV polarization amplitudes reach 0.9249 and 0.8872, respectively. The inversion of wave heights in different sea areas is the lowest in the South China Sea (accuracy rate of 78%) and the highest in the East China Sea (accuracy rate is 90%). The results of this article can help improve the ability of sea surface remote sensing and sea clutter suppression.
更多查看译文
关键词
Big data,deep neural network (DNN),long short-term memory (LSTM),parameter inversion,sea clutter prediction
AI 理解论文
溯源树
样例
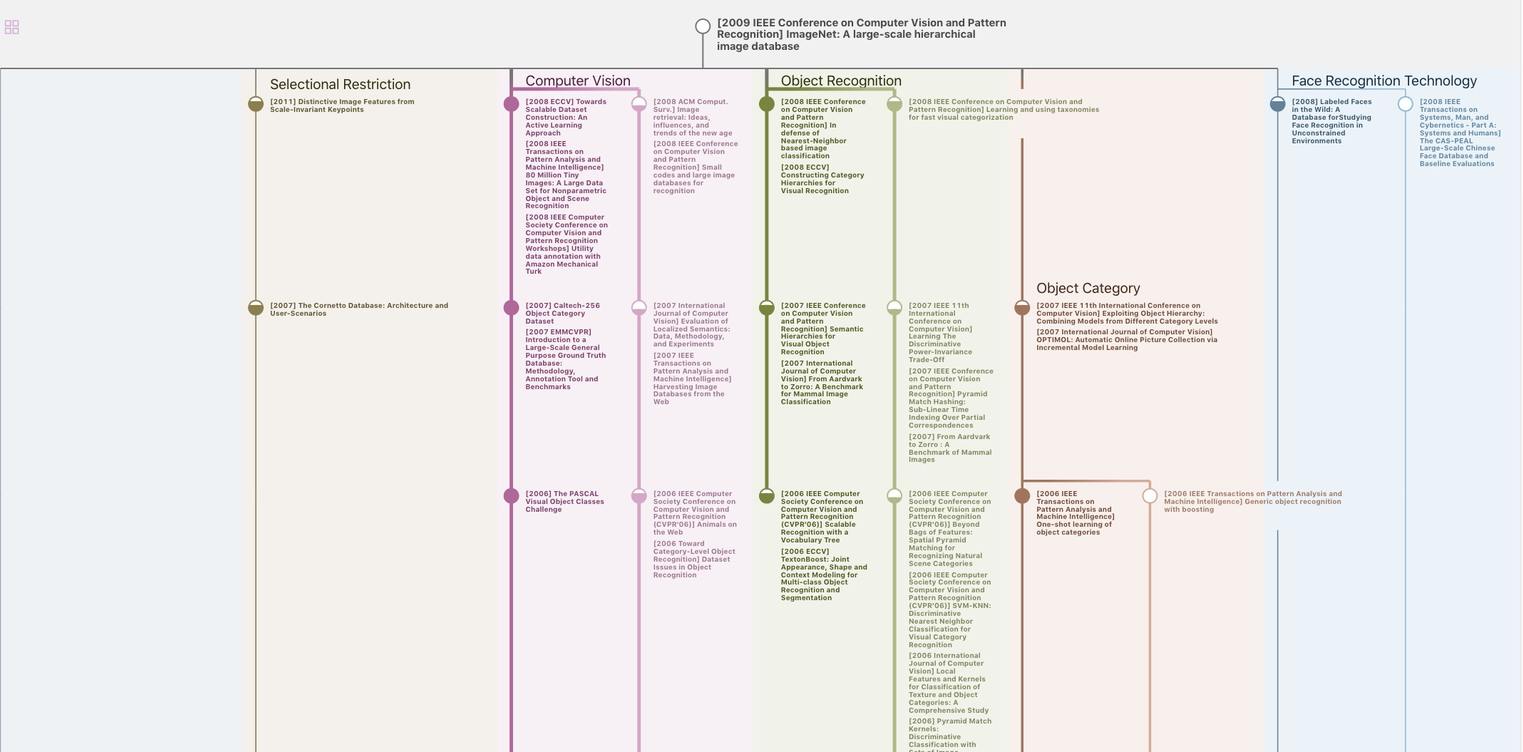
生成溯源树,研究论文发展脉络
Chat Paper
正在生成论文摘要