Hierarchical Neural Simulation-Based Inference Over Event Ensembles
CoRR(2023)
摘要
When analyzing real-world data it is common to work with event ensembles, which comprise sets of observations that collectively constrain the parameters of an underlying model of interest. Such models often have a hierarchical structure, where "local" parameters impact individual events and "global" parameters influence the entire dataset. We introduce practical approaches for optimal dataset-wide probabilistic inference in cases where the likelihood is intractable, but simulations can be realized via forward modeling. We construct neural estimators for the likelihood(-ratio) or posterior and show that explicitly accounting for the model's hierarchical structure can lead to tighter parameter constraints. We ground our discussion using case studies from the physical sciences, focusing on examples from particle physics (particle collider data) and astrophysics (strong gravitational lensing observations).
更多查看译文
关键词
event,simulation-based
AI 理解论文
溯源树
样例
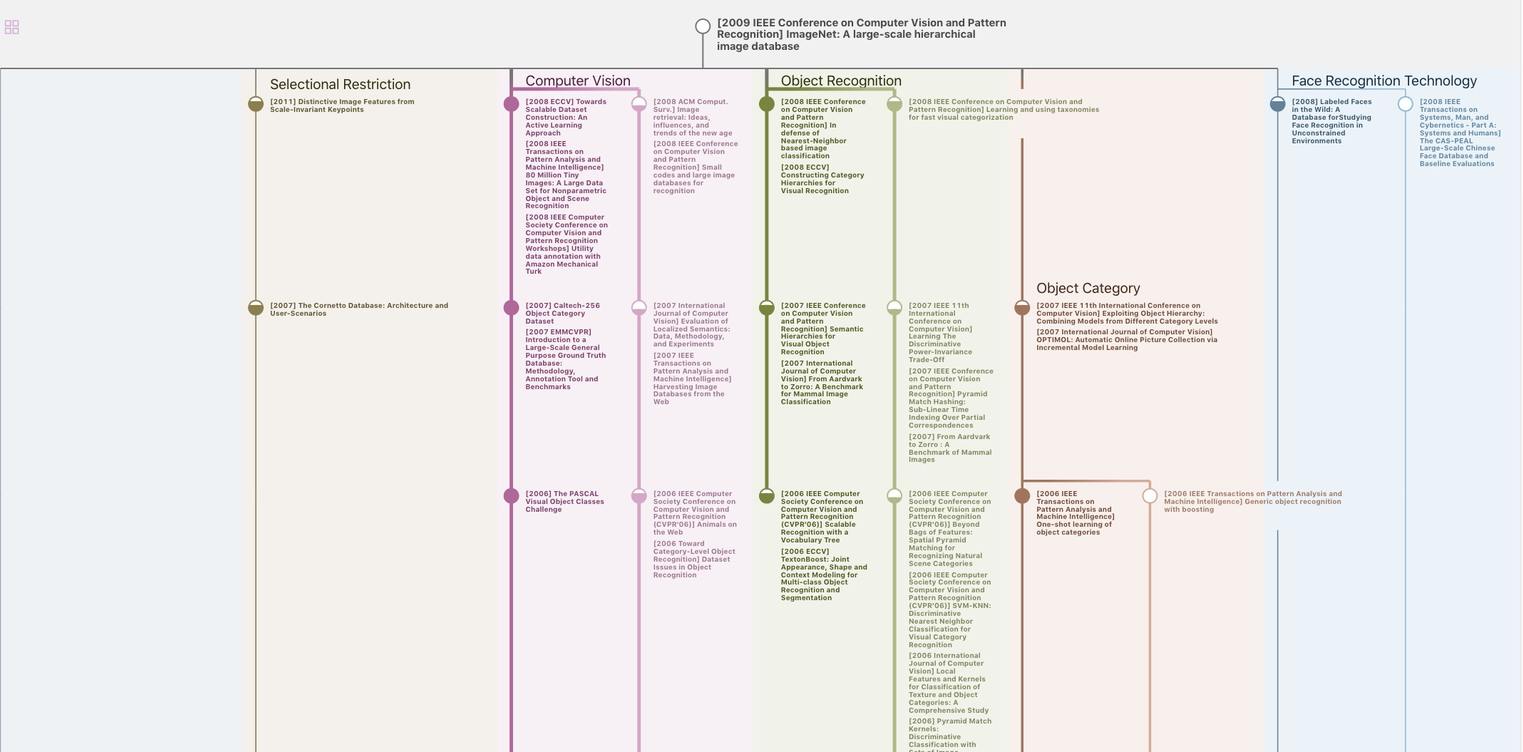
生成溯源树,研究论文发展脉络
Chat Paper
正在生成论文摘要