CEMSSL: A Unified Framework for Multi-Solution Inverse Kinematic Model Learning of Robot Arms with High-Precision Manipulation
CoRR(2023)
摘要
Multiple solutions mainly originate from the existence of redundant degrees of freedom in the robot arm, which may cause difficulties in inverse model learning but they can also bring many benefits, such as higher flexibility and robustness. Current multi-solution inverse model learning methods rely on conditional deep generative models, yet they often fail to achieve sufficient precision when learning multiple solutions. In this paper, we propose Conditional Embodied Self-Supervised Learning (CEMSSL) for robot arm multi-solution inverse model learning, and present a unified framework for high-precision multi-solution inverse model learning that is applicable to other conditional deep generative models. Our experimental results demonstrate that our framework can achieve a significant improvement in precision (up to 2 orders of magnitude) while preserving the properties of the original method. The related code will be available soon.
更多查看译文
关键词
robot arms,learning,multi-solution,high-precision
AI 理解论文
溯源树
样例
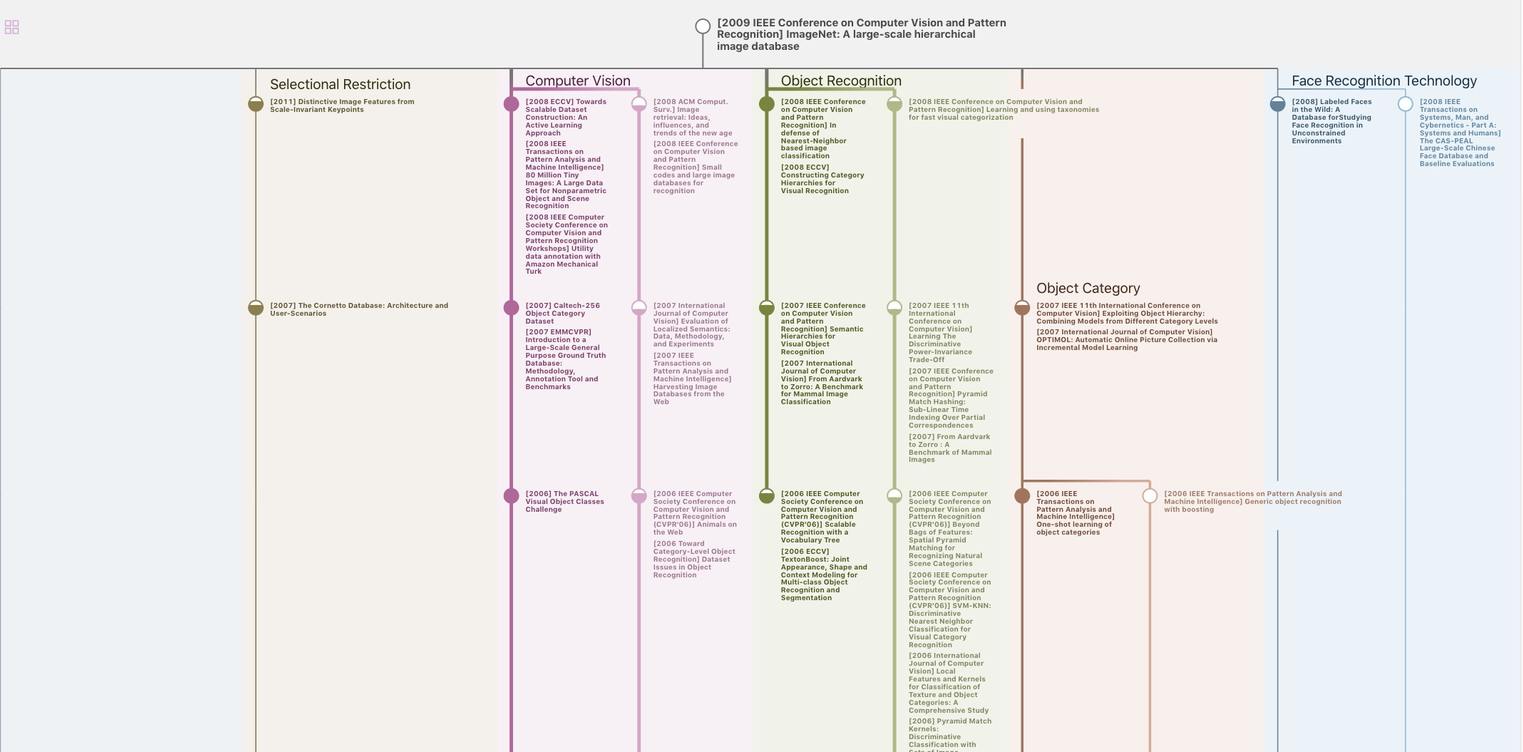
生成溯源树,研究论文发展脉络
Chat Paper
正在生成论文摘要