Solving the time-complexity problem and tuning the performance of quantum reservoir computing by artificial memory restriction
arxiv(2023)
摘要
Quantum reservoir computing is a computing approach which aims at utilising
the complexity and high-dimensionality of small quantum systems, together with
the fast trainability of reservoir computing, in order to solve complex tasks.
The suitability of quantum reservoir computing for solving temporal tasks is
hindered by the collapse of the quantum system when measurements are made. This
leads to the erasure of the memory of the reservoir. Hence, for every output,
the entire input signal is needed to reinitialise the reservoir, leading to
quadratic time complexity. Overcoming this issue is critical to the hardware
implementation of quantum reservoir computing. We propose artificially
restricting the memory of the quantum reservoir by only using a small number
inputs to reinitialise the reservoir after measurements are performed, leading
to linear time complexity. This not only substantially reduces the number of
quantum operations needed to perform timeseries prediction tasks, it also
provides a means of tuning the nonlinearity of the response of the reservoir,
which can lead to significant performance improvement. We numerically study the
linear and quadratic algorithms for a fully connected transverse Ising model
and a quantum processor model. We find that our proposed linear algorithm not
only significantly reduces the computational cost but also provides an
experimental accessible means to optimise the task specific reservoir computing
performance.
更多查看译文
AI 理解论文
溯源树
样例
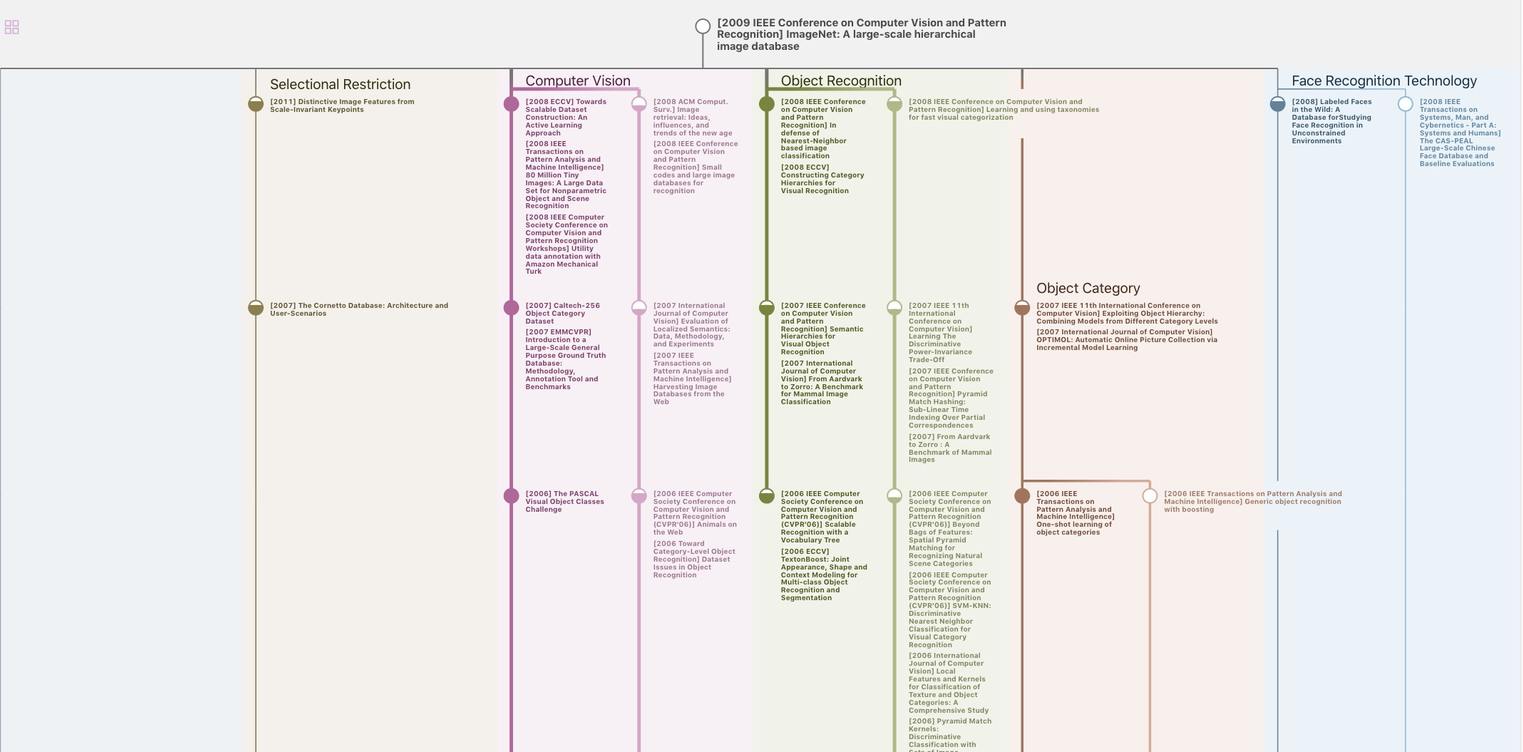
生成溯源树,研究论文发展脉络
Chat Paper
正在生成论文摘要