Device–Edge Collaborative Differentiated Data Caching Strategy Toward AIoT
IEEE Internet of Things Journal(2023)
摘要
Caching AI of Things (AIoT) data at the edge can reduce the load on cloud centers while providing real-time services for AIoT users. Existing static caching strategies based on popularity prediction fail to meet users’ demands for time-varying entity data, while dynamic caching strategies focus only on evaluating the time-varying state characteristics of entity data, but ignore the differences in popularity among entities, resulting in poor service experience. To this end, a device–edge collaborative differentiated data caching strategy considering static entity popularity as well as dynamic system state is proposed. First, a hot entity recognition method centered on user interests is designed to achieve individual preference estimation by mining users’ long short-term interests, and then achieve group interest prediction based on social computing. Based on this, a dynamic caching optimization method is designed, which considers the timeliness of entity data and communication cost of the system to design the objective function of optimal cache decision and then solve it based on reinforcement learning. Simulation results demonstrate that the proposed caching strategy achieves better performance than other benchmark strategies in terms of cache hit rate and search cost.
更多查看译文
关键词
device–edge,collaborative,device–edge
AI 理解论文
溯源树
样例
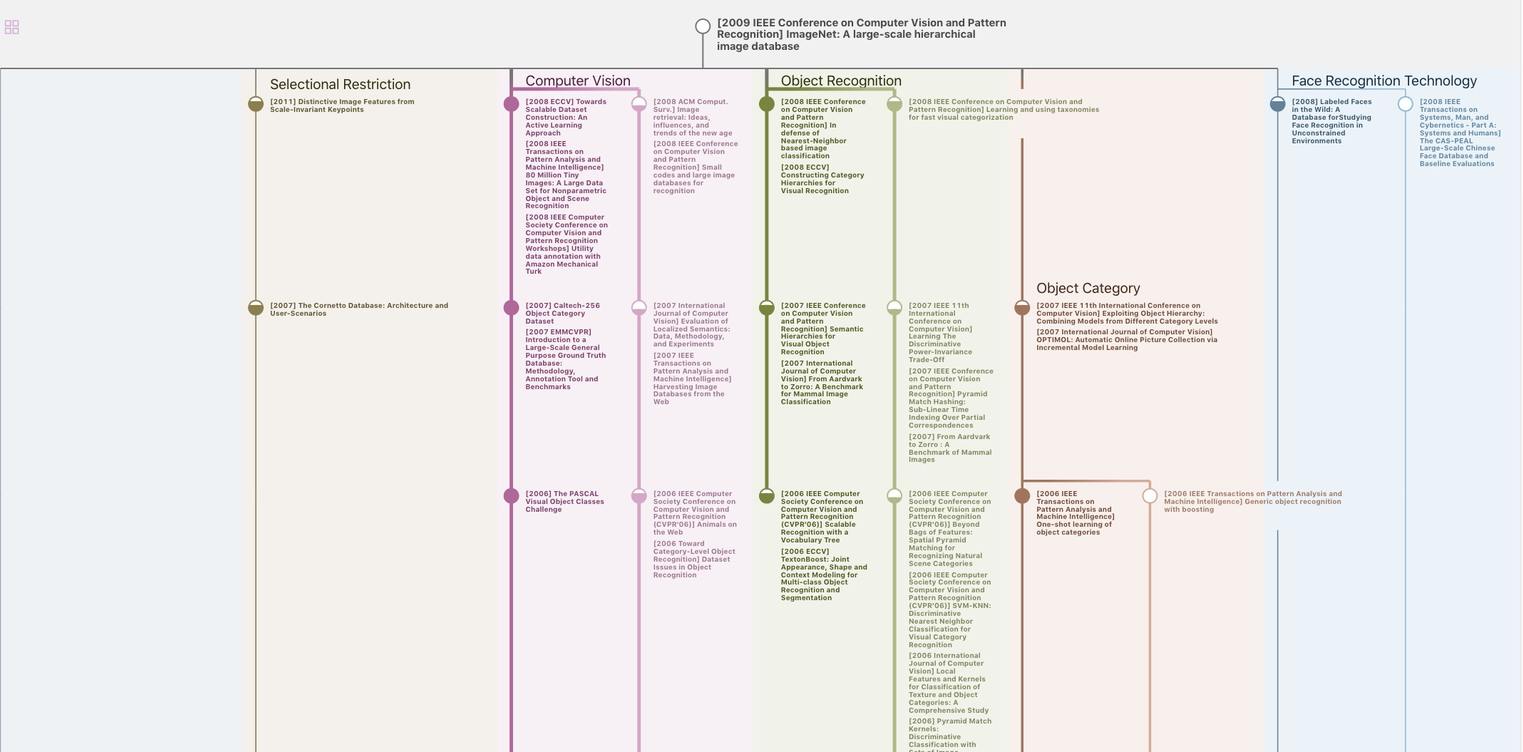
生成溯源树,研究论文发展脉络
Chat Paper
正在生成论文摘要