DiversiGATE: A Comprehensive Framework for Reliable Large Language Models
CoRR(2023)
摘要
In this paper, we introduce DiversiGATE, a unified framework that consolidates diverse methodologies for LLM verification. The proposed framework comprises two main components: Diversification and Aggregation which provide a holistic perspective on existing verification approaches, such as Self-Consistency, Math Prompter and WebGPT. Furthermore, we propose a novel `SelfLearner' model that conforms to the DiversiGATE framework which can learn from its own outputs and refine its performance over time, leading to improved accuracy. To evaluate the effectiveness of SelfLearner, we conducted a rigorous series of experiments, including tests on synthetic data as well as on popular arithmetic reasoning benchmarks such as GSM8K. Our results demonstrate that our approach outperforms traditional LLMs, achieving a considerable 54.8% -> 61.8% improvement on the GSM8K benchmark.
更多查看译文
关键词
reliable large language
AI 理解论文
溯源树
样例
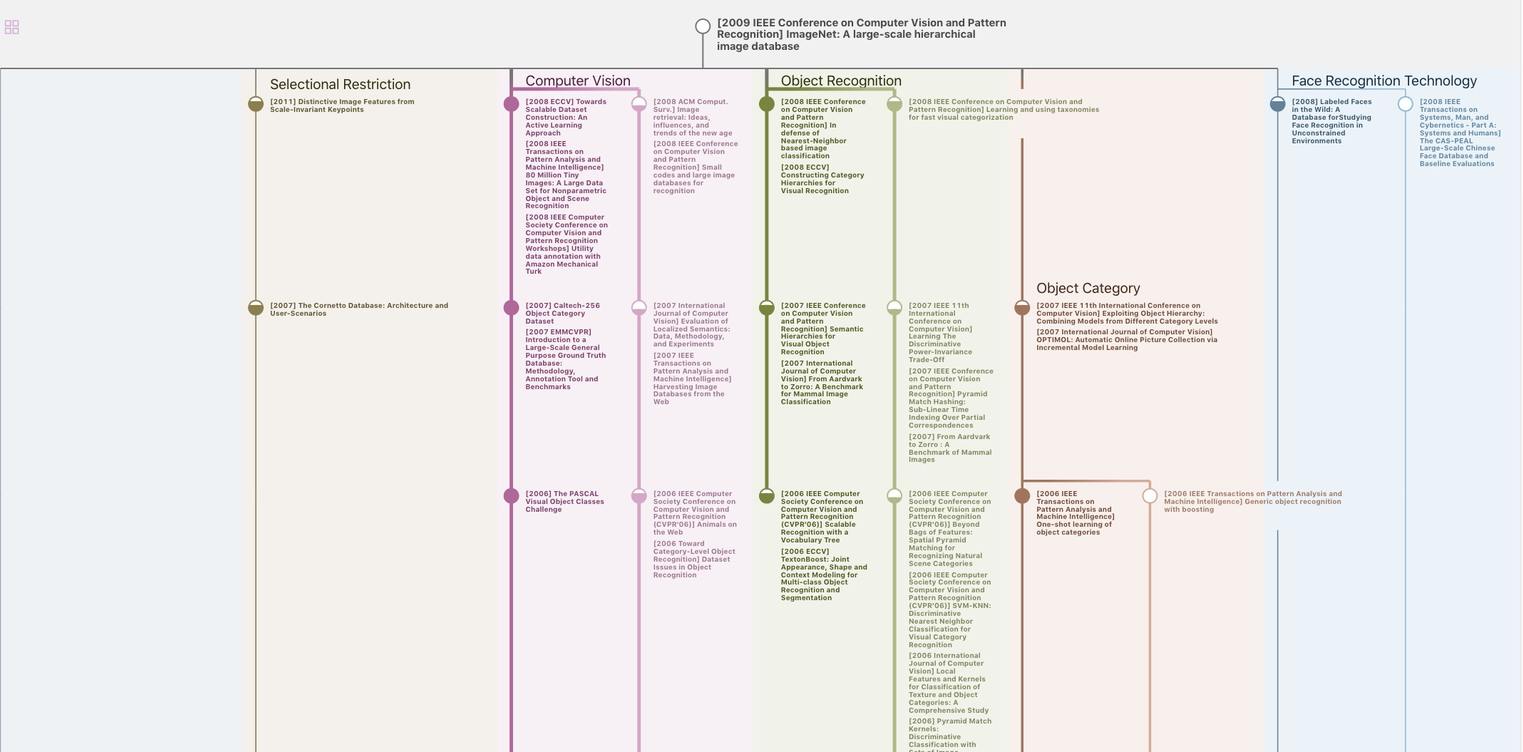
生成溯源树,研究论文发展脉络
Chat Paper
正在生成论文摘要