MUSER: A MUlti-Step Evidence Retrieval Enhancement Framework for Fake News Detection
PROCEEDINGS OF THE 29TH ACM SIGKDD CONFERENCE ON KNOWLEDGE DISCOVERY AND DATA MINING, KDD 2023(2023)
摘要
The ease of spreading false information online enables individuals with malicious intent to manipulate public opinion and destabilize social stability. Recently, fake news detection based on evidence retrieval has gained popularity in an effort to identify fake news reliably and reduce its impact. Evidence retrieval-based methods can improve the reliability of fake news detection by computing the textual consistency between the evidence and the claim in the news. In this paper, we propose a framework for fake news detection based on MUlti-Step Evidence Retrieval enhancement (MUSER), which simulates the steps of human beings in the process of reading news, summarizing, consulting materials, and inferring whether the news is true or fake. Our model can explicitly model dependencies among multiple pieces of evidence, and perform multi-step associations for the evidence required for news verification through multi-step retrieval. In addition, our model is able to automatically collect existing evidence through paragraph retrieval and key evidence selection, which can save the tedious process of manual evidence collection. We conducted extensive experiments on real-world datasets in different languages, and the results demonstrate that our proposed model outperforms state-of-the-art baseline methods for detecting fake news by at least 3% in F1-Macro and 4% in F1-Micro. Furthermore, it provides interpretable evidence for end users.
更多查看译文
关键词
Evidence-based Fake News Detection,Multi-step Retrieval,Explainability
AI 理解论文
溯源树
样例
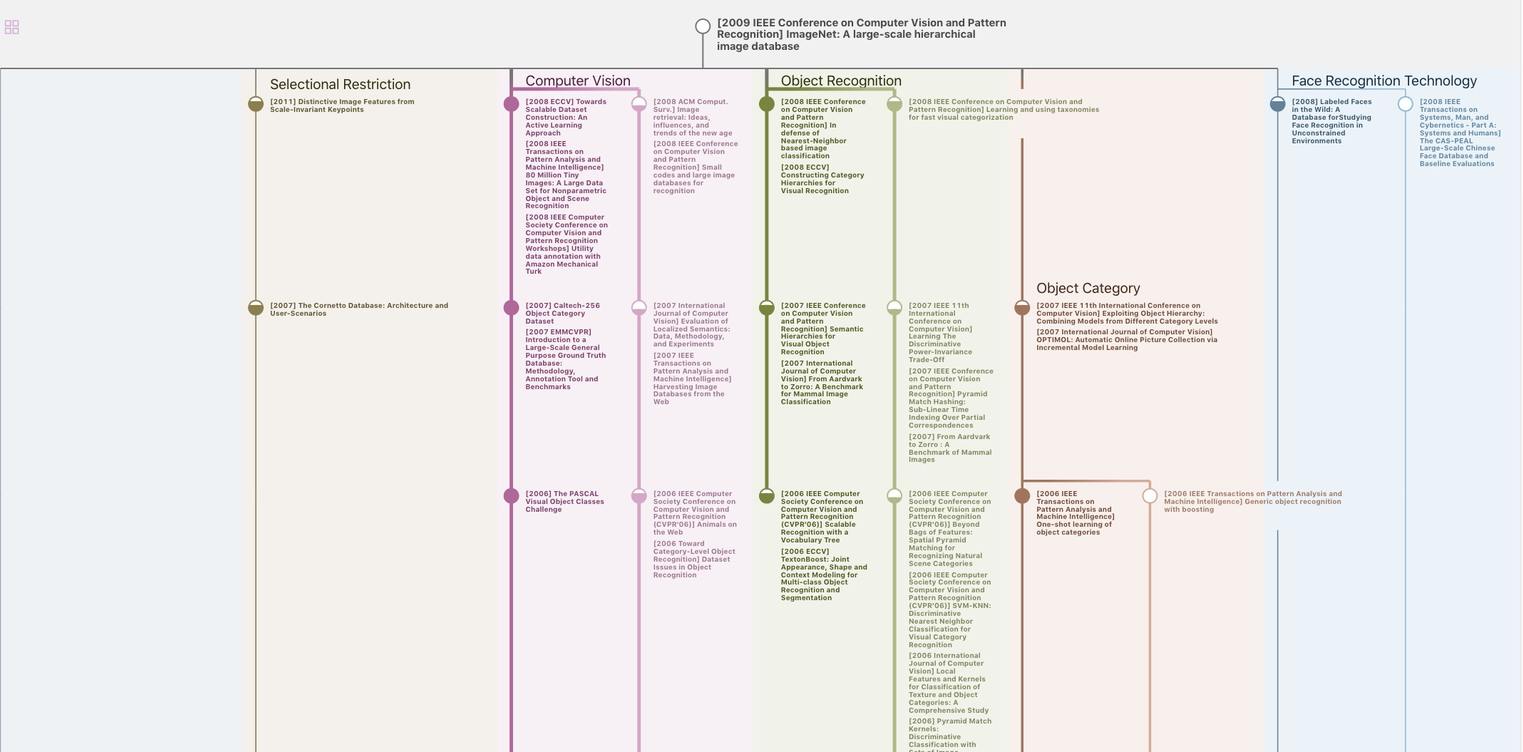
生成溯源树,研究论文发展脉络
Chat Paper
正在生成论文摘要