Understanding quantum machine learning also requires rethinking generalization
Nature Communications(2024)
摘要
Quantum machine learning models have shown successful generalization performance even when trained with few data. In this work, through systematic randomization experiments, we show that traditional approaches to understanding generalization fail to explain the behavior of such quantum models. Our experiments reveal that state-of-the-art quantum neural networks accurately fit random states and random labeling of training data. This ability to memorize random data defies current notions of small generalization error, problematizing approaches that build on complexity measures such as the VC dimension, the Rademacher complexity, and all their uniform relatives. We complement our empirical results with a theoretical construction showing that quantum neural networks can fit arbitrary labels to quantum states, hinting at their memorization ability. Our results do not preclude the possibility of good generalization with few training data but rather rule out any possible guarantees based only on the properties of the model family. These findings expose a fundamental challenge in the conventional understanding of generalization in quantum machine learning and highlight the need for a paradigm shift in the study of quantum models for machine learning tasks.
更多查看译文
关键词
quantum machine learning,generalization,machine learning
AI 理解论文
溯源树
样例
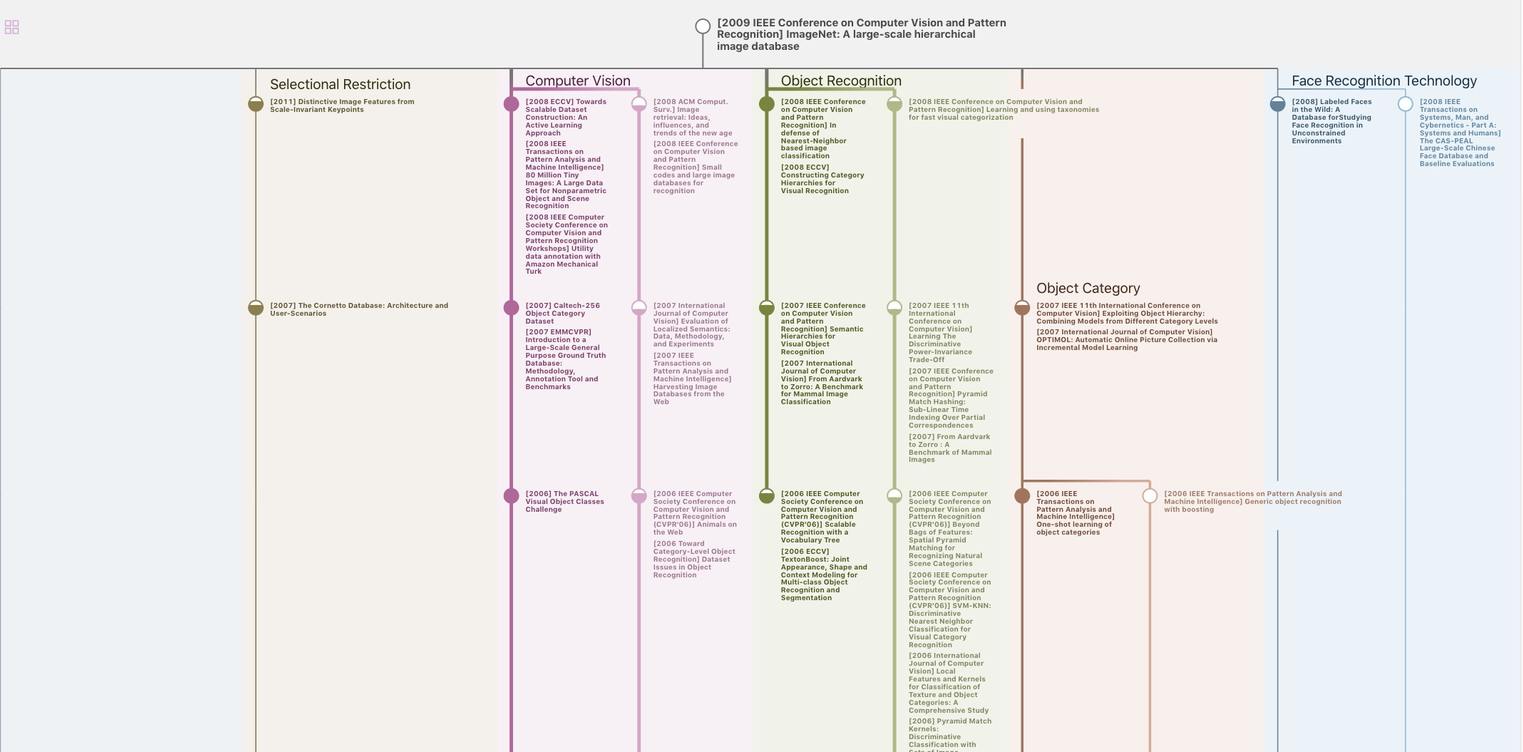
生成溯源树,研究论文发展脉络
Chat Paper
正在生成论文摘要