Masked Conditional Variational Autoencoders for Chromosome Straightening
IEEE TRANSACTIONS ON MEDICAL IMAGING(2024)
摘要
Karyotyping is of importance for detecting chromosomal aberrations in human disease. However, chromosomes easily appear curved in microscopic images, which prevents cytogeneticists from analyzing chromosome types. To address this issue, we propose a framework for chromosome straightening, which comprises a preliminary processing algorithm and a generative model called masked conditional variational autoencoders (MC-VAE). The processing method utilizes patch rearrangement to address the difficulty in erasing low degrees of curvature, providing reasonable preliminary results for the MC-VAE. The MC-VAE further straightens the results by leveraging chromosome patches conditioned on their curvatures to learn the mapping between banding patterns and conditions. During model training, we apply a masking strategy with a high masking ratio to train the MC-VAE with eliminated redundancy. This yields a non-trivial reconstruction task, allowing the model to effectively preserve chromosome banding patterns and structure details in the reconstructed results. Extensive experiments on three public datasets with two stain styles show that our framework surpasses the performance of state-of-the-art methods in retaining banding patterns and structure details. Compared to using real-world bent chromosomes, the use of high-quality straightened chromosomes generated by our proposed method can improve the performance of various deep learning models for chromosome classification by a large margin. Such a straightening approach has the potential to be combined with other karyotyping systems to assist cytogeneticists in chromosome analysis.
更多查看译文
关键词
Biological cells,Deep learning,Task analysis,Image reconstruction,Feature extraction,Training,Microscopy,Karyotyping,chromosome straightening,variational autoencoders,deep learning,microscopy image analysis
AI 理解论文
溯源树
样例
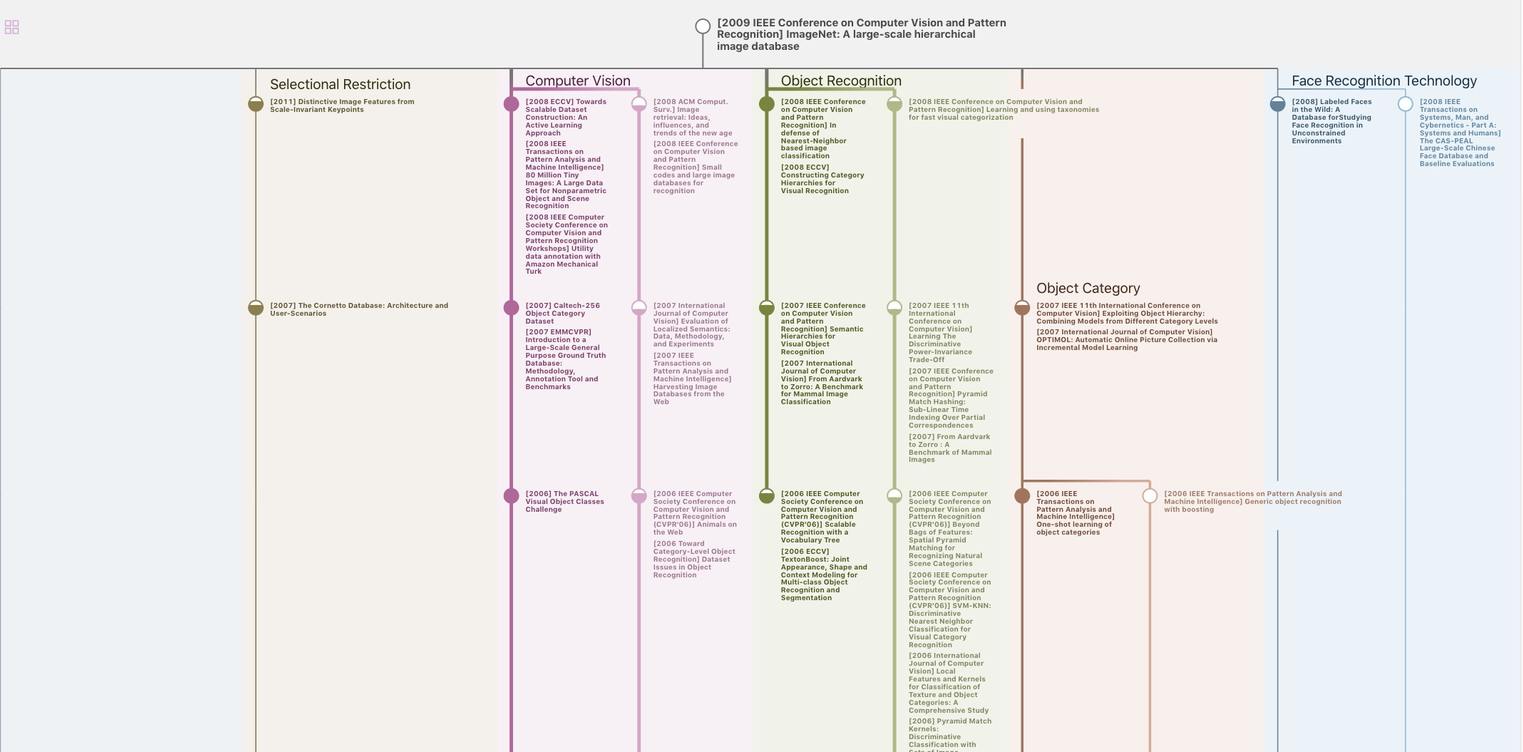
生成溯源树,研究论文发展脉络
Chat Paper
正在生成论文摘要