TCE: A Test-Based Approach to Measuring Calibration Error
CoRR(2023)
摘要
This paper proposes a new metric to measure the calibration error of probabilistic binary classifiers, called test-based calibration error (TCE). TCE incorporates a novel loss function based on a statistical test to examine the extent to which model predictions differ from probabilities estimated from data. It offers (i) a clear interpretation, (ii) a consistent scale that is unaffected by class imbalance, and (iii) an enhanced visual representation with repect to the standard reliability diagram. In addition, we introduce an optimality criterion for the binning procedure of calibration error metrics based on a minimal estimation error of the empirical probabilities. We provide a novel computational algorithm for optimal bins under bin-size constraints. We demonstrate properties of TCE through a range of experiments, including multiple real-world imbalanced datasets and ImageNet 1000.
更多查看译文
关键词
calibration,test-based
AI 理解论文
溯源树
样例
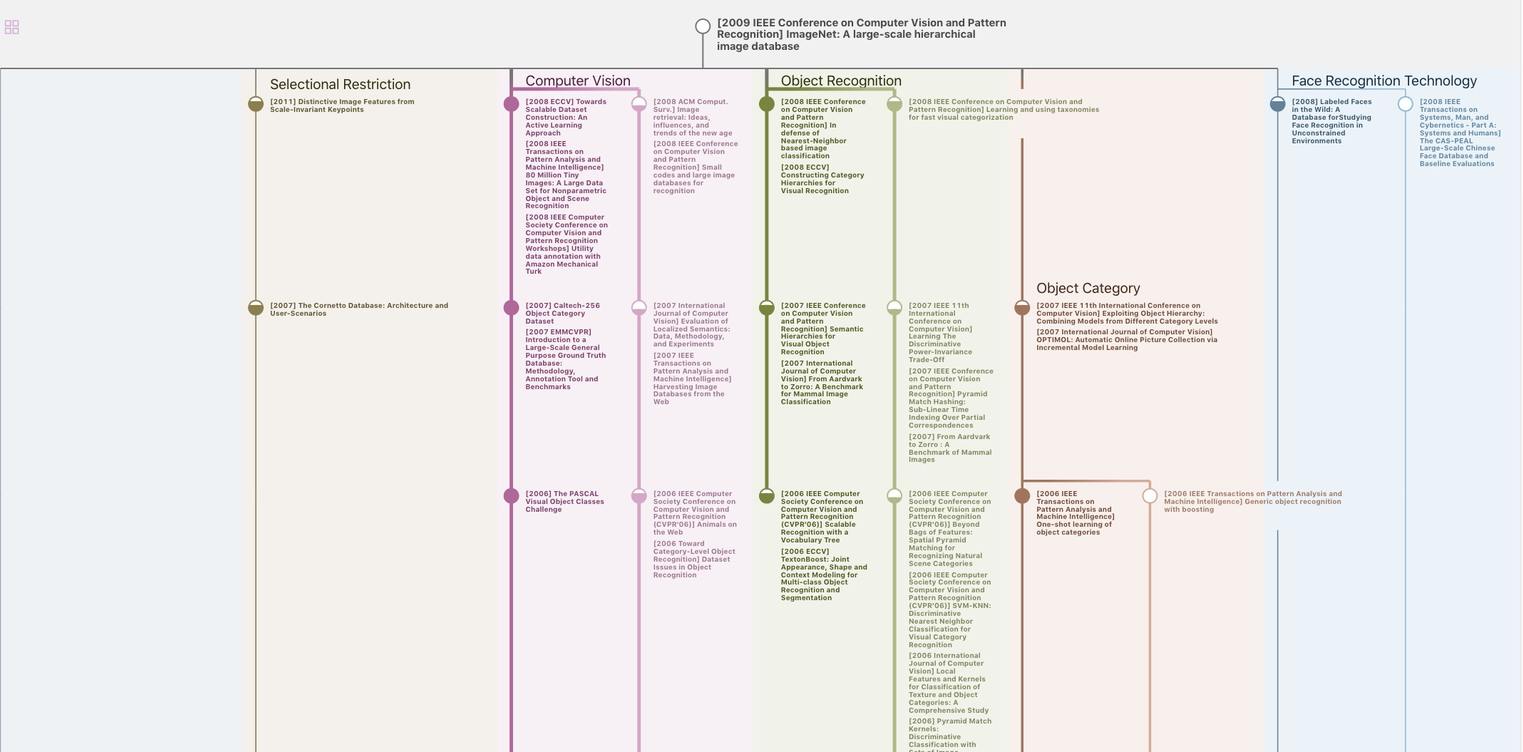
生成溯源树,研究论文发展脉络
Chat Paper
正在生成论文摘要